diff --git a/.github/dependabot.yml b/.github/dependabot.yml
index 0df37b1230..6267930e9e 100644
--- a/.github/dependabot.yml
+++ b/.github/dependabot.yml
@@ -21,3 +21,6 @@ updates:
python:
patterns:
- "*"
+ ignore:
+ # TODO: ignore all updates for JAX GPU due to cuda version issue
+ - dependency-name: "jax[cuda12_pip]"
diff --git a/.github/workflows/actions.yml b/.github/workflows/actions.yml
index 8be69b967b..316e623c57 100644
--- a/.github/workflows/actions.yml
+++ b/.github/workflows/actions.yml
@@ -38,6 +38,8 @@ jobs:
pip install torch>=2.0.1+cpu
pip install "jax[cpu]"
pip install keras-core
+ pip install keras-nlp-nightly --no-deps
+ pip install tensorflow-text==2.15
pip install -e ".[tests]" --progress-bar off --upgrade
- name: Test with pytest
env:
@@ -75,6 +77,7 @@ jobs:
run: |
pip install -r requirements.txt
pip install -e ".[tests]" --progress-bar off --upgrade
+ pip install keras-nlp-nightly
- name: Test with pytest
env:
TEST_CUSTOM_OPS: false # TODO(ianstenbit): test custom ops, or figure out what our story is here
diff --git a/.github/workflows/scorecard.yml b/.github/workflows/scorecard.yml
index 98509aef93..8bcdbe833a 100644
--- a/.github/workflows/scorecard.yml
+++ b/.github/workflows/scorecard.yml
@@ -45,7 +45,7 @@ jobs:
# Upload the results as artifacts (optional). Commenting out will disable uploads of run results in SARIF
# format to the repository Actions tab.
- name: "Upload artifact"
- uses: actions/upload-artifact@26f96dfa697d77e81fd5907df203aa23a56210a8 # v4.3.0
+ uses: actions/upload-artifact@5d5d22a31266ced268874388b861e4b58bb5c2f3 # v4.3.1
with:
name: SARIF file
path: results.sarif
@@ -53,6 +53,6 @@ jobs:
# Upload the results to GitHub's code scanning dashboard.
- name: "Upload to code-scanning"
- uses: github/codeql-action/upload-sarif@b7bf0a3ed3ecfa44160715d7c442788f65f0f923 # v3.23.2
+ uses: github/codeql-action/upload-sarif@e675ced7a7522a761fc9c8eb26682c8b27c42b2b # v3.24.1
with:
sarif_file: results.sarif
diff --git a/.kokoro/github/ubuntu/gpu/build.sh b/.kokoro/github/ubuntu/gpu/build.sh
index 9d07218317..76ac0631b4 100644
--- a/.kokoro/github/ubuntu/gpu/build.sh
+++ b/.kokoro/github/ubuntu/gpu/build.sh
@@ -20,6 +20,8 @@ nvcc --version
cd "src/github/keras-cv"
pip install -U pip setuptools
+# psutil is used by background log reader
+pip install -U psutil
if [ "${KERAS2:-0}" == "1" ]
then
@@ -29,21 +31,26 @@ then
pip install --extra-index-url https://download.pytorch.org/whl/cpu torch==2.1.0+cpu
pip install torchvision~=0.16.0
pip install "jax[cpu]"
+ pip install keras-nlp-nightly --no-deps
+ pip install tensorflow-text==2.15
elif [ "$KERAS_BACKEND" == "tensorflow" ]
then
echo "TensorFlow backend detected."
pip install -r requirements-tensorflow-cuda.txt --progress-bar off
+ pip install keras-nlp-nightly
elif [ "$KERAS_BACKEND" == "jax" ]
then
echo "JAX backend detected."
pip install -r requirements-jax-cuda.txt --progress-bar off
+ pip install keras-nlp-nightly
elif [ "$KERAS_BACKEND" == "torch" ]
then
echo "PyTorch backend detected."
pip install -r requirements-torch-cuda.txt --progress-bar off
+ pip install keras-nlp-nightly
fi
pip install --no-deps -e "." --progress-bar off
diff --git a/LICENSE b/LICENSE
index f2e54070a8..c7a6ac1f74 100644
--- a/LICENSE
+++ b/LICENSE
@@ -1,3 +1,5 @@
+Files: keras_cv/*
+
Copyright © 2023 The KerasCV Authors
All code in this repository excluding the code located in
keras_cv/layers/preprocessing_3d/waymo is licensed under the Apache License,
@@ -206,7 +208,58 @@ folder is licensed under terms appearing below.
See the License for the specific language governing permissions and
limitations under the License.
-# The following applies only to the code appearing in
-# keras_cv/layers/preprocessing_3d/waymo
-
-License: https://github.com/keras-team/keras-cv/blob/master/keras_cv/layers/preprocessing_3d/waymo/LICENSE
+---
+
+Files: keras_cv/layers/preprocessing_3d/waymo/*
+
+Copyright (c) 2023 Waymo LLC. All rights reserved.
+
+Redistribution and use in source and binary forms, with or without
+modification, are permitted provided that the following conditions are
+met:
+
+1. Redistributions of source code must retain the above copyright
+notice, this list of conditions and the following disclaimer.
+2. Redistributions in binary form must reproduce the above
+copyright notice, this list of conditions and the following disclaimer
+in the documentation and/or other materials provided with the
+distribution.
+3. Neither the name of the copyright holder nor the names of its
+contributors may be used to endorse or promote products derived
+from this software without specific prior written permission.
+
+Additional IP Rights Grant (Patents)
+"Works" means the code located at keras_cv/layers/preprocessing_3d/waymo
+licensed from Waymo LLC ("Waymo") for inclusion in the KerasCV project at
+github.com/keras-team/keras-cv. “Patents" means the pending U.S. Patent App.
+No. 63/418,259 and any issued patents arising therefrom. Subject to the terms
+and conditions of this license, Waymo hereby grants to you a limited worldwide,
+non-exclusive, royalty-free, personal patent license to make, have made, use,
+and import the Works, where such license applies only to those Patent claims
+that are necessarily infringed by the Works executing the ”preprocessing_3d”
+augmentation library on 3D perception tasks using the
+“lidaraugment_keraspolicy.py” file. This grant does not include claims that
+would be infringed by combining the Works with other works, utilizing the Works
+on other tasks, or as a consequence of further modification of the Works. If
+you or your agent or exclusive licensee institute or order or agree to the
+institution of patent litigation or any other patent enforcement activity
+against any entity (including a cross-claim or counterclaim in a lawsuit)
+alleging that the Works or any activity using the Works to execute functions for
+3D perception tasks constitutes direct or contributory patent infringement, or
+inducement of patent infringement, then any patent rights granted to you under
+this license for the Works shall terminate as of the date such litigation is
+filed.
+
+DISCLAIMER
+
+THIS SOFTWARE IS PROVIDED BY THE COPYRIGHT HOLDERS AND CONTRIBUTORS
+"AS IS" AND ANY EXPRESS OR IMPLIED WARRANTIES, INCLUDING, BUT NOT
+LIMITED TO, THE IMPLIED WARRANTIES OF MERCHANTABILITY AND FITNESS FOR
+A PARTICULAR PURPOSE ARE DISCLAIMED. IN NO EVENT SHALL THE COPYRIGHT
+OWNER OR CONTRIBUTORS BE LIABLE FOR ANY DIRECT, INDIRECT, INCIDENTAL,
+SPECIAL, EXEMPLARY, OR CONSEQUENTIAL DAMAGES (INCLUDING, BUT NOT
+LIMITED TO, PROCUREMENT OF SUBSTITUTE GOODS OR SERVICES; LOSS OF USE,
+DATA, OR PROFITS; OR BUSINESS INTERRUPTION) HOWEVER CAUSED AND ON ANY
+THEORY OF LIABILITY, WHETHER IN CONTRACT, STRICT LIABILITY, OR TORT
+(INCLUDING NEGLIGENCE OR OTHERWISE) ARISING IN ANY WAY OUT OF THE USE
+OF THIS SOFTWARE, EVEN IF ADVISED OF THE POSSIBILITY OF SUCH DAMAGE.
diff --git a/keras_cv/conftest.py b/conftest.py
similarity index 97%
rename from keras_cv/conftest.py
rename to conftest.py
index eaee5024b9..6d5630df53 100644
--- a/keras_cv/conftest.py
+++ b/conftest.py
@@ -12,7 +12,6 @@
# See the License for the specific language governing permissions and
# limitations under the License.
-import keras_core
import pytest
import tensorflow as tf
from packaging import version
@@ -101,7 +100,7 @@ def pytest_collection_modifyitems(config, items):
reason="This test is only supported on Keras 2",
)
skip_tf_only = pytest.mark.skipif(
- keras_3() and keras_core.backend.backend() != "tensorflow",
+ keras_3() and backend_config.backend() != "tensorflow",
reason="This test is only supported on TensorFlow",
)
for item in items:
diff --git a/keras_cv/custom_ops/BUILD b/keras_cv/custom_ops/BUILD
index 37b551dfbe..dcf45ab878 100644
--- a/keras_cv/custom_ops/BUILD
+++ b/keras_cv/custom_ops/BUILD
@@ -1,6 +1,7 @@
-licenses(["notice"]) # Apache 2.0
-
-package(default_visibility = ["//visibility:public"])
+package(
+ default_visibility = ["//visibility:public"],
+ licenses = ["notice"],
+)
config_setting(
name = "windows",
@@ -11,38 +12,68 @@ cc_library(
name = "box_util",
srcs = ["box_util.cc"],
hdrs = ["box_util.h"],
+ copts = select({
+ ":windows": [
+ "/DEIGEN_STRONG_INLINE=inline",
+ "-DTENSORFLOW_MONOLITHIC_BUILD",
+ "/DPLATFORM_WINDOWS",
+ "/DEIGEN_HAS_C99_MATH",
+ "/DTENSORFLOW_USE_EIGEN_THREADPOOL",
+ "/DEIGEN_AVOID_STL_ARRAY",
+ "/Iexternal/gemmlowp",
+ "/wd4018",
+ "/wd4577",
+ "/DNOGDI",
+ "/UTF_COMPILE_LIBRARY",
+ ],
+ "//conditions:default": [
+ "-pthread",
+ "-std=c++17",
+ ],
+ }),
deps = [
"@local_config_tf//:libtensorflow_framework",
"@local_config_tf//:tf_header_lib",
],
- copts = select({
- ":windows": ["/DEIGEN_STRONG_INLINE=inline", "-DTENSORFLOW_MONOLITHIC_BUILD", "/DPLATFORM_WINDOWS", "/DEIGEN_HAS_C99_MATH", "/DTENSORFLOW_USE_EIGEN_THREADPOOL", "/DEIGEN_AVOID_STL_ARRAY", "/Iexternal/gemmlowp", "/wd4018", "/wd4577", "/DNOGDI", "/UTF_COMPILE_LIBRARY"],
- "//conditions:default": ["-pthread", "-std=c++17"],
- }),
)
cc_binary(
- name = '_keras_cv_custom_ops.so',
+ name = "_keras_cv_custom_ops.so",
srcs = [
"kernels/pairwise_iou_kernel.cc",
- "ops/pairwise_iou_op.cc",
- "kernels/withinbox_op.cc",
- "ops/withinbox_op.cc",
"kernels/within_any_box_op.cc",
+ "kernels/withinbox_op.cc",
+ "ops/pairwise_iou_op.cc",
"ops/within_any_box_op.cc",
+ "ops/withinbox_op.cc",
],
+ copts = select({
+ ":windows": [
+ "/DEIGEN_STRONG_INLINE=inline",
+ "-DTENSORFLOW_MONOLITHIC_BUILD",
+ "/DPLATFORM_WINDOWS",
+ "/DEIGEN_HAS_C99_MATH",
+ "/DTENSORFLOW_USE_EIGEN_THREADPOOL",
+ "/DEIGEN_AVOID_STL_ARRAY",
+ "/Iexternal/gemmlowp",
+ "/wd4018",
+ "/wd4577",
+ "/DNOGDI",
+ "/UTF_COMPILE_LIBRARY",
+ ],
+ "//conditions:default": [
+ "-pthread",
+ "-std=c++17",
+ ],
+ }),
+ features = select({
+ ":windows": ["windows_export_all_symbols"],
+ "//conditions:default": [],
+ }),
linkshared = 1,
deps = [
+ ":box_util",
"@local_config_tf//:libtensorflow_framework",
"@local_config_tf//:tf_header_lib",
- ":box_util",
],
- features = select({
- ":windows": ["windows_export_all_symbols"],
- "//conditions:default": [],
- }),
- copts = select({
- ":windows": ["/DEIGEN_STRONG_INLINE=inline", "-DTENSORFLOW_MONOLITHIC_BUILD", "/DPLATFORM_WINDOWS", "/DEIGEN_HAS_C99_MATH", "/DTENSORFLOW_USE_EIGEN_THREADPOOL", "/DEIGEN_AVOID_STL_ARRAY", "/Iexternal/gemmlowp", "/wd4018", "/wd4577", "/DNOGDI", "/UTF_COMPILE_LIBRARY"],
- "//conditions:default": ["-pthread", "-std=c++17"],
- }),
)
diff --git a/keras_cv/layers/object_detection/roi_pool.py b/keras_cv/layers/object_detection/roi_pool.py
index 34b7c0fd08..5d054630c6 100644
--- a/keras_cv/layers/object_detection/roi_pool.py
+++ b/keras_cv/layers/object_detection/roi_pool.py
@@ -110,11 +110,12 @@ def _pool_single_sample(self, args):
feature_map: [H, W, C] float Tensor
rois: [N, 4] float Tensor
Returns:
- pooled_feature_map: [target_size, C] float Tensor
+ pooled_feature_map: [N, target_height, target_width, C] float Tensor
"""
feature_map, rois = args
- num_rois = ops.shape(rois)[0]
- height, width, channel = ops.shape(feature_map)
+ num_rois = rois.get_shape().as_list()[0]
+ height, width, channel = feature_map.get_shape().as_list()
+ regions = []
# TODO (consider vectorize it for better performance)
for n in range(num_rois):
# [4]
@@ -125,7 +126,7 @@ def _pool_single_sample(self, args):
region_width = width * (roi[3] - roi[1])
h_step = region_height / self.target_height
w_step = region_width / self.target_width
- regions = []
+ region_steps = []
for i in range(self.target_height):
for j in range(self.target_width):
height_start = y_start + i * h_step
@@ -145,16 +146,18 @@ def _pool_single_sample(self, args):
1, width_end - width_start
)
# [h_step, w_step, C]
- region = feature_map[
+ region_step = feature_map[
height_start:height_end, width_start:width_end, :
]
# target_height * target_width * [C]
- regions.append(ops.max(region, axis=[0, 1]))
- regions = ops.reshape(
- ops.stack(regions),
- [self.target_height, self.target_width, channel],
+ region_steps.append(ops.max(region_step, axis=[0, 1]))
+ regions.append(
+ ops.reshape(
+ ops.stack(region_steps),
+ [self.target_height, self.target_width, channel],
+ )
)
- return regions
+ return ops.stack(regions)
def get_config(self):
config = {
diff --git a/keras_cv/layers/object_detection/roi_pool_test.py b/keras_cv/layers/object_detection/roi_pool_test.py
index da367595c2..be51f121e1 100644
--- a/keras_cv/layers/object_detection/roi_pool_test.py
+++ b/keras_cv/layers/object_detection/roi_pool_test.py
@@ -41,7 +41,7 @@ def test_no_quantize(self):
# | 56, 57, 58, 59(max) | 60, 61, 62, 63(max) |
# --------------------------------------------
expected_feature_map = np.reshape(
- np.array([27, 31, 59, 63]), [1, 2, 2, 1]
+ np.array([27, 31, 59, 63]), [1, 1, 2, 2, 1]
)
self.assertAllClose(expected_feature_map, pooled_feature_map)
@@ -67,7 +67,7 @@ def test_roi_quantize_y(self):
# | 56, 57, 58(max) | 59, 60, 61, 62(max) | 63 (removed)
# --------------------------------------------
expected_feature_map = np.reshape(
- np.array([26, 30, 58, 62]), [1, 2, 2, 1]
+ np.array([26, 30, 58, 62]), [1, 1, 2, 2, 1]
)
self.assertAllClose(expected_feature_map, pooled_feature_map)
@@ -92,7 +92,7 @@ def test_roi_quantize_x(self):
# | 48, 49, 50, 51(max) | 52, 53, 54, 55(max) |
# --------------------------------------------
expected_feature_map = np.reshape(
- np.array([19, 23, 51, 55]), [1, 2, 2, 1]
+ np.array([19, 23, 51, 55]), [1, 1, 2, 2, 1]
)
self.assertAllClose(expected_feature_map, pooled_feature_map)
@@ -119,7 +119,7 @@ def test_roi_quantize_h(self):
# | 56, 57, 58, 59(max) | 60, 61, 62, 63(max) |
# --------------------------------------------
expected_feature_map = np.reshape(
- np.array([11, 15, 35, 39, 59, 63]), [1, 3, 2, 1]
+ np.array([11, 15, 35, 39, 59, 63]), [1, 1, 3, 2, 1]
)
self.assertAllClose(expected_feature_map, pooled_feature_map)
@@ -145,7 +145,7 @@ def test_roi_quantize_w(self):
# | 56, 57(max) | 58, 59, 60(max) | 61, 62, 63(max) |
# --------------------------------------------
expected_feature_map = np.reshape(
- np.array([25, 28, 31, 57, 60, 63]), [1, 2, 3, 1]
+ np.array([25, 28, 31, 57, 60, 63]), [1, 1, 2, 3, 1]
)
self.assertAllClose(expected_feature_map, pooled_feature_map)
@@ -166,7 +166,8 @@ def test_roi_feature_map_height_smaller_than_roi(self):
# ------------------repeated----------------------
# | 12, 13(max) | 14, 15(max) |
expected_feature_map = np.reshape(
- np.array([1, 3, 1, 3, 5, 7, 9, 11, 9, 11, 13, 15]), [1, 6, 2, 1]
+ np.array([1, 3, 1, 3, 5, 7, 9, 11, 9, 11, 13, 15]),
+ [1, 1, 6, 2, 1],
)
self.assertAllClose(expected_feature_map, pooled_feature_map)
@@ -187,7 +188,7 @@ def test_roi_feature_map_width_smaller_than_roi(self):
# --------------------------------------------
expected_feature_map = np.reshape(
np.array([4, 4, 5, 6, 6, 7, 12, 12, 13, 14, 14, 15]),
- [1, 2, 6, 1],
+ [1, 1, 2, 6, 1],
)
self.assertAllClose(expected_feature_map, pooled_feature_map)
@@ -201,10 +202,43 @@ def test_roi_empty(self):
rois = np.reshape(np.array([0.0, 0.0, 0.0, 0.0]), [1, 1, 4])
pooled_feature_map = roi_pooler(feature_map, rois)
# all outputs should be top-left pixel
- self.assertAllClose(np.ones([1, 2, 2, 1]), pooled_feature_map)
+ self.assertAllClose(np.ones([1, 1, 2, 2, 1]), pooled_feature_map)
def test_invalid_image_shape(self):
with self.assertRaisesRegex(ValueError, "dynamic shape"):
_ = ROIPooler(
"rel_yxyx", target_size=[2, 2], image_shape=[None, 224, 3]
)
+
+ def test_multiple_rois(self):
+ feature_map = np.expand_dims(
+ np.reshape(np.arange(0, 64), [8, 8, 1]), axis=0
+ )
+
+ roi_pooler = ROIPooler(
+ bounding_box_format="yxyx",
+ target_size=[2, 2],
+ image_shape=[224, 224, 3],
+ )
+ rois = np.array(
+ [[[0.0, 0.0, 112.0, 112.0], [0.0, 112.0, 224.0, 224.0]]],
+ )
+
+ pooled_feature_map = roi_pooler(feature_map, rois)
+ # the maximum value would be at bottom-right at each block, roi sharded
+ # into 2x2 blocks
+ # | 0, 1, 2, 3 | 4, 5, 6, 7 |
+ # | 8, 9, 10, 11 | 12, 13, 14, 15 |
+ # | 16, 17, 18, 19 | 20, 21, 22, 23 |
+ # | 24, 25, 26, 27(max) | 28, 29, 30, 31(max) |
+ # --------------------------------------------
+ # | 32, 33, 34, 35 | 36, 37, 38, 39 |
+ # | 40, 41, 42, 43 | 44, 45, 46, 47 |
+ # | 48, 49, 50, 51 | 52, 53, 54, 55 |
+ # | 56, 57, 58, 59(max) | 60, 61, 62, 63(max) |
+ # --------------------------------------------
+
+ expected_feature_map = np.reshape(
+ np.array([9, 11, 25, 27, 29, 31, 61, 63]), [1, 2, 2, 2, 1]
+ )
+ self.assertAllClose(expected_feature_map, pooled_feature_map)
diff --git a/keras_cv/models/__init__.py b/keras_cv/models/__init__.py
index b9b90b946a..77c3ad33d9 100644
--- a/keras_cv/models/__init__.py
+++ b/keras_cv/models/__init__.py
@@ -178,11 +178,13 @@
from keras_cv.models.backbones.resnet_v2.resnet_v2_backbone import (
ResNetV2Backbone,
)
+from keras_cv.models.backbones.vgg16.vgg16_backbone import VGG16Backbone
from keras_cv.models.backbones.vit_det.vit_det_aliases import ViTDetBBackbone
from keras_cv.models.backbones.vit_det.vit_det_aliases import ViTDetHBackbone
from keras_cv.models.backbones.vit_det.vit_det_aliases import ViTDetLBackbone
from keras_cv.models.backbones.vit_det.vit_det_backbone import ViTDetBackbone
from keras_cv.models.classification.image_classifier import ImageClassifier
+from keras_cv.models.feature_extractor.clip import CLIP
from keras_cv.models.object_detection.retinanet.retinanet import RetinaNet
from keras_cv.models.object_detection.yolo_v8.yolo_v8_backbone import (
YOLOV8Backbone,
diff --git a/keras_cv/models/backbones/vgg16/__init__.py b/keras_cv/models/backbones/vgg16/__init__.py
new file mode 100644
index 0000000000..3992ffb59a
--- /dev/null
+++ b/keras_cv/models/backbones/vgg16/__init__.py
@@ -0,0 +1,13 @@
+# Copyright 2023 The KerasCV Authors
+#
+# Licensed under the Apache License, Version 2.0 (the "License");
+# you may not use this file except in compliance with the License.
+# You may obtain a copy of the License at
+#
+# https://www.apache.org/licenses/LICENSE-2.0
+#
+# Unless required by applicable law or agreed to in writing, software
+# distributed under the License is distributed on an "AS IS" BASIS,
+# WITHOUT WARRANTIES OR CONDITIONS OF ANY KIND, either express or implied.
+# See the License for the specific language governing permissions and
+# limitations under the License.
diff --git a/keras_cv/models/backbones/vgg16/vgg16_backbone.py b/keras_cv/models/backbones/vgg16/vgg16_backbone.py
new file mode 100644
index 0000000000..901ab0d582
--- /dev/null
+++ b/keras_cv/models/backbones/vgg16/vgg16_backbone.py
@@ -0,0 +1,219 @@
+# Copyright 2023 The KerasCV Authors
+#
+# Licensed under the Apache License, Version 2.0 (the "License");
+# you may not use this file except in compliance with the License.
+# You may obtain a copy of the License at
+#
+# https://www.apache.org/licenses/LICENSE-2.0
+#
+# Unless required by applicable law or agreed to in writing, software
+# distributed under the License is distributed on an "AS IS" BASIS,
+# WITHOUT WARRANTIES OR CONDITIONS OF ANY KIND, either express or implied.
+# See the License for the specific language governing permissions and
+# limitations under the License.
+
+from keras import layers
+
+from keras_cv.models import utils
+from keras_cv.models.backbones.backbone import Backbone
+
+
+class VGG16Backbone(Backbone):
+ """
+ Reference:
+ - [Very Deep Convolutional Networks for Large-Scale Image Recognition](https://arxiv.org/abs/1409.1556)
+ (ICLR 2015)
+ This class represents Keras Backbone of VGG16 model.
+ Args:
+ include_rescaling: bool, whether to rescale the inputs. If set to
+ True, inputs will be passed through a `Rescaling(1/255.0)` layer.
+ include_top: bool, whether to include the 3 fully-connected
+ layers at the top of the network. If provided, num_classes must be
+ provided.
+ num_classes: int, optional number of classes to classify images into,
+ only to be specified if `include_top` is True.
+ input_shape: tuple, optional shape tuple, defaults to (224, 224, 3).
+ input_tensor: Tensor, optional Keras tensor (i.e. output of
+ `layers.Input()`) to use as image input for the model.
+ pooling: bool, Optional pooling mode for feature extraction
+ when `include_top` is `False`.
+ - `None` means that the output of the model will be
+ the 4D tensor output of the
+ last convolutional block.
+ - `avg` means that global average pooling
+ will be applied to the output of the
+ last convolutional block, and thus
+ the output of the model will be a 2D tensor.
+ - `max` means that global max pooling will
+ be applied.
+ classifier_activation:`str` or callable. The activation function to use
+ on the "top" layer. Ignored unless `include_top=True`. Set
+ `classifier_activation=None` to return the logits of the "top" layer.
+ When loading pretrained weights, `classifier_activation` can only
+ be `None` or `"softmax"`.
+ name: (Optional) name to pass to the model, defaults to "VGG16".
+ Returns:
+ A `keras.Model` instance.
+ """ # noqa: E501
+
+ def __init__(
+ self,
+ include_rescaling,
+ include_top,
+ input_tensor=None,
+ num_classes=None,
+ input_shape=(224, 224, 3),
+ pooling=None,
+ classifier_activation="softmax",
+ name="VGG16",
+ **kwargs,
+ ):
+
+ if include_top and num_classes is None:
+ raise ValueError(
+ "If `include_top` is True, you should specify `num_classes`. "
+ f"Received: num_classes={num_classes}"
+ )
+
+ if include_top and pooling:
+ raise ValueError(
+ f"`pooling` must be `None` when `include_top=True`."
+ f"Received pooling={pooling} and include_top={include_top}. "
+ )
+
+ img_input = utils.parse_model_inputs(input_shape, input_tensor)
+ x = img_input
+
+ if include_rescaling:
+ x = layers.Rescaling(scale=1 / 255.0)(x)
+
+ x = apply_vgg_block(
+ x=x,
+ num_layers=2,
+ filters=64,
+ kernel_size=(3, 3),
+ activation="relu",
+ padding="same",
+ max_pool=True,
+ name="block1",
+ )
+
+ x = apply_vgg_block(
+ x=x,
+ num_layers=2,
+ filters=128,
+ kernel_size=(3, 3),
+ activation="relu",
+ padding="same",
+ max_pool=True,
+ name="block2",
+ )
+
+ x = apply_vgg_block(
+ x=x,
+ num_layers=3,
+ filters=256,
+ kernel_size=(3, 3),
+ activation="relu",
+ padding="same",
+ max_pool=True,
+ name="block3",
+ )
+
+ x = apply_vgg_block(
+ x=x,
+ num_layers=3,
+ filters=512,
+ kernel_size=(3, 3),
+ activation="relu",
+ padding="same",
+ max_pool=True,
+ name="block4",
+ )
+
+ x = apply_vgg_block(
+ x=x,
+ num_layers=3,
+ filters=512,
+ kernel_size=(3, 3),
+ activation="relu",
+ padding="same",
+ max_pool=True,
+ name="block5",
+ )
+
+ if include_top:
+ x = layers.Flatten(name="flatten")(x)
+ x = layers.Dense(4096, activation="relu", name="fc1")(x)
+ x = layers.Dense(4096, activation="relu", name="fc2")(x)
+ x = layers.Dense(
+ num_classes,
+ activation=classifier_activation,
+ name="predictions",
+ )(x)
+ else:
+ if pooling == "avg":
+ x = layers.GlobalAveragePooling2D()(x)
+ elif pooling == "max":
+ x = layers.GlobalMaxPooling2D()(x)
+
+ super().__init__(inputs=img_input, outputs=x, name=name, **kwargs)
+
+ self.include_rescaling = include_rescaling
+ self.include_top = include_top
+ self.num_classes = num_classes
+ self.input_tensor = input_tensor
+ self.pooling = pooling
+ self.classifier_activation = classifier_activation
+
+ def get_config(self):
+ return {
+ "include_rescaling": self.include_rescaling,
+ "include_top": self.include_top,
+ "name": self.name,
+ "input_shape": self.input_shape[1:],
+ "input_tensor": self.input_tensor,
+ "pooling": self.pooling,
+ "num_classes": self.num_classes,
+ "classifier_activation": self.classifier_activation,
+ "trainable": self.trainable,
+ }
+
+
+def apply_vgg_block(
+ x,
+ num_layers,
+ filters,
+ kernel_size,
+ activation,
+ padding,
+ max_pool,
+ name,
+):
+ """
+ Applies VGG block
+ Args:
+ x: Tensor, input tensor to pass through network
+ num_layers: int, number of CNN layers in the block
+ filters: int, filter size of each CNN layer in block
+ kernel_size: int (or) tuple, kernel size for CNN layer in block
+ activation: str (or) callable, activation function for each CNN layer in
+ block
+ padding: str (or) callable, padding function for each CNN layer in block
+ max_pool: bool, whether to add MaxPooling2D layer at end of block
+ name: str, name of the block
+
+ Returns:
+ keras.KerasTensor
+ """
+ for num in range(1, num_layers + 1):
+ x = layers.Conv2D(
+ filters,
+ kernel_size,
+ activation=activation,
+ padding=padding,
+ name=f"{name}_conv{num}",
+ )(x)
+ if max_pool:
+ x = layers.MaxPooling2D((2, 2), (2, 2), name=f"{name}_pool")(x)
+ return x
diff --git a/keras_cv/models/backbones/vgg16/vgg16_backbone_test.py b/keras_cv/models/backbones/vgg16/vgg16_backbone_test.py
new file mode 100644
index 0000000000..d7a8c9724f
--- /dev/null
+++ b/keras_cv/models/backbones/vgg16/vgg16_backbone_test.py
@@ -0,0 +1,75 @@
+# Copyright 2023 The KerasCV Authors
+#
+# Licensed under the Apache License, Version 2.0 (the "License");
+# you may not use this file except in compliance with the License.
+# You may obtain a copy of the License at
+#
+# https://www.apache.org/licenses/LICENSE-2.0
+#
+# Unless required by applicable law or agreed to in writing, software
+# distributed under the License is distributed on an "AS IS" BASIS,
+# WITHOUT WARRANTIES OR CONDITIONS OF ANY KIND, either express or implied.
+# See the License for the specific language governing permissions and
+# limitations under the License.
+
+import os
+
+import numpy as np
+import pytest
+
+from keras_cv.backend import keras
+from keras_cv.models import VGG16Backbone
+from keras_cv.tests.test_case import TestCase
+
+
+class VGG16BackboneTest(TestCase):
+ def setUp(self):
+ self.img_input = np.ones((2, 224, 224, 3), dtype="float32")
+
+ def test_valid_call(self):
+ model = VGG16Backbone(
+ input_shape=(224, 224, 3),
+ include_top=False,
+ include_rescaling=False,
+ pooling="avg",
+ )
+ model(self.img_input)
+
+ def test_valid_call_with_rescaling(self):
+ model = VGG16Backbone(
+ input_shape=(224, 224, 3),
+ include_top=False,
+ include_rescaling=True,
+ pooling="avg",
+ )
+ model(self.img_input)
+
+ def test_valid_call_with_top(self):
+ model = VGG16Backbone(
+ input_shape=(224, 224, 3),
+ include_top=True,
+ include_rescaling=False,
+ num_classes=2,
+ )
+ model(self.img_input)
+
+ @pytest.mark.large
+ def test_saved_model(self):
+ model = VGG16Backbone(
+ input_shape=(224, 224, 3),
+ include_top=False,
+ include_rescaling=False,
+ num_classes=2,
+ pooling="avg",
+ )
+ model_output = model(self.img_input)
+ save_path = os.path.join(self.get_temp_dir(), "vgg16.keras")
+ model.save(save_path)
+ restored_model = keras.models.load_model(save_path)
+
+ # Check the restored model is instance of VGG16Backbone
+ self.assertIsInstance(restored_model, VGG16Backbone)
+
+ # Check if the restored model gives the same output
+ restored_model_output = restored_model(self.img_input)
+ self.assertAllClose(model_output, restored_model_output)
diff --git a/keras_cv/models/feature_extractor/__init__.py b/keras_cv/models/feature_extractor/__init__.py
new file mode 100644
index 0000000000..3992ffb59a
--- /dev/null
+++ b/keras_cv/models/feature_extractor/__init__.py
@@ -0,0 +1,13 @@
+# Copyright 2023 The KerasCV Authors
+#
+# Licensed under the Apache License, Version 2.0 (the "License");
+# you may not use this file except in compliance with the License.
+# You may obtain a copy of the License at
+#
+# https://www.apache.org/licenses/LICENSE-2.0
+#
+# Unless required by applicable law or agreed to in writing, software
+# distributed under the License is distributed on an "AS IS" BASIS,
+# WITHOUT WARRANTIES OR CONDITIONS OF ANY KIND, either express or implied.
+# See the License for the specific language governing permissions and
+# limitations under the License.
diff --git a/keras_cv/models/feature_extractor/clip/__init__.py b/keras_cv/models/feature_extractor/clip/__init__.py
new file mode 100644
index 0000000000..8826871115
--- /dev/null
+++ b/keras_cv/models/feature_extractor/clip/__init__.py
@@ -0,0 +1,23 @@
+# Copyright 2023 The KerasCV Authors
+#
+# Licensed under the Apache License, Version 2.0 (the "License");
+# you may not use this file except in compliance with the License.
+# You may obtain a copy of the License at
+#
+# https://www.apache.org/licenses/LICENSE-2.0
+#
+# Unless required by applicable law or agreed to in writing, software
+# distributed under the License is distributed on an "AS IS" BASIS,
+# WITHOUT WARRANTIES OR CONDITIONS OF ANY KIND, either express or implied.
+# See the License for the specific language governing permissions and
+# limitations under the License.
+
+from keras_cv.models.feature_extractor.clip.clip_image_model import (
+ CLIPImageEncoder,
+)
+from keras_cv.models.feature_extractor.clip.clip_model import CLIP
+from keras_cv.models.feature_extractor.clip.clip_processor import CLIPProcessor
+from keras_cv.models.feature_extractor.clip.clip_text_model import (
+ CLIPTextEncoder,
+)
+from keras_cv.models.feature_extractor.clip.clip_tokenizer import CLIPTokenizer
diff --git a/keras_cv/models/feature_extractor/clip/clip_encoder.py b/keras_cv/models/feature_extractor/clip/clip_encoder.py
new file mode 100644
index 0000000000..aeb345c857
--- /dev/null
+++ b/keras_cv/models/feature_extractor/clip/clip_encoder.py
@@ -0,0 +1,321 @@
+# Copyright 2023 The KerasCV Authors
+#
+# Licensed under the Apache License, Version 2.0 (the "License");
+# you may not use this file except in compliance with the License.
+# You may obtain a copy of the License at
+#
+# https://www.apache.org/licenses/LICENSE-2.0
+#
+# Unless required by applicable law or agreed to in writing, software
+# distributed under the License is distributed on an "AS IS" BASIS,
+# WITHOUT WARRANTIES OR CONDITIONS OF ANY KIND, either express or implied.
+# See the License for the specific language governing permissions and
+# limitations under the License.
+import numpy as np
+
+from keras_cv.backend import keras
+from keras_cv.backend import ops
+
+
+def get_initializer(initializer_range=0.02):
+ """
+ Creates a `keras.initializers.TruncatedNormal` with the given range.
+
+ Args:
+ initializer_range (*float*, defaults to 0.02): Standard deviation of the
+ initializer range.
+
+ Returns:
+ `keras.initializers.TruncatedNormal`: The truncated normal initializer.
+ """
+ return keras.initializers.TruncatedNormal(stddev=initializer_range)
+
+
+class QuickGELU(keras.layers.Layer):
+ def __init__(self, **kwargs):
+ super().__init__(**kwargs)
+
+ def call(self, x):
+ return x * ops.sigmoid(1.702 * x)
+
+
+class ResidualAttention(keras.layers.Layer):
+ def __init__(
+ self,
+ proj_dim,
+ num_heads,
+ num_hidden_layers,
+ **kwargs,
+ ):
+ super().__init__(**kwargs)
+ self.proj_dim = proj_dim
+ self.num_heads = num_heads
+ self.num_hidden_layers = num_hidden_layers
+ self.fc_std = np.power(2 * self.proj_dim, -0.5) * 0.02
+
+ self.in_proj_std = (
+ np.power(self.proj_dim, -0.5)
+ * (np.power(2 * self.num_hidden_layers, -0.5))
+ * 0.02
+ )
+ self.attn = CLIPAttention(
+ self.proj_dim,
+ self.num_heads,
+ self.num_hidden_layers,
+ name="multi_head_attention",
+ )
+ self.ln_1 = keras.layers.LayerNormalization(epsilon=1e-5, name="ln_1")
+ self.mlp_dense_1 = keras.layers.Dense(
+ self.proj_dim * 4,
+ name="c_fc",
+ )
+ self.mlp_activation = QuickGELU(name="gelu")
+ self.mlp_dense_2 = keras.layers.Dense(
+ self.proj_dim,
+ name="c_proj",
+ )
+ self.ln_2 = keras.layers.LayerNormalization(epsilon=1e-5, name="ln_2")
+
+ def attention(self, x, causal_attention_mask=None, attention_mask=None):
+ mask = None
+ if causal_attention_mask is not None:
+ mask = (
+ ops.cast(causal_attention_mask, dtype=x.dtype)
+ if causal_attention_mask is not None
+ else None
+ )
+ if attention_mask is not None:
+ attention_mask = (
+ ops.cast(attention_mask, dtype=x.dtype)
+ if attention_mask is not None
+ else None
+ )
+ mask = ops.add(causal_attention_mask, attention_mask)
+
+ return self.attn(
+ x,
+ attention_mask=mask,
+ )[0]
+
+ def build(self, input_shape):
+ super().build(input_shape)
+ self.attn.build(None)
+ self.ln_1.build([None, None, self.proj_dim])
+ self.mlp_dense_1.build([None, None, self.proj_dim])
+ self.mlp_dense_2.build([None, None, self.proj_dim * 4])
+ self.ln_2.build([None, None, self.proj_dim])
+
+ def call(self, x, causal_attention_mask=None, attention_mask=None):
+ residual = x
+ x = self.ln_1(x)
+ x = self.attention(
+ x,
+ causal_attention_mask=causal_attention_mask,
+ attention_mask=attention_mask,
+ )
+ x = x + residual
+ residual = x
+ x = self.mlp_dense_1(self.ln_2(residual))
+ x = self.mlp_activation(x)
+ x = self.mlp_dense_2(x)
+ x = residual + x
+ return x
+
+ def compute_output_shape(self, inputs_shape):
+ return inputs_shape
+
+ def get_config(self):
+ config = super().get_config()
+ config.update(
+ {
+ "proj_dim": self.proj_dim,
+ "num_heads": self.num_heads,
+ "num_hidden_layers": self.num_hidden_layers,
+ }
+ )
+ return config
+
+
+class CLIPEncoder(keras.layers.Layer):
+ def __init__(self, width, num_layers, heads, **kwargs):
+ super().__init__(**kwargs)
+ self.width = width
+ self.num_layers = num_layers
+ self.heads = heads
+ self.resblocks = [
+ ResidualAttention(
+ self.width,
+ self.heads,
+ self.num_layers,
+ )
+ for _ in range(self.num_layers)
+ ]
+
+ def build(self, input_shape):
+ super().build(input_shape)
+ for block in self.resblocks:
+ block.build(input_shape)
+
+ def call(
+ self,
+ x,
+ causal_attention_mask=None,
+ attention_mask=None,
+ ):
+ for block in self.resblocks:
+ x = block(
+ x,
+ causal_attention_mask=causal_attention_mask,
+ attention_mask=attention_mask,
+ )
+ return x
+
+ def compute_output_shape(self, inputs_shape):
+ return inputs_shape
+
+ def get_config(self):
+ config = super().get_config()
+ config.update(
+ {
+ "width": self.width,
+ "num_layers": self.num_layers,
+ "heads": self.heads,
+ }
+ )
+ return config
+
+
+class CLIPAttention(keras.layers.Layer):
+ """
+ Adapted from https://github.com/huggingface/transformers/blob/main/src/transformers/models/clip/modeling_clip.py # noqa: E501
+ """
+
+ def __init__(
+ self, proj_dim, num_heads, num_hidden_layers, dropout=0.0, **kwargs
+ ):
+ super().__init__(**kwargs)
+
+ self.proj_dim = proj_dim
+ self.num_heads = num_heads
+ self.num_hidden_layers = num_hidden_layers
+ self.dropout = dropout
+ self.head_dim = self.proj_dim // self.num_heads
+ if self.head_dim * self.num_heads != self.proj_dim:
+ raise ValueError(
+ f"proj_dim must be divisible by num_heads (got `proj_dim`"
+ f": {self.proj_dim} and `num_heads`:"
+ f" {self.num_heads})."
+ )
+
+ self.scale = self.head_dim**-0.5
+ in_proj_std = (
+ (self.proj_dim**-0.5)
+ * ((2 * self.num_hidden_layers) ** -0.5)
+ * 0.02
+ )
+ out_proj_std = (self.proj_dim**-0.5) * 0.02
+ self.q_proj = keras.layers.Dense(
+ units=self.proj_dim,
+ kernel_initializer=get_initializer(in_proj_std),
+ name="q_proj",
+ )
+ self.k_proj = keras.layers.Dense(
+ units=self.proj_dim,
+ kernel_initializer=get_initializer(in_proj_std),
+ name="k_proj",
+ )
+ self.v_proj = keras.layers.Dense(
+ units=self.proj_dim,
+ kernel_initializer=get_initializer(in_proj_std),
+ name="v_proj",
+ )
+ self.out_proj = keras.layers.Dense(
+ units=self.proj_dim,
+ kernel_initializer=get_initializer(out_proj_std),
+ name="out_proj",
+ )
+
+ def build(self, input_shape):
+ super().build(input_shape)
+ self.q_proj.build([None, None, self.proj_dim])
+ self.k_proj.build([None, None, self.proj_dim])
+ self.v_proj.build([None, None, self.proj_dim])
+ self.out_proj.build([None, None, self.proj_dim])
+
+ def _transpose_for_scores(self, tensor, batch_size):
+ """
+ Adapted from https://github.com/huggingface/transformers/blob/8e164c5400b7b413c7b8fb32e35132001effc970/src/transformers/models/bert/modeling_tf_bert.py#L252 # noqa: E501
+ """
+ # [batch_size, seq_len, all_head_dim] ->
+ # [batch_size, seq_len, num_heads, head_dim]
+ tensor = ops.reshape(
+ tensor, (batch_size, -1, self.num_heads, self.head_dim)
+ )
+ # [batch_size, seq_len, num_heads, head_dim] ->
+ # [batch_size, num_heads, seq_len, head_dim]
+ return ops.transpose(tensor, axes=[0, 2, 1, 3])
+
+ def call(
+ self,
+ x,
+ attention_mask=None,
+ output_attentions=None,
+ training=False,
+ ):
+ batch_size = ops.shape(x)[0]
+ mixed_query_layer = self.q_proj(inputs=x)
+ mixed_key_layer = self.k_proj(inputs=x)
+ mixed_value_layer = self.v_proj(inputs=x)
+ query_layer = self._transpose_for_scores(mixed_query_layer, batch_size)
+ key_layer = self._transpose_for_scores(mixed_key_layer, batch_size)
+ value_layer = self._transpose_for_scores(mixed_value_layer, batch_size)
+
+ # Scaled dot product between key and query = raw attention scores.
+ attention_scores = ops.matmul(
+ query_layer, ops.transpose(key_layer, axes=[0, 1, 3, 2])
+ )
+ dk = ops.cast(ops.sqrt(self.head_dim), dtype=attention_scores.dtype)
+ attention_scores = ops.divide(
+ attention_scores, dk
+ ) # (batch_size, num_heads, seq_len_q, seq_len_k)
+
+ if attention_mask is not None:
+ # Apply the attention mask (precomputed for all layers in the
+ # call() function)
+ attention_scores = ops.add(attention_scores, attention_mask)
+
+ # Normalize the attention scores to probabilities.
+ attention_probs = ops.softmax(attention_scores, axis=-1)
+
+ # This is actually dropping out entire tokens to attend to, which might
+ # seem a bit unusual, but is taken from the original Transformer paper.
+ dropout_attention_probs = keras.layers.Dropout(self.dropout)(
+ inputs=attention_probs, training=training
+ )
+
+ attn_output = ops.matmul(dropout_attention_probs, value_layer)
+ attn_output = ops.transpose(attn_output, axes=[0, 2, 1, 3])
+
+ # (batch_size, seq_len_q, proj_dim)
+ attn_output = ops.reshape(attn_output, (batch_size, -1, self.proj_dim))
+
+ attn_output = self.out_proj(attn_output, training=training)
+ outputs = (
+ (attn_output, attention_probs)
+ if output_attentions
+ else (attn_output,)
+ )
+
+ return outputs
+
+ def get_config(self):
+ config = super().get_config()
+ config.update(
+ {
+ "proj_dim": self.proj_dim,
+ "num_heads": self.num_heads,
+ "num_hidden_layers": self.num_hidden_layers,
+ "dropout": self.dropout,
+ }
+ )
+ return config
diff --git a/keras_cv/models/feature_extractor/clip/clip_image_model.py b/keras_cv/models/feature_extractor/clip/clip_image_model.py
new file mode 100644
index 0000000000..69c1002f8e
--- /dev/null
+++ b/keras_cv/models/feature_extractor/clip/clip_image_model.py
@@ -0,0 +1,170 @@
+# Copyright 2023 The KerasCV Authors
+#
+# Licensed under the Apache License, Version 2.0 (the "License");
+# you may not use this file except in compliance with the License.
+# You may obtain a copy of the License at
+#
+# https://www.apache.org/licenses/LICENSE-2.0
+#
+# Unless required by applicable law or agreed to in writing, software
+# distributed under the License is distributed on an "AS IS" BASIS,
+# WITHOUT WARRANTIES OR CONDITIONS OF ANY KIND, either express or implied.
+# See the License for the specific language governing permissions and
+# limitations under the License.
+
+from keras_cv.backend import keras
+from keras_cv.backend import ops
+from keras_cv.models.feature_extractor.clip.clip_encoder import CLIPEncoder
+from keras_cv.models.feature_extractor.clip.clip_encoder import get_initializer
+
+
+class CLIPPatchingAndEmbedding(keras.layers.Layer):
+ def __init__(
+ self, width, patch_size, input_resolution, output_dim, **kwargs
+ ):
+ super().__init__(**kwargs)
+
+ self.conv1 = keras.layers.Conv2D(
+ filters=width,
+ kernel_size=patch_size,
+ strides=patch_size,
+ padding="valid",
+ use_bias=False,
+ data_format="channels_last",
+ kernel_initializer=get_initializer(0.02),
+ name="patch_embed.embedding",
+ )
+ self.width = width
+ self.input_resolution = input_resolution
+ self.patch_size = patch_size
+ self.num_patches = ops.power(
+ (self.input_resolution // self.patch_size), 2
+ )
+ self.class_embedding_initializer = get_initializer(
+ ops.power(self.width, -0.5) * 0.02
+ )
+ self.output_dim = output_dim
+
+ def build(self, input_shape):
+ super().build(input_shape)
+ self.conv1.build(input_shape)
+ self.class_embedding = self.add_weight(
+ shape=((self.width,)),
+ initializer=self.class_embedding_initializer,
+ name="patch_embed.class_embedding",
+ )
+
+ self.positional_embedding = self.add_weight(
+ shape=(
+ (
+ (self.input_resolution // self.patch_size) ** 2 + 1,
+ self.width,
+ )
+ ),
+ trainable=True,
+ name="patch_embed.positional_embedding",
+ )
+
+ def call(self, x):
+ batch_size = ops.shape(x)[0]
+ patch_embeddings = self.conv1(x) # shape = [*, grid, grid, channel]
+
+ patch_embeddings = ops.reshape(
+ patch_embeddings, (batch_size, self.num_patches, -1)
+ )
+ class_embeds = ops.broadcast_to(
+ self.class_embedding.value, (batch_size, 1, self.width)
+ )
+ embeddings = ops.concatenate(
+ [class_embeds, patch_embeddings], axis=1
+ ) # shape = [*, grid ** 2 + 1, width]
+ positional_embedding = self.positional_embedding
+ embeddings = embeddings + positional_embedding
+ return embeddings
+
+ def get_config(self):
+ config = super().get_config()
+ config.update(
+ {
+ "width": self.width,
+ "patch_size": self.patch_size,
+ "input_resolution": self.input_resolution,
+ "output_dim": self.output_dim,
+ }
+ )
+ return config
+
+
+class CLIPImageEncoder(keras.Model):
+ def __init__(
+ self,
+ input_resolution,
+ patch_size,
+ width,
+ num_layers,
+ heads,
+ output_dim,
+ **kwargs,
+ ):
+ super().__init__(
+ **kwargs,
+ )
+ self.input_resolution = input_resolution
+ self.width = width
+ self.patch_size = patch_size
+ self.output_dim = output_dim
+ self.heads = heads
+ self.num_layers = num_layers
+
+ self.embeddings = CLIPPatchingAndEmbedding(
+ width=self.width,
+ patch_size=self.patch_size,
+ input_resolution=self.input_resolution,
+ output_dim=self.output_dim,
+ name="clip_patch_embedding",
+ )
+ self.pre_norm = keras.layers.LayerNormalization(
+ epsilon=1e-5, name="ln_1"
+ )
+ self.encoder = CLIPEncoder(
+ self.width,
+ self.num_layers,
+ self.heads,
+ name="clip_encoder",
+ )
+ self.post_norm = keras.layers.LayerNormalization(
+ epsilon=1e-5, name="ln_2"
+ )
+ self.image_projector = keras.layers.Dense(
+ output_dim, name="vision_projector", use_bias=False
+ )
+
+ def build(self, input_shape):
+ super().build(input_shape)
+ self.embeddings.build(input_shape)
+ self.pre_norm.build([None, None, self.width])
+ self.encoder.build(None)
+ self.post_norm.build([None, self.width])
+ self.image_projector.build([None, None, self.width])
+
+ def call(self, image):
+ x = self.embeddings(image)
+ x = self.pre_norm(x)
+ x = self.encoder(x)
+ x = self.post_norm(x[:, 0, :])
+ image_projected_embeddings = self.image_projector(x)
+ return image_projected_embeddings
+
+ def get_config(self):
+ config = super().get_config()
+ config.update(
+ {
+ "input_resolution": self.input_resolution,
+ "patch_size": self.patch_size,
+ "width": self.width,
+ "layers": self.num_layers,
+ "heads": self.heads,
+ "output_dim": self.output_dim,
+ }
+ )
+ return config
diff --git a/keras_cv/models/feature_extractor/clip/clip_model.py b/keras_cv/models/feature_extractor/clip/clip_model.py
new file mode 100644
index 0000000000..c3e6d49caf
--- /dev/null
+++ b/keras_cv/models/feature_extractor/clip/clip_model.py
@@ -0,0 +1,188 @@
+# Copyright 2023 The KerasCV Authors
+#
+# Licensed under the Apache License, Version 2.0 (the "License");
+# you may not use this file except in compliance with the License.
+# You may obtain a copy of the License at
+#
+# https://www.apache.org/licenses/LICENSE-2.0
+#
+# Unless required by applicable law or agreed to in writing, software
+# distributed under the License is distributed on an "AS IS" BASIS,
+# WITHOUT WARRANTIES OR CONDITIONS OF ANY KIND, either express or implied.
+# See the License for the specific language governing permissions and
+# limitations under the License.
+import copy
+
+from keras_cv.api_export import keras_cv_export
+from keras_cv.backend import keras
+from keras_cv.backend import ops
+from keras_cv.models.feature_extractor.clip.clip_image_model import (
+ CLIPImageEncoder,
+)
+from keras_cv.models.feature_extractor.clip.clip_presets import ( # noqa: E501
+ clip_presets,
+)
+from keras_cv.models.feature_extractor.clip.clip_text_model import (
+ CLIPTextEncoder,
+)
+from keras_cv.models.task import Task
+from keras_cv.utils.python_utils import classproperty
+
+try:
+ import keras_nlp
+except ImportError:
+ keras_nlp = None
+
+
+@keras_cv_export(["keras_cv.models.CLIP"])
+class CLIP(Task):
+ """
+ CLIP implements the Contrastive Language-Image Pretraining (CLIP)
+ architecture, which enables joint learning of visual and textual
+ representations for various downstream tasks. The deafult base model
+ achitecture will be set to clip-vit-base-patch32.
+
+ Args:
+ embed_dim (int): The dimensionality of the joint embedding space for
+ images and texts.
+ image_resolution (int): The resolution of the input images (both height
+ and width).
+ vision_layers (int): The number of layers in the vision (image) encoder.
+ vision_width (int): The width of the hidden layers in the vision
+ encoder.
+ vision_patch_size (int): The size of each square patch in the input
+ images.
+ context_length (int): The maximum length of the contextualized text
+ sequences.
+ vocab_size (int): The size of the vocabulary for tokenization.
+ transformer_width (int): The width of the hidden layers in the
+ transformer-based text encoder.
+ transformer_heads (int): The number of attention heads in the
+ transformer-based text encoder.
+ transformer_layers (int): The number of layers in the transformer-based
+ text encoder.
+ """
+
+ def __init__(
+ self,
+ embed_dim=512,
+ image_resolution=224,
+ vision_layers=12,
+ vision_width=768,
+ vision_patch_size=32,
+ context_length=77,
+ vocab_size=49408,
+ transformer_width=512,
+ transformer_heads=8,
+ transformer_layers=12,
+ **kwargs,
+ ):
+ super().__init__(**kwargs)
+ if keras_nlp is None:
+ raise ValueError(
+ "ClipTokenizer requires keras-nlp. Please install "
+ "using pip `pip install -U keras-nlp && pip install -U keras`"
+ )
+ self.embed_dim = embed_dim
+ self.image_resolution = image_resolution
+ self.vision_layers = vision_layers
+ self.vision_width = vision_width
+ self.vision_patch_size = vision_patch_size
+ self.context_length = context_length
+ self.vocab_size = vocab_size
+ self.transformer_width = transformer_width
+ self.transformer_heads = transformer_heads
+ self.transformer_layers = transformer_layers
+
+ vision_heads = self.vision_width // 64
+ self.image_encoder = CLIPImageEncoder(
+ input_resolution=self.image_resolution,
+ patch_size=self.vision_patch_size,
+ width=self.vision_width,
+ num_layers=self.vision_layers,
+ heads=vision_heads,
+ output_dim=self.embed_dim,
+ name="image_encoder",
+ )
+ self.text_encoder = CLIPTextEncoder(
+ transformer_width=self.transformer_width,
+ transformer_layers=self.transformer_layers,
+ transformer_heads=self.transformer_heads,
+ vocab_size=self.vocab_size,
+ embed_dim=self.embed_dim,
+ context_length=self.context_length,
+ name="text_encoder",
+ )
+
+ self.logit_scale = keras.Variable(
+ ops.ones([]) * ops.log(1 / 0.07), name="logit_scale"
+ )
+ self.image_embeddings = None
+ self.text_embeddings = None
+
+ def build(self, input_shape):
+ super().build(input_shape)
+ self.text_encoder.build([None, self.context_length])
+ self.image_encoder.build(
+ [None, self.image_resolution, self.image_resolution, 3]
+ )
+
+ def encode_images(self, image):
+ return self.image_encoder(image)
+
+ def encode_text(self, text, attention_mask=None):
+ return self.text_encoder(text, attention_mask=attention_mask)
+
+ def call(self, image, text, attention_mask=None):
+ self.image_embeddings = self.encode_images(image)
+ self.text_embeddings = self.encode_text(
+ text, attention_mask=attention_mask
+ )
+ normalize_image_features = ops.sqrt(
+ ops.sum(ops.power(self.image_embeddings, 2), keepdims=True)
+ )
+ normalize_text_features = ops.sqrt(
+ ops.sum(ops.power(self.text_embeddings, 2), keepdims=True)
+ )
+ self.image_embeddings = self.image_embeddings / normalize_image_features
+ self.text_embeddings = self.text_embeddings / normalize_text_features
+ logit_scale = ops.exp(self.logit_scale)
+ logits_per_image = (
+ ops.matmul(
+ self.image_embeddings,
+ ops.transpose(self.text_embeddings),
+ )
+ * logit_scale
+ )
+ logits_per_text = ops.transpose(logits_per_image)
+
+ return logits_per_image, logits_per_text
+
+ @classproperty
+ def presets(cls):
+ """Dictionary of preset names and configurations."""
+ return copy.deepcopy({**clip_presets})
+
+ @classproperty
+ def presets_with_weights(cls):
+ """Dictionary of preset names and configurations that include
+ weights."""
+ return copy.deepcopy({**clip_presets})
+
+ def get_config(self):
+ config = super().get_config()
+ config.update(
+ {
+ "embed_dim": self.embed_dim,
+ "image_resolution": self.image_resolution,
+ "vision_layers": self.vision_layers,
+ "vision_width": self.vision_width,
+ "vision_patch_size": self.vision_patch_size,
+ "context_length": self.context_length,
+ "vocab_size": self.vocab_size,
+ "transformer_width": self.transformer_width,
+ "transformer_heads": self.transformer_heads,
+ "transformer_layers": self.transformer_layers,
+ }
+ )
+ return config
diff --git a/keras_cv/models/feature_extractor/clip/clip_model_test.py b/keras_cv/models/feature_extractor/clip/clip_model_test.py
new file mode 100644
index 0000000000..14304b73ef
--- /dev/null
+++ b/keras_cv/models/feature_extractor/clip/clip_model_test.py
@@ -0,0 +1,135 @@
+# Copyright 2022 The KerasCV Authors
+#
+# Licensed under the Apache License, Version 2.0 (the "License");
+# you may not use this file except in compliance with the License.
+# You may obtain a copy of the License at
+#
+# https://www.apache.org/licenses/LICENSE-2.0
+#
+# Unless required by applicable law or agreed to in writing, software
+# distributed under the License is distributed on an "AS IS" BASIS,
+# WITHOUT WARRANTIES OR CONDITIONS OF ANY KIND, either express or implied.
+# See the License for the specific language governing permissions and
+# limitations under the License.
+
+import os
+
+import numpy as np
+import pytest
+from tensorflow import data as tf_data
+
+from keras_cv.backend import keras
+from keras_cv.backend import ops
+from keras_cv.backend.config import keras_3
+from keras_cv.models import CLIP
+from keras_cv.models.feature_extractor.clip import CLIPProcessor
+from keras_cv.tests.test_case import TestCase
+
+VOCAB_PATH = keras.utils.get_file(
+ None,
+ "https://storage.googleapis.com/keras-cv/models/clip/vocab.json",
+)
+MERGE_PATH = keras.utils.get_file(
+ None,
+ "https://storage.googleapis.com/keras-cv/models/clip/merges.txt",
+)
+
+MODEL_PATH = keras.utils.get_file(
+ None,
+ "https://storage.googleapis.com/keras-cv/models/clip/clip-vit-base-patch32.weights.h5", # noqa: E501
+)
+
+
+class CLIPTest(TestCase):
+ @pytest.mark.large
+ def test_clip_model_golden_values(self):
+ model = CLIP()
+ model.load_weights(MODEL_PATH)
+ processed_image = np.ones(shape=[1, 224, 224, 3])
+ processed_text = np.ones(shape=[3, 77])
+ attention_mask = np.ones(shape=[3, 77])
+ image_logits, text_logits = model(
+ processed_image, processed_text, attention_mask
+ )
+ print(image_logits)
+ self.assertAllClose(image_logits, [[1.896713, 1.896713, 1.896713]])
+ self.assertAllClose(
+ text_logits, ops.transpose([[1.896713, 1.896713, 1.896713]])
+ )
+
+ def test_clip_preprocessor(self):
+ processor = CLIPProcessor(224, VOCAB_PATH, MERGE_PATH)
+ processed_text, attention_mask = processor.process_texts(
+ ["mountains", "cat on tortoise"]
+ )
+ self.assertAllClose(
+ processed_text[:, :3], [[49406, 5873, 49407], [49406, 2368, 525]]
+ )
+ self.assertAllClose(
+ attention_mask[0, :5], [True, True, True, False, False]
+ )
+
+ def test_clip_preprocessor_tf_data(self):
+ processor = CLIPProcessor(224, VOCAB_PATH, MERGE_PATH)
+ text_input = ["a bus", "a dog", "a cat"]
+ dataset = tf_data.Dataset.from_tensor_slices(text_input)
+ dataset.map(processor.process_texts)
+
+ @pytest.mark.large
+ def test_presets(self):
+ # self.skipTest("TODO: Enable after Kaggle model is public")
+ model = CLIP.from_preset("clip-vit-base-patch16")
+ processed_image = np.ones(shape=[1, 224, 224, 3])
+ processed_text = np.ones(shape=[3, 77])
+ attention_mask = np.ones(shape=[3, 77])
+ image_logits, text_logits = model(
+ processed_image, processed_text, attention_mask
+ )
+
+ @pytest.mark.large
+ def test_image_encoder_golden_values(self):
+ model = CLIP()
+ model.load_weights(MODEL_PATH)
+ processed_image = np.ones(shape=[1, 224, 224, 3])
+ processed_text = np.ones(shape=[3, 77])
+ attention_mask = np.ones(shape=[3, 77])
+ model(processed_image, processed_text, attention_mask)
+ self.assertAllClose(
+ model.image_embeddings[:, :5],
+ [[0.023215, 0.026526, 0.008914, -0.091689, 0.021791]],
+ )
+
+ @pytest.mark.large
+ def test_text_encoder_golden_values(self):
+ model = CLIP()
+ processed_image = np.ones(shape=[1, 224, 224, 3])
+ processed_text = np.ones(shape=[3, 77])
+ attention_mask = np.ones(shape=[3, 77])
+ model(processed_image, processed_text, attention_mask)
+ print(model.text_embeddings)
+ self.assertAllClose(
+ model.text_embeddings[0, :3],
+ [0.007531, -0.038361, -0.035686],
+ )
+
+ @pytest.mark.large # Saving is slow, so mark these large.
+ def test_saved_model(self):
+ model = CLIP()
+ processed_image = np.ones(shape=[1, 224, 224, 3])
+ processed_text = np.ones(shape=[3, 77])
+ attention_mask = np.ones(shape=[3, 77])
+ model_output, _ = model(processed_image, processed_text, attention_mask)
+ save_path = os.path.join(self.get_temp_dir(), "model.keras")
+ if keras_3():
+ model.save(save_path)
+ else:
+ model.save(save_path, save_format="keras_v3")
+ restored_model = keras.models.load_model(save_path)
+
+ # Check we got the real object back.
+ self.assertIsInstance(restored_model, CLIP)
+ # Check that output matches.
+ restored_output, _ = restored_model(
+ processed_image, processed_text, attention_mask
+ )
+ self.assertAllClose(model_output, restored_output)
diff --git a/keras_cv/models/feature_extractor/clip/clip_presets.py b/keras_cv/models/feature_extractor/clip/clip_presets.py
new file mode 100644
index 0000000000..656c9ad8ed
--- /dev/null
+++ b/keras_cv/models/feature_extractor/clip/clip_presets.py
@@ -0,0 +1,81 @@
+# Copyright 2023 The KerasCV Authors
+#
+# Licensed under the Apache License, Version 2.0 (the "License");
+# you may not use this file except in compliance with the License.
+# You may obtain a copy of the License at
+#
+# https://www.apache.org/licenses/LICENSE-2.0
+#
+# Unless required by applicable law or agreed to in writing, software
+# distributed under the License is distributed on an "AS IS" BASIS,
+# WITHOUT WARRANTIES OR CONDITIONS OF ANY KIND, either express or implied.
+# See the License for the specific language governing permissions and
+# limitations under the License.
+"""CLIP presets."""
+
+clip_presets = {
+ "clip-vit-base-patch16": {
+ "metadata": {
+ "description": (
+ "The model uses a ViT-B/16 Transformer architecture as an "
+ "image encoder and uses a masked self-attention Transformer as "
+ "a text encoder. These encoders are trained to maximize the "
+ "similarity of (image, text) pairs via a contrastive loss. The "
+ "model uses a patch size of 16 and input images of size (224, "
+ "224)"
+ ),
+ "params": 149620737,
+ "official_name": "CLIP",
+ "path": "clip",
+ },
+ "kaggle_handle": "kaggle://keras/clip/keras/clip-vit-base-patch16/4",
+ },
+ "clip-vit-base-patch32": {
+ "metadata": {
+ "description": (
+ "The model uses a ViT-B/32 Transformer architecture as an "
+ "image encoder and uses a masked self-attention Transformer as "
+ "a text encoder. These encoders are trained to maximize the "
+ "similarity of (image, text) pairs via a contrastive loss.The "
+ "model uses a patch size of 32 and input images of size (224, "
+ "224)"
+ ),
+ "params": 151277313,
+ "official_name": "CLIP",
+ "path": "clip",
+ },
+ "kaggle_handle": "kaggle://keras/clip/keras/clip-vit-base-patch32/4",
+ },
+ "clip-vit-large-patch14": {
+ "metadata": {
+ "description": (
+ "The model uses a ViT-L/14 Transformer architecture as an "
+ "image encoder and uses a masked self-attention Transformer as "
+ "a text encoder. These encoders are trained to maximize the "
+ "similarity of (image, text) pairs via a contrastive loss.The "
+ "model uses a patch size of 14 and input images of size (224, "
+ "224)"
+ ),
+ "params": 427616513,
+ "official_name": "CLIP",
+ "path": "clip",
+ },
+ "kaggle_handle": "kaggle://keras/clip/keras/clip-vit-large-patch14/4",
+ },
+ "clip-vit-large-patch14-336": {
+ "metadata": {
+ "description": (
+ "The model uses a ViT-L/14 Transformer architecture as an "
+ "image encoder and uses a masked self-attention Transformer as "
+ "a text encoder. These encoders are trained to maximize the "
+ "similarity of (image, text) pairs via a contrastive loss.The "
+ "model uses a patch size of 14 and input images of size (336, "
+ "336)"
+ ),
+ "params": 427944193,
+ "official_name": "CLIP",
+ "path": "clip",
+ },
+ "kaggle_handle": "kaggle://keras/clip/keras/clip-vit-large-patch14-336/4", # noqa: E501
+ },
+}
diff --git a/keras_cv/models/feature_extractor/clip/clip_processor.py b/keras_cv/models/feature_extractor/clip/clip_processor.py
new file mode 100644
index 0000000000..80e616cc02
--- /dev/null
+++ b/keras_cv/models/feature_extractor/clip/clip_processor.py
@@ -0,0 +1,131 @@
+# Copyright 2023 The KerasCV Authors
+#
+# Licensed under the Apache License, Version 2.0 (the "License");
+# you may not use this file except in compliance with the License.
+# You may obtain a copy of the License at
+#
+# https://www.apache.org/licenses/LICENSE-2.0
+#
+# Unless required by applicable law or agreed to in writing, software
+# distributed under the License is distributed on an "AS IS" BASIS,
+# WITHOUT WARRANTIES OR CONDITIONS OF ANY KIND, either express or implied.
+# See the License for the specific language governing permissions and
+# limitations under the License.
+from keras_nlp.layers import StartEndPacker
+
+from keras_cv.api_export import keras_cv_export
+from keras_cv.backend import keras
+from keras_cv.backend import ops
+from keras_cv.models.feature_extractor.clip.clip_tokenizer import CLIPTokenizer
+
+
+@keras_cv_export("keras_cv.models.feature_extractors.CLIPProcessor")
+class CLIPProcessor:
+ """
+ CLIPProcessor is a utility class that provides functionality for processing
+ images and texts in the context of the CLIP (Contrastive Language-Image
+ Pretraining) model.
+
+ Args:
+ input_resolution (int): The resolution of input images.
+ vocabulary (str): string or dict, maps token to integer ids. If it is a
+ string, it should be the file path to a json file.
+ merges: string or list, contains the merge rule. If it is a string, it
+ should be the file path to merge rules. The merge rule file should
+ have one merge rule per line.
+
+ Methods:
+ process_images(image_path: List[str]): Transforms an image located at
+ the specified path.
+
+ process_texts(texts: Union[str, List[str]], context_length: int = 77):
+ Processes a single text or a list of texts, returning packed token
+ sequences.
+
+ """
+
+ def __init__(self, input_resolution, vocabulary, merges, **kwargs):
+ self.input_resolution = input_resolution
+ self.vocabulary = vocabulary
+ self.merges = merges
+ self.image_transform = self.transform_image
+ self.tokenizer = CLIPTokenizer(
+ vocabulary=self.vocabulary,
+ merges=self.merges,
+ unsplittable_tokens=[""],
+ )
+ self.packer = StartEndPacker(
+ start_value=self.tokenizer.token_to_id("<|startoftext|>"),
+ end_value=self.tokenizer.token_to_id("<|endoftext|>"),
+ pad_value=None,
+ sequence_length=77,
+ return_padding_mask=True,
+ )
+
+ def transform_image(self, image_path):
+ input_resolution = self.input_resolution
+ mean = ops.array([0.48145466, 0.4578275, 0.40821073])
+ std = ops.array([0.26862954, 0.26130258, 0.27577711])
+
+ image = keras.utils.load_img(image_path)
+ image = keras.utils.img_to_array(image)
+ image = (
+ ops.image.resize(
+ image,
+ (input_resolution, input_resolution),
+ interpolation="bicubic",
+ )
+ / 255.0
+ )
+ central_fraction = input_resolution / image.shape[0]
+ width, height = image.shape[0], image.shape[1]
+ left = ops.cast((width - width * central_fraction) / 2, dtype="int32")
+ top = ops.cast((height - height * central_fraction) / 2, dtype="int32")
+ right = ops.cast((width + width * central_fraction) / 2, dtype="int32")
+ bottom = ops.cast(
+ (height + height * central_fraction) / 2, dtype="int32"
+ )
+
+ image = ops.slice(
+ image, [left, top, 0], [right - left, bottom - top, 3]
+ )
+
+ image = (image - mean) / std
+ return image
+
+ def process_images(self, images):
+ if isinstance(images, str):
+ images = [images]
+
+ def process_image(image):
+ if isinstance(image, str):
+ return self.image_transform(image)
+
+ processed_images = list(map(process_image, images))
+ processed_images = ops.stack(processed_images)
+ return processed_images
+
+ def process_texts(self, texts, context_length: int = 77):
+ if isinstance(texts, str):
+ texts = [texts]
+
+ def pack_tokens(text):
+ return self.packer(
+ self.tokenizer(text),
+ sequence_length=context_length,
+ add_start_value=True,
+ add_end_value=True,
+ )
+
+ return pack_tokens(texts)
+
+ def get_config(self):
+ config = super().get_config()
+ config.update(
+ {
+ "input_resolution": self.input_resolution,
+ "vocabulary": self.vocabulary,
+ "merges": self.merges,
+ }
+ )
+ return config
diff --git a/keras_cv/models/feature_extractor/clip/clip_text_model.py b/keras_cv/models/feature_extractor/clip/clip_text_model.py
new file mode 100644
index 0000000000..5fc92990d2
--- /dev/null
+++ b/keras_cv/models/feature_extractor/clip/clip_text_model.py
@@ -0,0 +1,118 @@
+# Copyright 2023 The KerasCV Authors
+#
+# Licensed under the Apache License, Version 2.0 (the "License");
+# you may not use this file except in compliance with the License.
+# You may obtain a copy of the License at
+#
+# https://www.apache.org/licenses/LICENSE-2.0
+#
+# Unless required by applicable law or agreed to in writing, software
+# distributed under the License is distributed on an "AS IS" BASIS,
+# WITHOUT WARRANTIES OR CONDITIONS OF ANY KIND, either express or implied.
+# See the License for the specific language governing permissions and
+# limitations under the License.
+from keras_cv.backend import keras
+from keras_cv.backend import ops
+from keras_cv.models.feature_extractor.clip.clip_encoder import CLIPEncoder
+
+
+class CLIPTextEncoder(keras.Model):
+ def __init__(
+ self,
+ transformer_width,
+ transformer_layers,
+ transformer_heads,
+ vocab_size,
+ embed_dim,
+ context_length,
+ **kwargs,
+ ):
+ super().__init__(
+ **kwargs,
+ )
+ self.transformer_width = transformer_width
+ self.transformer_layers = transformer_layers
+ self.transformer_heads = transformer_heads
+ self.vocab_size = vocab_size
+ self.embed_dim = embed_dim
+ self.context_length = context_length
+ self.token_embedding = keras.layers.Embedding(
+ vocab_size,
+ transformer_width,
+ name="token_embedding",
+ )
+ self.positional_embedding = keras.layers.Embedding(
+ self.context_length,
+ transformer_width,
+ name="positional_embedding",
+ )
+
+ self.encoder = CLIPEncoder(
+ width=transformer_width,
+ num_layers=transformer_layers,
+ heads=transformer_heads,
+ name="clip_encoder",
+ )
+ self.ln_final = keras.layers.LayerNormalization(name="ln_final")
+
+ self.text_projector = keras.layers.Dense(
+ embed_dim, name="text_projector", use_bias=False
+ )
+
+ def build(self, input_shape):
+ super().build(input_shape)
+ self.token_embedding.build(input_shape)
+ self.positional_embedding.build([1, self.context_length])
+ self.encoder.build(None)
+ self.ln_final.build([None, None, self.transformer_width])
+ self.text_projector.build([None, None, self.transformer_width])
+
+ def call(self, inputs, attention_mask=None):
+ token_embedding = self.token_embedding(inputs)
+ position_ids = ops.expand_dims(
+ ops.arange(self.context_length, dtype="int32"), 0
+ )
+ position_embedding = self.positional_embedding(position_ids)
+ position_embedding = ops.tile(
+ position_embedding, repeats=(inputs.shape[0], 1, 1)
+ )
+ causal_attention_mask = ops.ones(
+ (self.context_length, self.context_length)
+ )
+ # Zero out the lower diagonal
+ causal_attention_mask = ops.triu(causal_attention_mask)
+ causal_attention_mask = ops.cast(causal_attention_mask, "float32")
+ attention_mask = ops.cast(attention_mask, dtype="float32")
+ expanded_mask = ops.tile(
+ attention_mask[:, None, None, :], (1, 1, self.context_length, 1)
+ )
+ expanded_mask = (1.0 - expanded_mask) * (-1e8)
+ encoded_output = self.encoder(
+ token_embedding + position_embedding,
+ causal_attention_mask=causal_attention_mask,
+ attention_mask=expanded_mask,
+ )
+ layer_norm = self.ln_final(encoded_output)
+ indices = ops.expand_dims(
+ ops.cast(ops.argmax(inputs, axis=-1), "int32"), axis=-1
+ )
+ selected_features = ops.take_along_axis(
+ layer_norm, indices[:, :, None], axis=1
+ )
+ text_features = self.text_projector(selected_features)
+ output = ops.squeeze(text_features, axis=1)
+ return output
+
+ def get_config(self):
+ config = super().get_config()
+ config.update(
+ {
+ "transformer_width": self.transformer_width,
+ "transformer_layers": self.transformer_layers,
+ "transformer_heads": self.transformer_heads,
+ "vocab_size": self.vocab_size,
+ "embed_dim": self.embed_dim,
+ "context_length": self.context_length,
+ }
+ )
+ return config
diff --git a/keras_cv/models/feature_extractor/clip/clip_tokenizer.py b/keras_cv/models/feature_extractor/clip/clip_tokenizer.py
new file mode 100644
index 0000000000..66b4d7cef6
--- /dev/null
+++ b/keras_cv/models/feature_extractor/clip/clip_tokenizer.py
@@ -0,0 +1,186 @@
+# Copyright 2023 The KerasCV Authors
+#
+# Licensed under the Apache License, Version 2.0 (the "License");
+# you may not use this file except in compliance with the License.
+# You may obtain a copy of the License at
+#
+# https://www.apache.org/licenses/LICENSE-2.0
+#
+# Unless required by applicable law or agreed to in writing, software
+# distributed under the License is distributed on an "AS IS" BASIS,
+# WITHOUT WARRANTIES OR CONDITIONS OF ANY KIND, either express or implied.
+# See the License for the specific language governing permissions and
+# limitations under the License.
+import regex as re
+import tensorflow as tf
+import tensorflow_text as tf_text
+
+try:
+ import keras_nlp
+ from keras_nlp.tokenizers import BytePairTokenizer
+except ImportError:
+ keras_nlp = None
+
+# As python and TF handles special spaces differently, we need to
+# manually handle special spaces during string split.
+SPECIAL_WHITESPACES = r"\x{a0}\x{2009}\x{202f}\x{3000}"
+SPLIT_PATTERN_1 = (
+ r"'s|'t|'re|'ve|'m|'ll|'d"
+ + r"|[\s{special_spaces}]+[\n\r\t\f६{special_spaces}]| ?\p{L}+|"
+ + r" ?[\p{N}]+| ?[^\s\p{L}\p{N}{special_spaces}]+"
+)
+SPLIT_PATTERN_1 = SPLIT_PATTERN_1.replace(
+ "{special_spaces}", SPECIAL_WHITESPACES
+)
+SPLIT_PATTERN_2 = rf"""[\s६{SPECIAL_WHITESPACES}]$"""
+
+
+def split_strings_for_bpe(inputs, unsplittable_tokens=None):
+ # We need to recreate the exact behavior of token presplitting in the
+ # original gpt2 tokenizer which uses a lookahead. As re2 does not
+ # support lookahead match, we are using an alternative insert a special
+ # token "६" before leading space of non-space characters and after the
+ # trailing space, e.g., " keras" will be "६ keras".
+ inputs = tf.strings.regex_replace(
+ inputs, rf"( )([^\s{SPECIAL_WHITESPACES}])", r"६\1\2"
+ )
+ inputs = tf.strings.regex_replace(
+ inputs, rf"(\s{SPECIAL_WHITESPACES})$", r"\1६"
+ )
+ inputs = tf.strings.regex_replace(inputs, r"\s", "")
+ if unsplittable_tokens:
+ alts = create_alts_for_unsplittable_tokens(unsplittable_tokens)
+ for token, alt in zip(unsplittable_tokens, alts):
+ escaped_token = re.escape(token)
+ inputs = tf_text.regex_split(inputs, escaped_token, escaped_token)
+ inputs = tf.strings.regex_replace(inputs, escaped_token, alt)
+ raw_tokens = tf_text.regex_split(inputs, SPLIT_PATTERN_1, SPLIT_PATTERN_1)
+ # Second pass splits out the last whilespace char or "६".
+ raw_tokens = tf_text.regex_split(
+ raw_tokens, SPLIT_PATTERN_2, SPLIT_PATTERN_2
+ )
+ if unsplittable_tokens:
+ # Replace special tokens alternate with originals.
+ for token, alt in zip(unsplittable_tokens, alts):
+ escaped_alt = re.escape(alt)
+ raw_tokens = tf.strings.regex_replace(
+ raw_tokens, escaped_alt, token
+ )
+
+ # Add '' to the end of each token
+ tokens_with_end_tag = tf.strings.regex_replace(
+ raw_tokens, r"(\p{L}+)", r"\1"
+ )
+
+ while tokens_with_end_tag.shape.rank > 2:
+ tokens_with_end_tag = tokens_with_end_tag.merge_dims(1, 2)
+
+ return remove_strings_from_inputs(tokens_with_end_tag, "६")
+
+
+def create_alts_for_unsplittable_tokens(unsplittable_tokens):
+ # Create alternates for all special tokens that will be not split during
+ # tokenization.
+ alts = []
+ prefix = "Ĵ"
+ # Trim out splitters.
+ replace_pattern = r"'|\s+|[^\p{L}\p{N}]+"
+ for token in unsplittable_tokens:
+ token = re.sub(replace_pattern, "", token)
+ alts.append(prefix + token)
+ return alts
+
+
+def remove_strings_from_inputs(tensor, string_to_remove):
+ """Remove certain strings from input tensor."""
+ non_empty_mask = tensor != string_to_remove
+ flatten_indexes = tf.where(non_empty_mask)
+ flatten_result = tf.gather_nd(tensor, flatten_indexes)
+ row_lengths = tf.reduce_sum(tf.cast(non_empty_mask, "int64"), axis=1)
+ result = tf.RaggedTensor.from_row_lengths(
+ values=flatten_result,
+ row_lengths=row_lengths,
+ )
+ return result
+
+
+class CLIPTokenizer(BytePairTokenizer):
+ def __init__(self, **kwargs):
+ super().__init__(**kwargs)
+ if keras_nlp is None:
+ raise ValueError(
+ "ClipTokenizer requires keras-nlp. Please install "
+ "using pip `pip install -U keras-nlp && pip install -U keras`"
+ )
+
+ def _bpe_merge_and_update_cache(self, tokens):
+ """Process unseen tokens and add to cache."""
+ words = self._transform_bytes(tokens)
+ tokenized_words = self._bpe_merge(words)
+
+ # For each word, join all its token by a whitespace,
+ # e.g., ["dragon", "fly"] => "dragon fly" for hash purpose.
+ tokenized_words = tf.strings.reduce_join(
+ tokenized_words,
+ axis=1,
+ )
+ self.cache.insert(tokens, tokenized_words)
+
+ def tokenize(self, inputs):
+ self._check_vocabulary()
+ if not isinstance(inputs, (tf.Tensor, tf.RaggedTensor)):
+ inputs = tf.convert_to_tensor(inputs)
+
+ if self.add_prefix_space:
+ inputs = tf.strings.join([" ", inputs])
+
+ scalar_input = inputs.shape.rank == 0
+ if scalar_input:
+ inputs = tf.expand_dims(inputs, 0)
+
+ raw_tokens = split_strings_for_bpe(inputs, self.unsplittable_tokens)
+ token_row_splits = raw_tokens.row_splits
+ flat_tokens = raw_tokens.flat_values
+ # Check cache.
+ cache_lookup = self.cache.lookup(flat_tokens)
+ cache_mask = cache_lookup == ""
+
+ has_unseen_words = tf.math.reduce_any(
+ (cache_lookup == "") & (flat_tokens != "")
+ )
+
+ def process_unseen_tokens():
+ unseen_tokens = tf.boolean_mask(flat_tokens, cache_mask)
+ self._bpe_merge_and_update_cache(unseen_tokens)
+ return self.cache.lookup(flat_tokens)
+
+ # If `has_unseen_words == True`, it means not all tokens are in cache,
+ # we will process the unseen tokens. Otherwise return the cache lookup.
+ tokenized_words = tf.cond(
+ has_unseen_words,
+ process_unseen_tokens,
+ lambda: cache_lookup,
+ )
+ tokens = tf.strings.split(tokenized_words, sep=" ")
+ if self.compute_dtype != tf.string:
+ # Encode merged tokens.
+ tokens = self.token_to_id_map.lookup(tokens)
+
+ # Unflatten to match input.
+ tokens = tf.RaggedTensor.from_row_splits(
+ tokens.flat_values,
+ tf.gather(tokens.row_splits, token_row_splits),
+ )
+
+ # Convert to a dense output if `sequence_length` is set.
+ if self.sequence_length:
+ output_shape = tokens.shape.as_list()
+ output_shape[-1] = self.sequence_length
+ tokens = tokens.to_tensor(shape=output_shape)
+
+ # Convert to a dense output if input in scalar
+ if scalar_input:
+ tokens = tf.squeeze(tokens, 0)
+ tf.ensure_shape(tokens, shape=[self.sequence_length])
+
+ return tokens
diff --git a/keras_cv/tools/checkpoint_conversion/clip_weights_conversion.ipynb b/keras_cv/tools/checkpoint_conversion/clip_weights_conversion.ipynb
new file mode 100644
index 0000000000..ff3bb4c991
--- /dev/null
+++ b/keras_cv/tools/checkpoint_conversion/clip_weights_conversion.ipynb
@@ -0,0 +1,3910 @@
+{
+ "cells": [
+ {
+ "cell_type": "markdown",
+ "metadata": {
+ "id": "0DhV6hzOMY0W"
+ },
+ "source": [
+ "# Setup"
+ ]
+ },
+ {
+ "cell_type": "code",
+ "execution_count": null,
+ "metadata": {
+ "colab": {
+ "base_uri": "https://localhost:8080/"
+ },
+ "id": "cRzYR-oFgxt1",
+ "outputId": "80b8db20-da09-43bd-9b70-fad93b1e1ca1"
+ },
+ "outputs": [
+ {
+ "name": "stdout",
+ "output_type": "stream",
+ "text": [
+ " Installing build dependencies ... \u001b[?25l\u001b[?25hdone\n",
+ " Getting requirements to build wheel ... \u001b[?25l\u001b[?25hdone\n",
+ " Preparing metadata (pyproject.toml) ... \u001b[?25l\u001b[?25hdone\n",
+ "\u001b[2K \u001b[90m━━━━━━━━━━━━━━━━━━━━━━━━━━━━━━━━━━━━━━━━\u001b[0m \u001b[32m950.8/950.8 kB\u001b[0m \u001b[31m6.0 MB/s\u001b[0m eta \u001b[36m0:00:00\u001b[0m\n",
+ "\u001b[?25h Building wheel for keras-cv (pyproject.toml) ... \u001b[?25l\u001b[?25hdone\n",
+ "\u001b[2K \u001b[90m━━━━━━━━━━━━━━━━━━━━━━━━━━━━━━━━━━━━━━━━\u001b[0m \u001b[32m465.2/465.2 kB\u001b[0m \u001b[31m3.8 MB/s\u001b[0m eta \u001b[36m0:00:00\u001b[0m\n",
+ "\u001b[2K \u001b[90m━━━━━━━━━━━━━━━━━━━━━━━━━━━━━━━━━━━━━━━━\u001b[0m \u001b[32m5.2/5.2 MB\u001b[0m \u001b[31m36.1 MB/s\u001b[0m eta \u001b[36m0:00:00\u001b[0m\n",
+ "\u001b[2K \u001b[90m━━━━━━━━━━━━━━━━━━━━━━━━━━━━━━━━━━━━━━━━\u001b[0m \u001b[32m1.0/1.0 MB\u001b[0m \u001b[31m2.8 MB/s\u001b[0m eta \u001b[36m0:00:00\u001b[0m\n",
+ "\u001b[?25h\u001b[31mERROR: pip's dependency resolver does not currently take into account all the packages that are installed. This behaviour is the source of the following dependency conflicts.\n",
+ "tensorflow 2.15.0 requires keras<2.16,>=2.15.0, but you have keras 3.0.2 which is incompatible.\u001b[0m\u001b[31m\n",
+ "\u001b[0m"
+ ]
+ }
+ ],
+ "source": [
+ "!pip install -q git+https://github.com/divyashreepathihalli/keras-cv.git@CLIP_refactor\n",
+ "!pip install -q keras-nlp\n",
+ "!pip install -q tf-keras\n",
+ "!pip install -q tensorflow-text\n",
+ "!pip install -q keras==3.0.2"
+ ]
+ },
+ {
+ "cell_type": "code",
+ "execution_count": null,
+ "metadata": {
+ "colab": {
+ "base_uri": "https://localhost:8080/"
+ },
+ "id": "nuFgha2jTshi",
+ "outputId": "63d4160e-42b3-4f6b-e672-ba30c9402d25"
+ },
+ "outputs": [
+ {
+ "name": "stdout",
+ "output_type": "stream",
+ "text": [
+ "--2024-02-21 20:54:06-- https://i.imgur.com/8H7XCH0.jpg\n",
+ "Resolving i.imgur.com (i.imgur.com)... 146.75.76.193\n",
+ "Connecting to i.imgur.com (i.imgur.com)|146.75.76.193|:443... connected.\n",
+ "HTTP request sent, awaiting response... 200 OK\n",
+ "Length: 44544 (44K) [image/jpeg]\n",
+ "Saving to: ‘cat.jpg’\n",
+ "\n",
+ "\rcat.jpg 0%[ ] 0 --.-KB/s \rcat.jpg 100%[===================>] 43.50K --.-KB/s in 0.01s \n",
+ "\n",
+ "2024-02-21 20:54:06 (4.16 MB/s) - ‘cat.jpg’ saved [44544/44544]\n",
+ "\n",
+ "--2024-02-21 20:54:06-- http://images.cocodataset.org/val2017/000000039769.jpg\n",
+ "Resolving images.cocodataset.org (images.cocodataset.org)... 52.217.206.137, 16.182.42.89, 54.231.201.177, ...\n",
+ "Connecting to images.cocodataset.org (images.cocodataset.org)|52.217.206.137|:80... connected.\n",
+ "HTTP request sent, awaiting response... 200 OK\n",
+ "Length: 173131 (169K) [image/jpeg]\n",
+ "Saving to: ‘two_cats.jpg’\n",
+ "\n",
+ "two_cats.jpg 100%[===================>] 169.07K --.-KB/s in 0.09s \n",
+ "\n",
+ "2024-02-21 20:54:07 (1.77 MB/s) - ‘two_cats.jpg’ saved [173131/173131]\n",
+ "\n",
+ "--2024-02-21 20:54:07-- https://i.imgur.com/PpgZzP4.jpeg\n",
+ "Resolving i.imgur.com (i.imgur.com)... 146.75.76.193\n",
+ "Connecting to i.imgur.com (i.imgur.com)|146.75.76.193|:443... connected.\n",
+ "HTTP request sent, awaiting response... 200 OK\n",
+ "Length: 1610285 (1.5M) [image/jpeg]\n",
+ "Saving to: ‘mountain.jpg’\n",
+ "\n",
+ "mountain.jpg 100%[===================>] 1.54M --.-KB/s in 0.06s \n",
+ "\n",
+ "2024-02-21 20:54:07 (27.6 MB/s) - ‘mountain.jpg’ saved [1610285/1610285]\n",
+ "\n"
+ ]
+ }
+ ],
+ "source": [
+ "!wget https://i.imgur.com/8H7XCH0.jpg -O cat.jpg\n",
+ "!wget http://images.cocodataset.org/val2017/000000039769.jpg -O two_cats.jpg\n",
+ "!wget https://i.imgur.com/PpgZzP4.jpeg -O mountain.jpg"
+ ]
+ },
+ {
+ "cell_type": "markdown",
+ "metadata": {
+ "id": "mdGT8Em4Mc4b"
+ },
+ "source": [
+ "# Import"
+ ]
+ },
+ {
+ "cell_type": "code",
+ "execution_count": null,
+ "metadata": {
+ "id": "0mtj1abS2cVf"
+ },
+ "outputs": [],
+ "source": [
+ "import os\n",
+ "\n",
+ "os.environ[\"KERAS_BACKEND\"] = \"torch\""
+ ]
+ },
+ {
+ "cell_type": "code",
+ "execution_count": null,
+ "metadata": {
+ "id": "GDvJmQuug4-x"
+ },
+ "outputs": [],
+ "source": [
+ "from keras_cv.models.feature_extractor.clip import CLIPProcessor\n",
+ "import keras\n",
+ "from keras_cv.models import CLIP"
+ ]
+ },
+ {
+ "cell_type": "code",
+ "execution_count": null,
+ "metadata": {
+ "id": "X3kkmK6h_gFH"
+ },
+ "outputs": [],
+ "source": [
+ "# @title Select which model weights you would like to convert\n",
+ "MODEL_CONFIGS = {\n",
+ " \"CLIP_B32\": {\n",
+ " \"embed_dim\": 512,\n",
+ " \"context_length\": 77,\n",
+ " \"vocab_size\": 49408,\n",
+ " \"transformer_width\": 512,\n",
+ " \"transformer_heads\": 8,\n",
+ " \"transformer_layers\": 12,\n",
+ " \"vision_layers\": 12,\n",
+ " \"vision_width\": 768,\n",
+ " \"image_resolution\": 224,\n",
+ " \"vision_patch_size\": 32,\n",
+ " },\n",
+ " \"CLIP_B16\": {\n",
+ " \"embed_dim\": 512,\n",
+ " \"context_length\": 77,\n",
+ " \"vocab_size\": 49408,\n",
+ " \"transformer_width\": 512,\n",
+ " \"transformer_heads\": 8,\n",
+ " \"transformer_layers\": 12,\n",
+ " \"vision_layers\": 12,\n",
+ " \"vision_width\": 768,\n",
+ " \"image_resolution\": 224,\n",
+ " \"vision_patch_size\": 16,\n",
+ " },\n",
+ " \"CLIP_L14\": {\n",
+ " \"embed_dim\": 768,\n",
+ " \"context_length\": 77,\n",
+ " \"vocab_size\": 49408,\n",
+ " \"transformer_width\": 768,\n",
+ " \"transformer_heads\": 12,\n",
+ " \"transformer_layers\": 12,\n",
+ " \"vision_layers\": 24,\n",
+ " \"vision_width\": 1024,\n",
+ " \"image_resolution\": 224,\n",
+ " \"vision_patch_size\": 14,\n",
+ " },\n",
+ " \"CLIP_L14_336\": {\n",
+ " \"embed_dim\": 768,\n",
+ " \"context_length\": 77,\n",
+ " \"vocab_size\": 49408,\n",
+ " \"transformer_width\": 768,\n",
+ " \"transformer_heads\": 12,\n",
+ " \"transformer_layers\": 12,\n",
+ " \"vision_layers\": 24,\n",
+ " \"vision_width\": 1024,\n",
+ " \"image_resolution\": 336,\n",
+ " \"vision_patch_size\": 14,\n",
+ " },\n",
+ "}\n",
+ "model_map_hf = {\n",
+ " \"CLIP_B16\": \"openai/clip-vit-base-patch16\",\n",
+ " \"CLIP_B32\": \"openai/clip-vit-base-patch32\",\n",
+ " \"CLIP_L14\": \"openai/clip-vit-large-patch14\",\n",
+ " \"CLIP_L14_336\": \"openai/clip-vit-large-patch14-336\",\n",
+ "}\n",
+ "config_name = \"CLIP_L14_336\" # @param [\"CLIP_B16\", \"CLIP_B32\", \"CLIP_L14\", \"CLIP_L14_336\"]\n",
+ "config_name_hf = model_map_hf[config_name]"
+ ]
+ },
+ {
+ "cell_type": "markdown",
+ "metadata": {
+ "id": "2l3Ll7dMMd-m"
+ },
+ "source": [
+ "# Keras 3 CLIP"
+ ]
+ },
+ {
+ "cell_type": "code",
+ "execution_count": null,
+ "metadata": {
+ "id": "urhuhwq0Dczo"
+ },
+ "outputs": [],
+ "source": [
+ "embed_dim = MODEL_CONFIGS[config_name][\"embed_dim\"]\n",
+ "context_length = MODEL_CONFIGS[config_name][\"context_length\"]\n",
+ "vocab_size = MODEL_CONFIGS[config_name][\"vocab_size\"]\n",
+ "transformer_width = MODEL_CONFIGS[config_name][\"transformer_width\"]\n",
+ "transformer_heads = MODEL_CONFIGS[config_name][\"transformer_heads\"]\n",
+ "transformer_layers = MODEL_CONFIGS[config_name][\"transformer_layers\"]\n",
+ "vision_layers = MODEL_CONFIGS[config_name][\"vision_layers\"]\n",
+ "vision_width = MODEL_CONFIGS[config_name][\"vision_width\"]\n",
+ "vision_patch_size = MODEL_CONFIGS[config_name][\"vision_patch_size\"]\n",
+ "image_resolution = MODEL_CONFIGS[config_name][\"image_resolution\"]\n",
+ "model = CLIP(\n",
+ " embed_dim,\n",
+ " image_resolution,\n",
+ " vision_layers,\n",
+ " vision_width,\n",
+ " vision_patch_size,\n",
+ " context_length,\n",
+ " vocab_size,\n",
+ " transformer_width,\n",
+ " transformer_heads,\n",
+ " transformer_layers,\n",
+ ")"
+ ]
+ },
+ {
+ "cell_type": "code",
+ "execution_count": null,
+ "metadata": {
+ "colab": {
+ "base_uri": "https://localhost:8080/",
+ "height": 193
+ },
+ "id": "uE6x7gfqa3Ee",
+ "outputId": "f55fc358-04a4-42ce-c397-3f81a238ab1e"
+ },
+ "outputs": [
+ {
+ "data": {
+ "text/html": [
+ "
Model: \"clip\"\n",
+ "
\n"
+ ],
+ "text/plain": [
+ "\u001b[1mModel: \"clip\"\u001b[0m\n"
+ ]
+ },
+ "metadata": {},
+ "output_type": "display_data"
+ },
+ {
+ "data": {
+ "text/html": [
+ "┏━━━━━━━━━━━━━━━━━━━━━━━━━━━━━━━━━━━━┳━━━━━━━━━━━━━━━━━━━━━━━━━━━━━━━┳━━━━━━━━━━━━━┓\n",
+ "┃ Layer (type) ┃ Output Shape ┃ Param # ┃\n",
+ "┡━━━━━━━━━━━━━━━━━━━━━━━━━━━━━━━━━━━━╇━━━━━━━━━━━━━━━━━━━━━━━━━━━━━━━╇━━━━━━━━━━━━━┩\n",
+ "│ image_encoder (CLIPImageEncoder) │ ? │ 0 (unbuilt) │\n",
+ "├────────────────────────────────────┼───────────────────────────────┼─────────────┤\n",
+ "│ text_encoder (CLIPTextEncoder) │ ? │ 0 (unbuilt) │\n",
+ "└────────────────────────────────────┴───────────────────────────────┴─────────────┘\n",
+ "
\n"
+ ],
+ "text/plain": [
+ "┏━━━━━━━━━━━━━━━━━━━━━━━━━━━━━━━━━━━━┳━━━━━━━━━━━━━━━━━━━━━━━━━━━━━━━┳━━━━━━━━━━━━━┓\n",
+ "┃\u001b[1m \u001b[0m\u001b[1mLayer (type) \u001b[0m\u001b[1m \u001b[0m┃\u001b[1m \u001b[0m\u001b[1mOutput Shape \u001b[0m\u001b[1m \u001b[0m┃\u001b[1m \u001b[0m\u001b[1m Param #\u001b[0m\u001b[1m \u001b[0m┃\n",
+ "┡━━━━━━━━━━━━━━━━━━━━━━━━━━━━━━━━━━━━╇━━━━━━━━━━━━━━━━━━━━━━━━━━━━━━━╇━━━━━━━━━━━━━┩\n",
+ "│ image_encoder (\u001b[38;5;33mCLIPImageEncoder\u001b[0m) │ ? │ \u001b[38;5;34m0\u001b[0m (unbuilt) │\n",
+ "├────────────────────────────────────┼───────────────────────────────┼─────────────┤\n",
+ "│ text_encoder (\u001b[38;5;33mCLIPTextEncoder\u001b[0m) │ ? │ \u001b[38;5;34m0\u001b[0m (unbuilt) │\n",
+ "└────────────────────────────────────┴───────────────────────────────┴─────────────┘\n"
+ ]
+ },
+ "metadata": {},
+ "output_type": "display_data"
+ },
+ {
+ "data": {
+ "text/html": [
+ " Total params: 1 (4.00 B)\n",
+ "
\n"
+ ],
+ "text/plain": [
+ "\u001b[1m Total params: \u001b[0m\u001b[38;5;34m1\u001b[0m (4.00 B)\n"
+ ]
+ },
+ "metadata": {},
+ "output_type": "display_data"
+ },
+ {
+ "data": {
+ "text/html": [
+ " Trainable params: 1 (4.00 B)\n",
+ "
\n"
+ ],
+ "text/plain": [
+ "\u001b[1m Trainable params: \u001b[0m\u001b[38;5;34m1\u001b[0m (4.00 B)\n"
+ ]
+ },
+ "metadata": {},
+ "output_type": "display_data"
+ },
+ {
+ "data": {
+ "text/html": [
+ " Non-trainable params: 0 (0.00 B)\n",
+ "
\n"
+ ],
+ "text/plain": [
+ "\u001b[1m Non-trainable params: \u001b[0m\u001b[38;5;34m0\u001b[0m (0.00 B)\n"
+ ]
+ },
+ "metadata": {},
+ "output_type": "display_data"
+ }
+ ],
+ "source": [
+ "model.summary()"
+ ]
+ },
+ {
+ "cell_type": "code",
+ "execution_count": null,
+ "metadata": {
+ "id": "buXKlNfGTenW"
+ },
+ "outputs": [],
+ "source": [
+ "processor = CLIPProcessor(\n",
+ " MODEL_CONFIGS[config_name][\"image_resolution\"], \"vocab.json\", \"merges.txt\"\n",
+ ")\n",
+ "image = processor.process_images([\"two_cats.jpg\"])\n",
+ "text_input = [\"mountains\", \"cat on tortoise\", \"two cats\"]\n",
+ "text, attention_mask = processor.process_texts(text_input)"
+ ]
+ },
+ {
+ "cell_type": "code",
+ "execution_count": null,
+ "metadata": {
+ "id": "BHSpMv0PT5SX"
+ },
+ "outputs": [],
+ "source": [
+ "image_logits, text_logits = model(image, text, attention_mask)"
+ ]
+ },
+ {
+ "cell_type": "code",
+ "execution_count": null,
+ "metadata": {
+ "colab": {
+ "base_uri": "https://localhost:8080/"
+ },
+ "id": "JPn0gACJjKy5",
+ "outputId": "cbc7313a-4ddd-4021-9e84-fa668987849d"
+ },
+ "outputs": [
+ {
+ "data": {
+ "text/plain": [
+ "tensor([[3.7318, 3.7792, 3.7633]], grad_fn=)"
+ ]
+ },
+ "execution_count": 32,
+ "metadata": {},
+ "output_type": "execute_result"
+ }
+ ],
+ "source": [
+ "image_logits"
+ ]
+ },
+ {
+ "cell_type": "code",
+ "execution_count": null,
+ "metadata": {
+ "colab": {
+ "base_uri": "https://localhost:8080/",
+ "height": 193
+ },
+ "id": "GgNBvYCTtmA3",
+ "outputId": "a667a9e5-397e-4299-fdc1-8899621112ad"
+ },
+ "outputs": [
+ {
+ "data": {
+ "text/html": [
+ "Model: \"clip\"\n",
+ "
\n"
+ ],
+ "text/plain": [
+ "\u001b[1mModel: \"clip\"\u001b[0m\n"
+ ]
+ },
+ "metadata": {},
+ "output_type": "display_data"
+ },
+ {
+ "data": {
+ "text/html": [
+ "┏━━━━━━━━━━━━━━━━━━━━━━━━━━━━━━━━━━━━┳━━━━━━━━━━━━━━━━━━━━━━━━━━━━━━━┳━━━━━━━━━━━━━┓\n",
+ "┃ Layer (type) ┃ Output Shape ┃ Param # ┃\n",
+ "┡━━━━━━━━━━━━━━━━━━━━━━━━━━━━━━━━━━━━╇━━━━━━━━━━━━━━━━━━━━━━━━━━━━━━━╇━━━━━━━━━━━━━┩\n",
+ "│ image_encoder (CLIPImageEncoder) │ ? │ 304,293,888 │\n",
+ "├────────────────────────────────────┼───────────────────────────────┼─────────────┤\n",
+ "│ text_encoder (CLIPTextEncoder) │ ? │ 123,650,304 │\n",
+ "└────────────────────────────────────┴───────────────────────────────┴─────────────┘\n",
+ "
\n"
+ ],
+ "text/plain": [
+ "┏━━━━━━━━━━━━━━━━━━━━━━━━━━━━━━━━━━━━┳━━━━━━━━━━━━━━━━━━━━━━━━━━━━━━━┳━━━━━━━━━━━━━┓\n",
+ "┃\u001b[1m \u001b[0m\u001b[1mLayer (type) \u001b[0m\u001b[1m \u001b[0m┃\u001b[1m \u001b[0m\u001b[1mOutput Shape \u001b[0m\u001b[1m \u001b[0m┃\u001b[1m \u001b[0m\u001b[1m Param #\u001b[0m\u001b[1m \u001b[0m┃\n",
+ "┡━━━━━━━━━━━━━━━━━━━━━━━━━━━━━━━━━━━━╇━━━━━━━━━━━━━━━━━━━━━━━━━━━━━━━╇━━━━━━━━━━━━━┩\n",
+ "│ image_encoder (\u001b[38;5;33mCLIPImageEncoder\u001b[0m) │ ? │ \u001b[38;5;34m304,293,888\u001b[0m │\n",
+ "├────────────────────────────────────┼───────────────────────────────┼─────────────┤\n",
+ "│ text_encoder (\u001b[38;5;33mCLIPTextEncoder\u001b[0m) │ ? │ \u001b[38;5;34m123,650,304\u001b[0m │\n",
+ "└────────────────────────────────────┴───────────────────────────────┴─────────────┘\n"
+ ]
+ },
+ "metadata": {},
+ "output_type": "display_data"
+ },
+ {
+ "data": {
+ "text/html": [
+ " Total params: 427,944,193 (1.59 GB)\n",
+ "
\n"
+ ],
+ "text/plain": [
+ "\u001b[1m Total params: \u001b[0m\u001b[38;5;34m427,944,193\u001b[0m (1.59 GB)\n"
+ ]
+ },
+ "metadata": {},
+ "output_type": "display_data"
+ },
+ {
+ "data": {
+ "text/html": [
+ " Trainable params: 427,944,193 (1.59 GB)\n",
+ "
\n"
+ ],
+ "text/plain": [
+ "\u001b[1m Trainable params: \u001b[0m\u001b[38;5;34m427,944,193\u001b[0m (1.59 GB)\n"
+ ]
+ },
+ "metadata": {},
+ "output_type": "display_data"
+ },
+ {
+ "data": {
+ "text/html": [
+ " Non-trainable params: 0 (0.00 B)\n",
+ "
\n"
+ ],
+ "text/plain": [
+ "\u001b[1m Non-trainable params: \u001b[0m\u001b[38;5;34m0\u001b[0m (0.00 B)\n"
+ ]
+ },
+ "metadata": {},
+ "output_type": "display_data"
+ }
+ ],
+ "source": [
+ "model.summary()"
+ ]
+ },
+ {
+ "cell_type": "markdown",
+ "metadata": {
+ "id": "P8DWYq_hVFnz"
+ },
+ "source": [
+ "# HF CLIP"
+ ]
+ },
+ {
+ "cell_type": "code",
+ "execution_count": null,
+ "metadata": {
+ "id": "3W2prd6C0pxe"
+ },
+ "outputs": [],
+ "source": [
+ "from PIL import Image\n",
+ "import requests\n",
+ "\n",
+ "from transformers import CLIPProcessor as CP\n",
+ "from transformers import CLIPModel as CM"
+ ]
+ },
+ {
+ "cell_type": "code",
+ "execution_count": null,
+ "metadata": {
+ "colab": {
+ "base_uri": "https://localhost:8080/",
+ "height": 432,
+ "referenced_widgets": [
+ "46636db47838400cb7407fc2ab0720eb",
+ "718081783f7f411599ba5bac18748697",
+ "effcd9cbd407405cbbffe4e76b19df72",
+ "7d4e0cdb53474d50a0b70a6f2d9a0eba",
+ "b3bf65796de8494e82506f6849316426",
+ "5c1c89c4eafc4dc7888236023a4716fd",
+ "3e48c9a0c27d49829a0637c9c727ed8f",
+ "9b1db90efb8b4ef096c5254494c063db",
+ "c9fdbe12b7934fd8accc09645df0f214",
+ "a5b8a21a005d4ef7b253b782dae3b88d",
+ "002207337b4b47c9a6b8ddda823128ba",
+ "f359ba8ef0cf4841b40acafcd770480c",
+ "0861f873665f4514abfd2b09ad944ab8",
+ "b8924ff1bbb5409c8dc141e65c4cdcdb",
+ "4b62b742aacb45c7a286a5d85a0194da",
+ "dfbe3622fad14e798c9f413a134fd107",
+ "0eda9fca096945a48059cbc2b1c9ffdf",
+ "e2bc0bc25b5044abb8bb1c752935922e",
+ "b1b3b62f8d7545938d1e2d8f8757c589",
+ "2d744d00de6745bda5835f0cd66e3909",
+ "6f708ee77df84a8cb1bff9df119ca7df",
+ "8b766a31754f4445bf2614da1ad45446",
+ "3af19b8b653c4b21a65f7e96dd463aac",
+ "a4eddaf970084d9ba057e7038423be01",
+ "f0af662a1a884fb78c693ccf0d0b6d8e",
+ "9acadb088a75425a8115ffd876e161bf",
+ "ef6fd54de3aa46af868c8c810068d9ad",
+ "af229a4850174254b09f850c67aefe3a",
+ "b007afd6777e4364a57a717088356780",
+ "4609b1b46de5441a9632985833bd0b05",
+ "5c5e2b0d9fa7435a92c95d417ed03956",
+ "b8bd9787d9c640e19798be15e94ede04",
+ "e191179e7e4048b69b47d3b9b550b459",
+ "5c21455d9faa4112ba6f18819f7ef038",
+ "a6bd1a75b94f4809b5d275db402f1751",
+ "10b9c5cf60b04a2c875ffe63adb55fb7",
+ "a6e1fe5e2caf42b2968a19df388daf66",
+ "1978049440924b349939aac789bdf797",
+ "6ac5948711754a6c9ef851f6db861e72",
+ "096f5fba1a1e4fbe82d0411363b8c477",
+ "923748d15c194b93bc71fb1046775903",
+ "c37415464174453b9ce13466ed6ff20c",
+ "15b5253136ec4e7db56e103960f4d3f6",
+ "f0ff7fa2d15f41b4b6fae00cb2936acd",
+ "418143d2ad92458094259dfca0a747cc",
+ "6aa0b130877c40f1ab51435705ee1459",
+ "5c4391af49964b7c8dc9839fe649376d",
+ "a45a2501e43448289e482a5713c5fa91",
+ "2b7b34c0eeec43aea25c723ef11d9263",
+ "a2cd61263e2e41e59d3e32a0bafe149a",
+ "9d1ecd1c6e584b7baae967ecba6eaa10",
+ "cb386abe77244108b8f98de9ad3f1bdd",
+ "77f3821d937b486e8d1b40c0f7c4c7dd",
+ "9551ec31f22a4e5fb3c7b6aa618d7f09",
+ "de2e8bd2816b4b2990a78bdb5091f097",
+ "c06b5a6588eb42189210d1c20ccba87a",
+ "da46a678b1fc40d7b660de63d9202997",
+ "c0e3b6e7e7304dc9877d6800f924d05e",
+ "e7035db245c7430c92ceb5b61c31ba14",
+ "d0d7ebc4ce264a6b8ae5c6ba4e18a9b3",
+ "0c46bf3c0a1840cfba66afef11e16cd2",
+ "2a1f21cd845e44c89197edc86b482b71",
+ "837c2d8dd75342a8bbeb1c5ce899e759",
+ "95649d04b8b144b091bba9e8106a44d6",
+ "081d380b0c52402abfd57337726b1aa3",
+ "5da887b8b4fd4437846c639b3ffb575b",
+ "79020bd42626472a85bf9047d014830f",
+ "1771b7a0f46e41dbaa5720effb6084ac",
+ "4542b8ce91584e42b7b98518726ab009",
+ "2b5e2622c68a46d2b407c7cfeca32ae5",
+ "b2d0b2f0ec7648b89fc19a1dda8462ba",
+ "fa6ed2fba5bf4abdaceefc180e4f9a41",
+ "029f9b9eea5a4bd9a70d29a3c9478cb8",
+ "9d61237ba4944593adbfcffd51aa6889",
+ "fc83fdb519174250ae312080e2918abe",
+ "4ec11a213b0d4fdd8300c0ea5a8f8db7",
+ "49807785ba664c49a6b2395ebe7fbec8",
+ "ec7bc6e82f2042b8b29a6f21e6db1709",
+ "609cc0908e6f4edd95306f72b40afd0c",
+ "d5611bb67e8d49f19e2700652d5309c1",
+ "fed0f8954a6b4e1194c63ccc9fba1238",
+ "174aacf5b59048b6ad27a6dffeb87950",
+ "b1cc5c487a364d3ba8d33e0aa3b2a305",
+ "0d9650ba583e45c18bf7c57cc6c57e4b",
+ "380b18596be246d6bc6fd4412fd20379",
+ "2d6ea61d0fa84510b44fff80ab10e553",
+ "098832b366c6410b824d2c210222dc24",
+ "e7e79c91380c478dabb2b1e7ddca647e"
+ ]
+ },
+ "id": "EntuvOq1MhwU",
+ "outputId": "cbd7cd77-6d8f-4a76-dae0-24530c12eeb6"
+ },
+ "outputs": [
+ {
+ "name": "stderr",
+ "output_type": "stream",
+ "text": [
+ "/usr/local/lib/python3.10/dist-packages/huggingface_hub/utils/_token.py:88: UserWarning: \n",
+ "The secret `HF_TOKEN` does not exist in your Colab secrets.\n",
+ "To authenticate with the Hugging Face Hub, create a token in your settings tab (https://huggingface.co/settings/tokens), set it as secret in your Google Colab and restart your session.\n",
+ "You will be able to reuse this secret in all of your notebooks.\n",
+ "Please note that authentication is recommended but still optional to access public models or datasets.\n",
+ " warnings.warn(\n"
+ ]
+ },
+ {
+ "data": {
+ "application/vnd.jupyter.widget-view+json": {
+ "model_id": "46636db47838400cb7407fc2ab0720eb",
+ "version_major": 2,
+ "version_minor": 0
+ },
+ "text/plain": [
+ "config.json: 0%| | 0.00/4.76k [00:00, ?B/s]"
+ ]
+ },
+ "metadata": {},
+ "output_type": "display_data"
+ },
+ {
+ "data": {
+ "application/vnd.jupyter.widget-view+json": {
+ "model_id": "f359ba8ef0cf4841b40acafcd770480c",
+ "version_major": 2,
+ "version_minor": 0
+ },
+ "text/plain": [
+ "pytorch_model.bin: 0%| | 0.00/1.71G [00:00, ?B/s]"
+ ]
+ },
+ "metadata": {},
+ "output_type": "display_data"
+ },
+ {
+ "name": "stderr",
+ "output_type": "stream",
+ "text": [
+ "/usr/local/lib/python3.10/dist-packages/torch/_utils.py:831: UserWarning: TypedStorage is deprecated. It will be removed in the future and UntypedStorage will be the only storage class. This should only matter to you if you are using storages directly. To access UntypedStorage directly, use tensor.untyped_storage() instead of tensor.storage()\n",
+ " return self.fget.__get__(instance, owner)()\n"
+ ]
+ },
+ {
+ "data": {
+ "application/vnd.jupyter.widget-view+json": {
+ "model_id": "3af19b8b653c4b21a65f7e96dd463aac",
+ "version_major": 2,
+ "version_minor": 0
+ },
+ "text/plain": [
+ "preprocessor_config.json: 0%| | 0.00/316 [00:00, ?B/s]"
+ ]
+ },
+ "metadata": {},
+ "output_type": "display_data"
+ },
+ {
+ "data": {
+ "application/vnd.jupyter.widget-view+json": {
+ "model_id": "5c21455d9faa4112ba6f18819f7ef038",
+ "version_major": 2,
+ "version_minor": 0
+ },
+ "text/plain": [
+ "tokenizer_config.json: 0%| | 0.00/844 [00:00, ?B/s]"
+ ]
+ },
+ "metadata": {},
+ "output_type": "display_data"
+ },
+ {
+ "data": {
+ "application/vnd.jupyter.widget-view+json": {
+ "model_id": "418143d2ad92458094259dfca0a747cc",
+ "version_major": 2,
+ "version_minor": 0
+ },
+ "text/plain": [
+ "vocab.json: 0%| | 0.00/862k [00:00, ?B/s]"
+ ]
+ },
+ "metadata": {},
+ "output_type": "display_data"
+ },
+ {
+ "data": {
+ "application/vnd.jupyter.widget-view+json": {
+ "model_id": "c06b5a6588eb42189210d1c20ccba87a",
+ "version_major": 2,
+ "version_minor": 0
+ },
+ "text/plain": [
+ "merges.txt: 0%| | 0.00/525k [00:00, ?B/s]"
+ ]
+ },
+ "metadata": {},
+ "output_type": "display_data"
+ },
+ {
+ "data": {
+ "application/vnd.jupyter.widget-view+json": {
+ "model_id": "79020bd42626472a85bf9047d014830f",
+ "version_major": 2,
+ "version_minor": 0
+ },
+ "text/plain": [
+ "tokenizer.json: 0%| | 0.00/2.22M [00:00, ?B/s]"
+ ]
+ },
+ "metadata": {},
+ "output_type": "display_data"
+ },
+ {
+ "data": {
+ "application/vnd.jupyter.widget-view+json": {
+ "model_id": "ec7bc6e82f2042b8b29a6f21e6db1709",
+ "version_major": 2,
+ "version_minor": 0
+ },
+ "text/plain": [
+ "special_tokens_map.json: 0%| | 0.00/389 [00:00, ?B/s]"
+ ]
+ },
+ "metadata": {},
+ "output_type": "display_data"
+ }
+ ],
+ "source": [
+ "model_hf = CM.from_pretrained(config_name_hf)\n",
+ "processor_hf = CP.from_pretrained(config_name_hf)"
+ ]
+ },
+ {
+ "cell_type": "code",
+ "execution_count": null,
+ "metadata": {
+ "colab": {
+ "base_uri": "https://localhost:8080/"
+ },
+ "id": "Ep8DRTkv3AwS",
+ "outputId": "6e3e802c-3db6-48ac-e3ab-4f52416449a8"
+ },
+ "outputs": [
+ {
+ "data": {
+ "text/plain": [
+ "tensor([[11.7865, 31.2010, 11.9718]], grad_fn=)"
+ ]
+ },
+ "execution_count": 11,
+ "metadata": {},
+ "output_type": "execute_result"
+ }
+ ],
+ "source": [
+ "import torch\n",
+ "import numpy as np\n",
+ "\n",
+ "photo = {\n",
+ " \"cat\": \"https://i.imgur.com/8H7XCH0.jpg\",\n",
+ " \"two_cats\": \"http://images.cocodataset.org/val2017/000000039769.jpg\",\n",
+ " \"mountain\": \"https://i.imgur.com/PpgZzP4.jpeg\",\n",
+ "}\n",
+ "url = photo[\"cat\"]\n",
+ "image_hf = Image.open(requests.get(url, stream=True).raw)\n",
+ "text_inputs = [\"mountains\", \"cat on tortoise\", \"two dogs\"]\n",
+ "inputs = processor_hf(\n",
+ " text=text_inputs, images=image_hf, return_tensors=\"pt\", padding=True\n",
+ ")\n",
+ "outputs = model_hf(**inputs)\n",
+ "logits_per_image = (\n",
+ " outputs.logits_per_image\n",
+ ") # this is the image-text similarity score\n",
+ "probs = logits_per_image.softmax(\n",
+ " dim=1\n",
+ ") # we can take the softmax to get the label probabilitiesprobs\n",
+ "logits_per_image"
+ ]
+ },
+ {
+ "cell_type": "markdown",
+ "metadata": {
+ "id": "ArkCHlVZVKfM"
+ },
+ "source": [
+ "# Copy weights"
+ ]
+ },
+ {
+ "cell_type": "code",
+ "execution_count": null,
+ "metadata": {
+ "id": "wPa0cVnY3cBC"
+ },
+ "outputs": [],
+ "source": [
+ "# hugging face weights\n",
+ "hf_wts = model_hf.state_dict()"
+ ]
+ },
+ {
+ "cell_type": "markdown",
+ "metadata": {
+ "id": "TUCpKltRG4Gd"
+ },
+ "source": [
+ "##vision encoder"
+ ]
+ },
+ {
+ "cell_type": "code",
+ "execution_count": null,
+ "metadata": {
+ "id": "tn_U02N7U2VN"
+ },
+ "outputs": [],
+ "source": [
+ "model.logit_scale.assign(hf_wts.pop(\"logit_scale\").numpy())\n",
+ "model.get_layer(\"image_encoder\").get_layer(\n",
+ " \"clip_patch_embedding\"\n",
+ ").class_embedding.assign(\n",
+ " hf_wts.pop(\"vision_model.embeddings.class_embedding\").numpy().T\n",
+ ")\n",
+ "model.get_layer(\"image_encoder\").get_layer(\n",
+ " \"clip_patch_embedding\"\n",
+ ").positional_embedding.assign(\n",
+ " hf_wts.pop(\"vision_model.embeddings.position_embedding.weight\").numpy()\n",
+ ")\n",
+ "model.get_layer(\"image_encoder\").get_layer(\n",
+ " \"clip_patch_embedding\"\n",
+ ").conv1.weights[0].assign(\n",
+ " hf_wts.pop(\"vision_model.embeddings.patch_embedding.weight\")\n",
+ " .permute(3, 2, 1, 0)\n",
+ " .numpy()\n",
+ ")\n",
+ "model.get_layer(\"image_encoder\").get_layer(\"ln_1\").weights[0].assign(\n",
+ " hf_wts.pop(\"vision_model.pre_layrnorm.weight\").numpy()\n",
+ ")\n",
+ "model.get_layer(\"image_encoder\").get_layer(\"ln_1\").weights[1].assign(\n",
+ " hf_wts.pop(\"vision_model.pre_layrnorm.bias\").numpy()\n",
+ ")\n",
+ "model.get_layer(\"image_encoder\").get_layer(\"ln_2\").weights[0].assign(\n",
+ " hf_wts.pop(\"vision_model.post_layernorm.weight\").numpy()\n",
+ ")\n",
+ "model.get_layer(\"image_encoder\").get_layer(\"ln_2\").weights[1].assign(\n",
+ " hf_wts.pop(\"vision_model.post_layernorm.bias\").numpy()\n",
+ ")\n",
+ "model.get_layer(\"image_encoder\").get_layer(\"vision_projector\").weights[\n",
+ " 0\n",
+ "].assign(hf_wts.pop(\"visual_projection.weight\").numpy().T)"
+ ]
+ },
+ {
+ "cell_type": "code",
+ "execution_count": null,
+ "metadata": {
+ "id": "YRXC2HZC3FjG"
+ },
+ "outputs": [],
+ "source": [
+ "for i in range(0, MODEL_CONFIGS[config_name][\"vision_layers\"]):\n",
+ " if i == 0:\n",
+ " residual_attention = f\"residual_attention\"\n",
+ " else:\n",
+ " residual_attention = f\"residual_attention_{i}\"\n",
+ "\n",
+ " model.get_layer(\"image_encoder\").get_layer(\"clip_encoder\").resblocks[\n",
+ " i\n",
+ " ].attn.q_proj.weights[0].assign(\n",
+ " hf_wts.pop(f\"vision_model.encoder.layers.{i}.self_attn.q_proj.weight\").T\n",
+ " )\n",
+ " model.get_layer(\"image_encoder\").get_layer(\"clip_encoder\").resblocks[\n",
+ " i\n",
+ " ].attn.q_proj.weights[1].assign(\n",
+ " hf_wts.pop(f\"vision_model.encoder.layers.{i}.self_attn.q_proj.bias\")\n",
+ " )\n",
+ " model.get_layer(\"image_encoder\").get_layer(\"clip_encoder\").resblocks[\n",
+ " i\n",
+ " ].attn.k_proj.weights[0].assign(\n",
+ " hf_wts.pop(f\"vision_model.encoder.layers.{i}.self_attn.k_proj.weight\").T\n",
+ " )\n",
+ " model.get_layer(\"image_encoder\").get_layer(\"clip_encoder\").resblocks[\n",
+ " i\n",
+ " ].attn.k_proj.weights[1].assign(\n",
+ " hf_wts.pop(f\"vision_model.encoder.layers.{i}.self_attn.k_proj.bias\")\n",
+ " )\n",
+ " model.get_layer(\"image_encoder\").get_layer(\"clip_encoder\").resblocks[\n",
+ " i\n",
+ " ].attn.v_proj.weights[0].assign(\n",
+ " hf_wts.pop(f\"vision_model.encoder.layers.{i}.self_attn.v_proj.weight\").T\n",
+ " )\n",
+ " model.get_layer(\"image_encoder\").get_layer(\"clip_encoder\").resblocks[\n",
+ " i\n",
+ " ].attn.v_proj.weights[1].assign(\n",
+ " hf_wts.pop(f\"vision_model.encoder.layers.{i}.self_attn.v_proj.bias\")\n",
+ " )\n",
+ " model.get_layer(\"image_encoder\").get_layer(\"clip_encoder\").resblocks[\n",
+ " i\n",
+ " ].attn.out_proj.weights[1].assign(\n",
+ " hf_wts.pop(\n",
+ " f\"vision_model.encoder.layers.{i}.self_attn.out_proj.bias\"\n",
+ " ).numpy()\n",
+ " )\n",
+ " model.get_layer(\"image_encoder\").get_layer(\"clip_encoder\").resblocks[\n",
+ " i\n",
+ " ].attn.out_proj.weights[0].assign(\n",
+ " hf_wts.pop(f\"vision_model.encoder.layers.{i}.self_attn.out_proj.weight\")\n",
+ " .numpy()\n",
+ " .T\n",
+ " )\n",
+ " model.get_layer(\"image_encoder\").get_layer(\"clip_encoder\").resblocks[\n",
+ " i\n",
+ " ].ln_1.weights[0].assign(\n",
+ " hf_wts.pop(\n",
+ " f\"vision_model.encoder.layers.{i}.layer_norm1.weight\"\n",
+ " ).numpy()\n",
+ " )\n",
+ " model.get_layer(\"image_encoder\").get_layer(\"clip_encoder\").resblocks[\n",
+ " i\n",
+ " ].ln_1.weights[1].assign(\n",
+ " hf_wts.pop(f\"vision_model.encoder.layers.{i}.layer_norm1.bias\").numpy()\n",
+ " )\n",
+ " model.get_layer(\"image_encoder\").get_layer(\"clip_encoder\").resblocks[\n",
+ " i\n",
+ " ].ln_2.weights[0].assign(\n",
+ " hf_wts.pop(\n",
+ " f\"vision_model.encoder.layers.{i}.layer_norm2.weight\"\n",
+ " ).numpy()\n",
+ " )\n",
+ " model.get_layer(\"image_encoder\").get_layer(\"clip_encoder\").resblocks[\n",
+ " i\n",
+ " ].ln_2.weights[1].assign(\n",
+ " hf_wts.pop(f\"vision_model.encoder.layers.{i}.layer_norm2.bias\").numpy()\n",
+ " )\n",
+ " model.get_layer(\"image_encoder\").get_layer(\"clip_encoder\").resblocks[\n",
+ " i\n",
+ " ].mlp_dense_1.weights[0].assign(\n",
+ " hf_wts.pop(f\"vision_model.encoder.layers.{i}.mlp.fc1.weight\").numpy().T\n",
+ " )\n",
+ " model.get_layer(\"image_encoder\").get_layer(\"clip_encoder\").resblocks[\n",
+ " i\n",
+ " ].mlp_dense_1.weights[1].assign(\n",
+ " hf_wts.pop(f\"vision_model.encoder.layers.{i}.mlp.fc1.bias\").numpy()\n",
+ " )\n",
+ " model.get_layer(\"image_encoder\").get_layer(\"clip_encoder\").resblocks[\n",
+ " i\n",
+ " ].mlp_dense_2.weights[0].assign(\n",
+ " hf_wts.pop(f\"vision_model.encoder.layers.{i}.mlp.fc2.weight\").numpy().T\n",
+ " )\n",
+ " model.get_layer(\"image_encoder\").get_layer(\"clip_encoder\").resblocks[\n",
+ " i\n",
+ " ].mlp_dense_2.weights[1].assign(\n",
+ " hf_wts.pop(f\"vision_model.encoder.layers.{i}.mlp.fc2.bias\").numpy()\n",
+ " )"
+ ]
+ },
+ {
+ "cell_type": "markdown",
+ "metadata": {
+ "id": "1RN2aVrYG8T3"
+ },
+ "source": [
+ "## Text encoder"
+ ]
+ },
+ {
+ "cell_type": "code",
+ "execution_count": null,
+ "metadata": {
+ "id": "_1AD7TcbdWEC"
+ },
+ "outputs": [],
+ "source": [
+ "model.get_layer(\"text_encoder\").get_layer(\"text_projector\").weights[0].assign(\n",
+ " hf_wts.pop(\"text_projection.weight\").numpy().T\n",
+ ")\n",
+ "model.get_layer(\"text_encoder\").get_layer(\"token_embedding\").weights[0].assign(\n",
+ " hf_wts.pop(\"text_model.embeddings.token_embedding.weight\").numpy()\n",
+ ")\n",
+ "model.get_layer(\"text_encoder\").get_layer(\"positional_embedding\").weights[\n",
+ " 0\n",
+ "].assign(hf_wts.pop(\"text_model.embeddings.position_embedding.weight\").numpy())\n",
+ "model.get_layer(\"text_encoder\").get_layer(\"ln_final\").weights[0].assign(\n",
+ " hf_wts.pop(\"text_model.final_layer_norm.weight\")\n",
+ ")\n",
+ "model.get_layer(\"text_encoder\").get_layer(\"ln_final\").weights[1].assign(\n",
+ " hf_wts.pop(\"text_model.final_layer_norm.bias\")\n",
+ ")"
+ ]
+ },
+ {
+ "cell_type": "code",
+ "execution_count": null,
+ "metadata": {
+ "id": "IQFquy9R75G8"
+ },
+ "outputs": [],
+ "source": [
+ "for i in range(MODEL_CONFIGS[config_name][\"transformer_layers\"]):\n",
+ " model.get_layer(\"text_encoder\").get_layer(\"clip_encoder\").resblocks[\n",
+ " i\n",
+ " ].attn.k_proj.weights[0].assign(\n",
+ " hf_wts.pop(f\"text_model.encoder.layers.{i}.self_attn.k_proj.weight\").T\n",
+ " )\n",
+ " model.get_layer(\"text_encoder\").get_layer(\"clip_encoder\").resblocks[\n",
+ " i\n",
+ " ].attn.k_proj.weights[1].assign(\n",
+ " hf_wts.pop(f\"text_model.encoder.layers.{i}.self_attn.k_proj.bias\")\n",
+ " )\n",
+ " model.get_layer(\"text_encoder\").get_layer(\"clip_encoder\").resblocks[\n",
+ " i\n",
+ " ].attn.q_proj.weights[0].assign(\n",
+ " hf_wts.pop(f\"text_model.encoder.layers.{i}.self_attn.q_proj.weight\").T\n",
+ " )\n",
+ " model.get_layer(\"text_encoder\").get_layer(\"clip_encoder\").resblocks[\n",
+ " i\n",
+ " ].attn.q_proj.weights[1].assign(\n",
+ " hf_wts.pop(f\"text_model.encoder.layers.{i}.self_attn.q_proj.bias\")\n",
+ " )\n",
+ " model.get_layer(\"text_encoder\").get_layer(\"clip_encoder\").resblocks[\n",
+ " i\n",
+ " ].attn.v_proj.weights[0].assign(\n",
+ " hf_wts.pop(f\"text_model.encoder.layers.{i}.self_attn.v_proj.weight\").T\n",
+ " )\n",
+ " model.get_layer(\"text_encoder\").get_layer(\"clip_encoder\").resblocks[\n",
+ " i\n",
+ " ].attn.v_proj.weights[1].assign(\n",
+ " hf_wts.pop(f\"text_model.encoder.layers.{i}.self_attn.v_proj.bias\")\n",
+ " )\n",
+ " model.get_layer(\"text_encoder\").get_layer(\"clip_encoder\").resblocks[\n",
+ " i\n",
+ " ].attn.out_proj.weights[0].assign(\n",
+ " hf_wts.pop(f\"text_model.encoder.layers.{i}.self_attn.out_proj.weight\").T\n",
+ " )\n",
+ " model.get_layer(\"text_encoder\").get_layer(\"clip_encoder\").resblocks[\n",
+ " i\n",
+ " ].attn.out_proj.weights[1].assign(\n",
+ " hf_wts.pop(f\"text_model.encoder.layers.{i}.self_attn.out_proj.bias\")\n",
+ " )\n",
+ " model.get_layer(\"text_encoder\").get_layer(\"clip_encoder\").resblocks[\n",
+ " i\n",
+ " ].ln_1.weights[0].assign(\n",
+ " hf_wts.pop(f\"text_model.encoder.layers.{i}.layer_norm1.weight\").numpy()\n",
+ " )\n",
+ " model.get_layer(\"text_encoder\").get_layer(\"clip_encoder\").resblocks[\n",
+ " i\n",
+ " ].ln_1.weights[1].assign(\n",
+ " hf_wts.pop(f\"text_model.encoder.layers.{i}.layer_norm1.bias\").numpy()\n",
+ " )\n",
+ " model.get_layer(\"text_encoder\").get_layer(\"clip_encoder\").resblocks[\n",
+ " i\n",
+ " ].ln_2.weights[0].assign(\n",
+ " hf_wts.pop(f\"text_model.encoder.layers.{i}.layer_norm2.weight\").numpy()\n",
+ " )\n",
+ " model.get_layer(\"text_encoder\").get_layer(\"clip_encoder\").resblocks[\n",
+ " i\n",
+ " ].ln_2.weights[1].assign(\n",
+ " hf_wts.pop(f\"text_model.encoder.layers.{i}.layer_norm2.bias\").numpy()\n",
+ " )\n",
+ " model.get_layer(\"text_encoder\").get_layer(\"clip_encoder\").resblocks[\n",
+ " i\n",
+ " ].mlp_dense_1.weights[0].assign(\n",
+ " hf_wts.pop(f\"text_model.encoder.layers.{i}.mlp.fc1.weight\").numpy().T\n",
+ " )\n",
+ " model.get_layer(\"text_encoder\").get_layer(\"clip_encoder\").resblocks[\n",
+ " i\n",
+ " ].mlp_dense_1.weights[1].assign(\n",
+ " hf_wts.pop(f\"text_model.encoder.layers.{i}.mlp.fc1.bias\").numpy()\n",
+ " )\n",
+ " model.get_layer(\"text_encoder\").get_layer(\"clip_encoder\").resblocks[\n",
+ " i\n",
+ " ].mlp_dense_2.weights[0].assign(\n",
+ " hf_wts.pop(f\"text_model.encoder.layers.{i}.mlp.fc2.weight\").numpy().T\n",
+ " )\n",
+ " model.get_layer(\"text_encoder\").get_layer(\"clip_encoder\").resblocks[\n",
+ " i\n",
+ " ].mlp_dense_2.weights[1].assign(\n",
+ " hf_wts.pop(f\"text_model.encoder.layers.{i}.mlp.fc2.bias\").numpy()\n",
+ " )"
+ ]
+ },
+ {
+ "cell_type": "code",
+ "execution_count": null,
+ "metadata": {
+ "colab": {
+ "base_uri": "https://localhost:8080/"
+ },
+ "id": "Bgen7hxCCeZ7",
+ "outputId": "e706ca82-d292-4868-9215-d8c160b3736c"
+ },
+ "outputs": [
+ {
+ "data": {
+ "text/plain": [
+ "odict_keys([])"
+ ]
+ },
+ "execution_count": 17,
+ "metadata": {},
+ "output_type": "execute_result"
+ }
+ ],
+ "source": [
+ "# verify that we copied all weights\n",
+ "hf_wts.keys()"
+ ]
+ },
+ {
+ "cell_type": "markdown",
+ "metadata": {
+ "id": "wlfDdO-mid62"
+ },
+ "source": [
+ "# save weights"
+ ]
+ },
+ {
+ "cell_type": "code",
+ "execution_count": null,
+ "metadata": {
+ "id": "QscCUUZFiqBV"
+ },
+ "outputs": [],
+ "source": [
+ "model.save_weights(\"model.weights.h5\")"
+ ]
+ }
+ ],
+ "metadata": {
+ "accelerator": "GPU",
+ "colab": {
+ "gpuType": "V100",
+ "machine_shape": "hm",
+ "provenance": []
+ },
+ "kernelspec": {
+ "display_name": "Python 3",
+ "name": "python3"
+ },
+ "language_info": {
+ "name": "python"
+ },
+ "widgets": {
+ "application/vnd.jupyter.widget-state+json": {
+ "002207337b4b47c9a6b8ddda823128ba": {
+ "model_module": "@jupyter-widgets/controls",
+ "model_module_version": "1.5.0",
+ "model_name": "DescriptionStyleModel",
+ "state": {
+ "_model_module": "@jupyter-widgets/controls",
+ "_model_module_version": "1.5.0",
+ "_model_name": "DescriptionStyleModel",
+ "_view_count": null,
+ "_view_module": "@jupyter-widgets/base",
+ "_view_module_version": "1.2.0",
+ "_view_name": "StyleView",
+ "description_width": ""
+ }
+ },
+ "029f9b9eea5a4bd9a70d29a3c9478cb8": {
+ "model_module": "@jupyter-widgets/controls",
+ "model_module_version": "1.5.0",
+ "model_name": "DescriptionStyleModel",
+ "state": {
+ "_model_module": "@jupyter-widgets/controls",
+ "_model_module_version": "1.5.0",
+ "_model_name": "DescriptionStyleModel",
+ "_view_count": null,
+ "_view_module": "@jupyter-widgets/base",
+ "_view_module_version": "1.2.0",
+ "_view_name": "StyleView",
+ "description_width": ""
+ }
+ },
+ "081d380b0c52402abfd57337726b1aa3": {
+ "model_module": "@jupyter-widgets/base",
+ "model_module_version": "1.2.0",
+ "model_name": "LayoutModel",
+ "state": {
+ "_model_module": "@jupyter-widgets/base",
+ "_model_module_version": "1.2.0",
+ "_model_name": "LayoutModel",
+ "_view_count": null,
+ "_view_module": "@jupyter-widgets/base",
+ "_view_module_version": "1.2.0",
+ "_view_name": "LayoutView",
+ "align_content": null,
+ "align_items": null,
+ "align_self": null,
+ "border": null,
+ "bottom": null,
+ "display": null,
+ "flex": null,
+ "flex_flow": null,
+ "grid_area": null,
+ "grid_auto_columns": null,
+ "grid_auto_flow": null,
+ "grid_auto_rows": null,
+ "grid_column": null,
+ "grid_gap": null,
+ "grid_row": null,
+ "grid_template_areas": null,
+ "grid_template_columns": null,
+ "grid_template_rows": null,
+ "height": null,
+ "justify_content": null,
+ "justify_items": null,
+ "left": null,
+ "margin": null,
+ "max_height": null,
+ "max_width": null,
+ "min_height": null,
+ "min_width": null,
+ "object_fit": null,
+ "object_position": null,
+ "order": null,
+ "overflow": null,
+ "overflow_x": null,
+ "overflow_y": null,
+ "padding": null,
+ "right": null,
+ "top": null,
+ "visibility": null,
+ "width": null
+ }
+ },
+ "0861f873665f4514abfd2b09ad944ab8": {
+ "model_module": "@jupyter-widgets/controls",
+ "model_module_version": "1.5.0",
+ "model_name": "HTMLModel",
+ "state": {
+ "_dom_classes": [],
+ "_model_module": "@jupyter-widgets/controls",
+ "_model_module_version": "1.5.0",
+ "_model_name": "HTMLModel",
+ "_view_count": null,
+ "_view_module": "@jupyter-widgets/controls",
+ "_view_module_version": "1.5.0",
+ "_view_name": "HTMLView",
+ "description": "",
+ "description_tooltip": null,
+ "layout": "IPY_MODEL_0eda9fca096945a48059cbc2b1c9ffdf",
+ "placeholder": "",
+ "style": "IPY_MODEL_e2bc0bc25b5044abb8bb1c752935922e",
+ "value": "pytorch_model.bin: 100%"
+ }
+ },
+ "096f5fba1a1e4fbe82d0411363b8c477": {
+ "model_module": "@jupyter-widgets/controls",
+ "model_module_version": "1.5.0",
+ "model_name": "DescriptionStyleModel",
+ "state": {
+ "_model_module": "@jupyter-widgets/controls",
+ "_model_module_version": "1.5.0",
+ "_model_name": "DescriptionStyleModel",
+ "_view_count": null,
+ "_view_module": "@jupyter-widgets/base",
+ "_view_module_version": "1.2.0",
+ "_view_name": "StyleView",
+ "description_width": ""
+ }
+ },
+ "098832b366c6410b824d2c210222dc24": {
+ "model_module": "@jupyter-widgets/base",
+ "model_module_version": "1.2.0",
+ "model_name": "LayoutModel",
+ "state": {
+ "_model_module": "@jupyter-widgets/base",
+ "_model_module_version": "1.2.0",
+ "_model_name": "LayoutModel",
+ "_view_count": null,
+ "_view_module": "@jupyter-widgets/base",
+ "_view_module_version": "1.2.0",
+ "_view_name": "LayoutView",
+ "align_content": null,
+ "align_items": null,
+ "align_self": null,
+ "border": null,
+ "bottom": null,
+ "display": null,
+ "flex": null,
+ "flex_flow": null,
+ "grid_area": null,
+ "grid_auto_columns": null,
+ "grid_auto_flow": null,
+ "grid_auto_rows": null,
+ "grid_column": null,
+ "grid_gap": null,
+ "grid_row": null,
+ "grid_template_areas": null,
+ "grid_template_columns": null,
+ "grid_template_rows": null,
+ "height": null,
+ "justify_content": null,
+ "justify_items": null,
+ "left": null,
+ "margin": null,
+ "max_height": null,
+ "max_width": null,
+ "min_height": null,
+ "min_width": null,
+ "object_fit": null,
+ "object_position": null,
+ "order": null,
+ "overflow": null,
+ "overflow_x": null,
+ "overflow_y": null,
+ "padding": null,
+ "right": null,
+ "top": null,
+ "visibility": null,
+ "width": null
+ }
+ },
+ "0c46bf3c0a1840cfba66afef11e16cd2": {
+ "model_module": "@jupyter-widgets/base",
+ "model_module_version": "1.2.0",
+ "model_name": "LayoutModel",
+ "state": {
+ "_model_module": "@jupyter-widgets/base",
+ "_model_module_version": "1.2.0",
+ "_model_name": "LayoutModel",
+ "_view_count": null,
+ "_view_module": "@jupyter-widgets/base",
+ "_view_module_version": "1.2.0",
+ "_view_name": "LayoutView",
+ "align_content": null,
+ "align_items": null,
+ "align_self": null,
+ "border": null,
+ "bottom": null,
+ "display": null,
+ "flex": null,
+ "flex_flow": null,
+ "grid_area": null,
+ "grid_auto_columns": null,
+ "grid_auto_flow": null,
+ "grid_auto_rows": null,
+ "grid_column": null,
+ "grid_gap": null,
+ "grid_row": null,
+ "grid_template_areas": null,
+ "grid_template_columns": null,
+ "grid_template_rows": null,
+ "height": null,
+ "justify_content": null,
+ "justify_items": null,
+ "left": null,
+ "margin": null,
+ "max_height": null,
+ "max_width": null,
+ "min_height": null,
+ "min_width": null,
+ "object_fit": null,
+ "object_position": null,
+ "order": null,
+ "overflow": null,
+ "overflow_x": null,
+ "overflow_y": null,
+ "padding": null,
+ "right": null,
+ "top": null,
+ "visibility": null,
+ "width": null
+ }
+ },
+ "0d9650ba583e45c18bf7c57cc6c57e4b": {
+ "model_module": "@jupyter-widgets/controls",
+ "model_module_version": "1.5.0",
+ "model_name": "DescriptionStyleModel",
+ "state": {
+ "_model_module": "@jupyter-widgets/controls",
+ "_model_module_version": "1.5.0",
+ "_model_name": "DescriptionStyleModel",
+ "_view_count": null,
+ "_view_module": "@jupyter-widgets/base",
+ "_view_module_version": "1.2.0",
+ "_view_name": "StyleView",
+ "description_width": ""
+ }
+ },
+ "0eda9fca096945a48059cbc2b1c9ffdf": {
+ "model_module": "@jupyter-widgets/base",
+ "model_module_version": "1.2.0",
+ "model_name": "LayoutModel",
+ "state": {
+ "_model_module": "@jupyter-widgets/base",
+ "_model_module_version": "1.2.0",
+ "_model_name": "LayoutModel",
+ "_view_count": null,
+ "_view_module": "@jupyter-widgets/base",
+ "_view_module_version": "1.2.0",
+ "_view_name": "LayoutView",
+ "align_content": null,
+ "align_items": null,
+ "align_self": null,
+ "border": null,
+ "bottom": null,
+ "display": null,
+ "flex": null,
+ "flex_flow": null,
+ "grid_area": null,
+ "grid_auto_columns": null,
+ "grid_auto_flow": null,
+ "grid_auto_rows": null,
+ "grid_column": null,
+ "grid_gap": null,
+ "grid_row": null,
+ "grid_template_areas": null,
+ "grid_template_columns": null,
+ "grid_template_rows": null,
+ "height": null,
+ "justify_content": null,
+ "justify_items": null,
+ "left": null,
+ "margin": null,
+ "max_height": null,
+ "max_width": null,
+ "min_height": null,
+ "min_width": null,
+ "object_fit": null,
+ "object_position": null,
+ "order": null,
+ "overflow": null,
+ "overflow_x": null,
+ "overflow_y": null,
+ "padding": null,
+ "right": null,
+ "top": null,
+ "visibility": null,
+ "width": null
+ }
+ },
+ "10b9c5cf60b04a2c875ffe63adb55fb7": {
+ "model_module": "@jupyter-widgets/controls",
+ "model_module_version": "1.5.0",
+ "model_name": "FloatProgressModel",
+ "state": {
+ "_dom_classes": [],
+ "_model_module": "@jupyter-widgets/controls",
+ "_model_module_version": "1.5.0",
+ "_model_name": "FloatProgressModel",
+ "_view_count": null,
+ "_view_module": "@jupyter-widgets/controls",
+ "_view_module_version": "1.5.0",
+ "_view_name": "ProgressView",
+ "bar_style": "success",
+ "description": "",
+ "description_tooltip": null,
+ "layout": "IPY_MODEL_923748d15c194b93bc71fb1046775903",
+ "max": 844,
+ "min": 0,
+ "orientation": "horizontal",
+ "style": "IPY_MODEL_c37415464174453b9ce13466ed6ff20c",
+ "value": 844
+ }
+ },
+ "15b5253136ec4e7db56e103960f4d3f6": {
+ "model_module": "@jupyter-widgets/base",
+ "model_module_version": "1.2.0",
+ "model_name": "LayoutModel",
+ "state": {
+ "_model_module": "@jupyter-widgets/base",
+ "_model_module_version": "1.2.0",
+ "_model_name": "LayoutModel",
+ "_view_count": null,
+ "_view_module": "@jupyter-widgets/base",
+ "_view_module_version": "1.2.0",
+ "_view_name": "LayoutView",
+ "align_content": null,
+ "align_items": null,
+ "align_self": null,
+ "border": null,
+ "bottom": null,
+ "display": null,
+ "flex": null,
+ "flex_flow": null,
+ "grid_area": null,
+ "grid_auto_columns": null,
+ "grid_auto_flow": null,
+ "grid_auto_rows": null,
+ "grid_column": null,
+ "grid_gap": null,
+ "grid_row": null,
+ "grid_template_areas": null,
+ "grid_template_columns": null,
+ "grid_template_rows": null,
+ "height": null,
+ "justify_content": null,
+ "justify_items": null,
+ "left": null,
+ "margin": null,
+ "max_height": null,
+ "max_width": null,
+ "min_height": null,
+ "min_width": null,
+ "object_fit": null,
+ "object_position": null,
+ "order": null,
+ "overflow": null,
+ "overflow_x": null,
+ "overflow_y": null,
+ "padding": null,
+ "right": null,
+ "top": null,
+ "visibility": null,
+ "width": null
+ }
+ },
+ "174aacf5b59048b6ad27a6dffeb87950": {
+ "model_module": "@jupyter-widgets/base",
+ "model_module_version": "1.2.0",
+ "model_name": "LayoutModel",
+ "state": {
+ "_model_module": "@jupyter-widgets/base",
+ "_model_module_version": "1.2.0",
+ "_model_name": "LayoutModel",
+ "_view_count": null,
+ "_view_module": "@jupyter-widgets/base",
+ "_view_module_version": "1.2.0",
+ "_view_name": "LayoutView",
+ "align_content": null,
+ "align_items": null,
+ "align_self": null,
+ "border": null,
+ "bottom": null,
+ "display": null,
+ "flex": null,
+ "flex_flow": null,
+ "grid_area": null,
+ "grid_auto_columns": null,
+ "grid_auto_flow": null,
+ "grid_auto_rows": null,
+ "grid_column": null,
+ "grid_gap": null,
+ "grid_row": null,
+ "grid_template_areas": null,
+ "grid_template_columns": null,
+ "grid_template_rows": null,
+ "height": null,
+ "justify_content": null,
+ "justify_items": null,
+ "left": null,
+ "margin": null,
+ "max_height": null,
+ "max_width": null,
+ "min_height": null,
+ "min_width": null,
+ "object_fit": null,
+ "object_position": null,
+ "order": null,
+ "overflow": null,
+ "overflow_x": null,
+ "overflow_y": null,
+ "padding": null,
+ "right": null,
+ "top": null,
+ "visibility": null,
+ "width": null
+ }
+ },
+ "1771b7a0f46e41dbaa5720effb6084ac": {
+ "model_module": "@jupyter-widgets/controls",
+ "model_module_version": "1.5.0",
+ "model_name": "HTMLModel",
+ "state": {
+ "_dom_classes": [],
+ "_model_module": "@jupyter-widgets/controls",
+ "_model_module_version": "1.5.0",
+ "_model_name": "HTMLModel",
+ "_view_count": null,
+ "_view_module": "@jupyter-widgets/controls",
+ "_view_module_version": "1.5.0",
+ "_view_name": "HTMLView",
+ "description": "",
+ "description_tooltip": null,
+ "layout": "IPY_MODEL_fa6ed2fba5bf4abdaceefc180e4f9a41",
+ "placeholder": "",
+ "style": "IPY_MODEL_029f9b9eea5a4bd9a70d29a3c9478cb8",
+ "value": "tokenizer.json: 100%"
+ }
+ },
+ "1978049440924b349939aac789bdf797": {
+ "model_module": "@jupyter-widgets/base",
+ "model_module_version": "1.2.0",
+ "model_name": "LayoutModel",
+ "state": {
+ "_model_module": "@jupyter-widgets/base",
+ "_model_module_version": "1.2.0",
+ "_model_name": "LayoutModel",
+ "_view_count": null,
+ "_view_module": "@jupyter-widgets/base",
+ "_view_module_version": "1.2.0",
+ "_view_name": "LayoutView",
+ "align_content": null,
+ "align_items": null,
+ "align_self": null,
+ "border": null,
+ "bottom": null,
+ "display": null,
+ "flex": null,
+ "flex_flow": null,
+ "grid_area": null,
+ "grid_auto_columns": null,
+ "grid_auto_flow": null,
+ "grid_auto_rows": null,
+ "grid_column": null,
+ "grid_gap": null,
+ "grid_row": null,
+ "grid_template_areas": null,
+ "grid_template_columns": null,
+ "grid_template_rows": null,
+ "height": null,
+ "justify_content": null,
+ "justify_items": null,
+ "left": null,
+ "margin": null,
+ "max_height": null,
+ "max_width": null,
+ "min_height": null,
+ "min_width": null,
+ "object_fit": null,
+ "object_position": null,
+ "order": null,
+ "overflow": null,
+ "overflow_x": null,
+ "overflow_y": null,
+ "padding": null,
+ "right": null,
+ "top": null,
+ "visibility": null,
+ "width": null
+ }
+ },
+ "2a1f21cd845e44c89197edc86b482b71": {
+ "model_module": "@jupyter-widgets/controls",
+ "model_module_version": "1.5.0",
+ "model_name": "DescriptionStyleModel",
+ "state": {
+ "_model_module": "@jupyter-widgets/controls",
+ "_model_module_version": "1.5.0",
+ "_model_name": "DescriptionStyleModel",
+ "_view_count": null,
+ "_view_module": "@jupyter-widgets/base",
+ "_view_module_version": "1.2.0",
+ "_view_name": "StyleView",
+ "description_width": ""
+ }
+ },
+ "2b5e2622c68a46d2b407c7cfeca32ae5": {
+ "model_module": "@jupyter-widgets/controls",
+ "model_module_version": "1.5.0",
+ "model_name": "HTMLModel",
+ "state": {
+ "_dom_classes": [],
+ "_model_module": "@jupyter-widgets/controls",
+ "_model_module_version": "1.5.0",
+ "_model_name": "HTMLModel",
+ "_view_count": null,
+ "_view_module": "@jupyter-widgets/controls",
+ "_view_module_version": "1.5.0",
+ "_view_name": "HTMLView",
+ "description": "",
+ "description_tooltip": null,
+ "layout": "IPY_MODEL_4ec11a213b0d4fdd8300c0ea5a8f8db7",
+ "placeholder": "",
+ "style": "IPY_MODEL_49807785ba664c49a6b2395ebe7fbec8",
+ "value": " 2.22M/2.22M [00:00<00:00, 22.9MB/s]"
+ }
+ },
+ "2b7b34c0eeec43aea25c723ef11d9263": {
+ "model_module": "@jupyter-widgets/base",
+ "model_module_version": "1.2.0",
+ "model_name": "LayoutModel",
+ "state": {
+ "_model_module": "@jupyter-widgets/base",
+ "_model_module_version": "1.2.0",
+ "_model_name": "LayoutModel",
+ "_view_count": null,
+ "_view_module": "@jupyter-widgets/base",
+ "_view_module_version": "1.2.0",
+ "_view_name": "LayoutView",
+ "align_content": null,
+ "align_items": null,
+ "align_self": null,
+ "border": null,
+ "bottom": null,
+ "display": null,
+ "flex": null,
+ "flex_flow": null,
+ "grid_area": null,
+ "grid_auto_columns": null,
+ "grid_auto_flow": null,
+ "grid_auto_rows": null,
+ "grid_column": null,
+ "grid_gap": null,
+ "grid_row": null,
+ "grid_template_areas": null,
+ "grid_template_columns": null,
+ "grid_template_rows": null,
+ "height": null,
+ "justify_content": null,
+ "justify_items": null,
+ "left": null,
+ "margin": null,
+ "max_height": null,
+ "max_width": null,
+ "min_height": null,
+ "min_width": null,
+ "object_fit": null,
+ "object_position": null,
+ "order": null,
+ "overflow": null,
+ "overflow_x": null,
+ "overflow_y": null,
+ "padding": null,
+ "right": null,
+ "top": null,
+ "visibility": null,
+ "width": null
+ }
+ },
+ "2d6ea61d0fa84510b44fff80ab10e553": {
+ "model_module": "@jupyter-widgets/controls",
+ "model_module_version": "1.5.0",
+ "model_name": "ProgressStyleModel",
+ "state": {
+ "_model_module": "@jupyter-widgets/controls",
+ "_model_module_version": "1.5.0",
+ "_model_name": "ProgressStyleModel",
+ "_view_count": null,
+ "_view_module": "@jupyter-widgets/base",
+ "_view_module_version": "1.2.0",
+ "_view_name": "StyleView",
+ "bar_color": null,
+ "description_width": ""
+ }
+ },
+ "2d744d00de6745bda5835f0cd66e3909": {
+ "model_module": "@jupyter-widgets/controls",
+ "model_module_version": "1.5.0",
+ "model_name": "ProgressStyleModel",
+ "state": {
+ "_model_module": "@jupyter-widgets/controls",
+ "_model_module_version": "1.5.0",
+ "_model_name": "ProgressStyleModel",
+ "_view_count": null,
+ "_view_module": "@jupyter-widgets/base",
+ "_view_module_version": "1.2.0",
+ "_view_name": "StyleView",
+ "bar_color": null,
+ "description_width": ""
+ }
+ },
+ "380b18596be246d6bc6fd4412fd20379": {
+ "model_module": "@jupyter-widgets/base",
+ "model_module_version": "1.2.0",
+ "model_name": "LayoutModel",
+ "state": {
+ "_model_module": "@jupyter-widgets/base",
+ "_model_module_version": "1.2.0",
+ "_model_name": "LayoutModel",
+ "_view_count": null,
+ "_view_module": "@jupyter-widgets/base",
+ "_view_module_version": "1.2.0",
+ "_view_name": "LayoutView",
+ "align_content": null,
+ "align_items": null,
+ "align_self": null,
+ "border": null,
+ "bottom": null,
+ "display": null,
+ "flex": null,
+ "flex_flow": null,
+ "grid_area": null,
+ "grid_auto_columns": null,
+ "grid_auto_flow": null,
+ "grid_auto_rows": null,
+ "grid_column": null,
+ "grid_gap": null,
+ "grid_row": null,
+ "grid_template_areas": null,
+ "grid_template_columns": null,
+ "grid_template_rows": null,
+ "height": null,
+ "justify_content": null,
+ "justify_items": null,
+ "left": null,
+ "margin": null,
+ "max_height": null,
+ "max_width": null,
+ "min_height": null,
+ "min_width": null,
+ "object_fit": null,
+ "object_position": null,
+ "order": null,
+ "overflow": null,
+ "overflow_x": null,
+ "overflow_y": null,
+ "padding": null,
+ "right": null,
+ "top": null,
+ "visibility": null,
+ "width": null
+ }
+ },
+ "3af19b8b653c4b21a65f7e96dd463aac": {
+ "model_module": "@jupyter-widgets/controls",
+ "model_module_version": "1.5.0",
+ "model_name": "HBoxModel",
+ "state": {
+ "_dom_classes": [],
+ "_model_module": "@jupyter-widgets/controls",
+ "_model_module_version": "1.5.0",
+ "_model_name": "HBoxModel",
+ "_view_count": null,
+ "_view_module": "@jupyter-widgets/controls",
+ "_view_module_version": "1.5.0",
+ "_view_name": "HBoxView",
+ "box_style": "",
+ "children": [
+ "IPY_MODEL_a4eddaf970084d9ba057e7038423be01",
+ "IPY_MODEL_f0af662a1a884fb78c693ccf0d0b6d8e",
+ "IPY_MODEL_9acadb088a75425a8115ffd876e161bf"
+ ],
+ "layout": "IPY_MODEL_ef6fd54de3aa46af868c8c810068d9ad"
+ }
+ },
+ "3e48c9a0c27d49829a0637c9c727ed8f": {
+ "model_module": "@jupyter-widgets/controls",
+ "model_module_version": "1.5.0",
+ "model_name": "DescriptionStyleModel",
+ "state": {
+ "_model_module": "@jupyter-widgets/controls",
+ "_model_module_version": "1.5.0",
+ "_model_name": "DescriptionStyleModel",
+ "_view_count": null,
+ "_view_module": "@jupyter-widgets/base",
+ "_view_module_version": "1.2.0",
+ "_view_name": "StyleView",
+ "description_width": ""
+ }
+ },
+ "418143d2ad92458094259dfca0a747cc": {
+ "model_module": "@jupyter-widgets/controls",
+ "model_module_version": "1.5.0",
+ "model_name": "HBoxModel",
+ "state": {
+ "_dom_classes": [],
+ "_model_module": "@jupyter-widgets/controls",
+ "_model_module_version": "1.5.0",
+ "_model_name": "HBoxModel",
+ "_view_count": null,
+ "_view_module": "@jupyter-widgets/controls",
+ "_view_module_version": "1.5.0",
+ "_view_name": "HBoxView",
+ "box_style": "",
+ "children": [
+ "IPY_MODEL_6aa0b130877c40f1ab51435705ee1459",
+ "IPY_MODEL_5c4391af49964b7c8dc9839fe649376d",
+ "IPY_MODEL_a45a2501e43448289e482a5713c5fa91"
+ ],
+ "layout": "IPY_MODEL_2b7b34c0eeec43aea25c723ef11d9263"
+ }
+ },
+ "4542b8ce91584e42b7b98518726ab009": {
+ "model_module": "@jupyter-widgets/controls",
+ "model_module_version": "1.5.0",
+ "model_name": "FloatProgressModel",
+ "state": {
+ "_dom_classes": [],
+ "_model_module": "@jupyter-widgets/controls",
+ "_model_module_version": "1.5.0",
+ "_model_name": "FloatProgressModel",
+ "_view_count": null,
+ "_view_module": "@jupyter-widgets/controls",
+ "_view_module_version": "1.5.0",
+ "_view_name": "ProgressView",
+ "bar_style": "success",
+ "description": "",
+ "description_tooltip": null,
+ "layout": "IPY_MODEL_9d61237ba4944593adbfcffd51aa6889",
+ "max": 2224041,
+ "min": 0,
+ "orientation": "horizontal",
+ "style": "IPY_MODEL_fc83fdb519174250ae312080e2918abe",
+ "value": 2224041
+ }
+ },
+ "4609b1b46de5441a9632985833bd0b05": {
+ "model_module": "@jupyter-widgets/base",
+ "model_module_version": "1.2.0",
+ "model_name": "LayoutModel",
+ "state": {
+ "_model_module": "@jupyter-widgets/base",
+ "_model_module_version": "1.2.0",
+ "_model_name": "LayoutModel",
+ "_view_count": null,
+ "_view_module": "@jupyter-widgets/base",
+ "_view_module_version": "1.2.0",
+ "_view_name": "LayoutView",
+ "align_content": null,
+ "align_items": null,
+ "align_self": null,
+ "border": null,
+ "bottom": null,
+ "display": null,
+ "flex": null,
+ "flex_flow": null,
+ "grid_area": null,
+ "grid_auto_columns": null,
+ "grid_auto_flow": null,
+ "grid_auto_rows": null,
+ "grid_column": null,
+ "grid_gap": null,
+ "grid_row": null,
+ "grid_template_areas": null,
+ "grid_template_columns": null,
+ "grid_template_rows": null,
+ "height": null,
+ "justify_content": null,
+ "justify_items": null,
+ "left": null,
+ "margin": null,
+ "max_height": null,
+ "max_width": null,
+ "min_height": null,
+ "min_width": null,
+ "object_fit": null,
+ "object_position": null,
+ "order": null,
+ "overflow": null,
+ "overflow_x": null,
+ "overflow_y": null,
+ "padding": null,
+ "right": null,
+ "top": null,
+ "visibility": null,
+ "width": null
+ }
+ },
+ "46636db47838400cb7407fc2ab0720eb": {
+ "model_module": "@jupyter-widgets/controls",
+ "model_module_version": "1.5.0",
+ "model_name": "HBoxModel",
+ "state": {
+ "_dom_classes": [],
+ "_model_module": "@jupyter-widgets/controls",
+ "_model_module_version": "1.5.0",
+ "_model_name": "HBoxModel",
+ "_view_count": null,
+ "_view_module": "@jupyter-widgets/controls",
+ "_view_module_version": "1.5.0",
+ "_view_name": "HBoxView",
+ "box_style": "",
+ "children": [
+ "IPY_MODEL_718081783f7f411599ba5bac18748697",
+ "IPY_MODEL_effcd9cbd407405cbbffe4e76b19df72",
+ "IPY_MODEL_7d4e0cdb53474d50a0b70a6f2d9a0eba"
+ ],
+ "layout": "IPY_MODEL_b3bf65796de8494e82506f6849316426"
+ }
+ },
+ "49807785ba664c49a6b2395ebe7fbec8": {
+ "model_module": "@jupyter-widgets/controls",
+ "model_module_version": "1.5.0",
+ "model_name": "DescriptionStyleModel",
+ "state": {
+ "_model_module": "@jupyter-widgets/controls",
+ "_model_module_version": "1.5.0",
+ "_model_name": "DescriptionStyleModel",
+ "_view_count": null,
+ "_view_module": "@jupyter-widgets/base",
+ "_view_module_version": "1.2.0",
+ "_view_name": "StyleView",
+ "description_width": ""
+ }
+ },
+ "4b62b742aacb45c7a286a5d85a0194da": {
+ "model_module": "@jupyter-widgets/controls",
+ "model_module_version": "1.5.0",
+ "model_name": "HTMLModel",
+ "state": {
+ "_dom_classes": [],
+ "_model_module": "@jupyter-widgets/controls",
+ "_model_module_version": "1.5.0",
+ "_model_name": "HTMLModel",
+ "_view_count": null,
+ "_view_module": "@jupyter-widgets/controls",
+ "_view_module_version": "1.5.0",
+ "_view_name": "HTMLView",
+ "description": "",
+ "description_tooltip": null,
+ "layout": "IPY_MODEL_6f708ee77df84a8cb1bff9df119ca7df",
+ "placeholder": "",
+ "style": "IPY_MODEL_8b766a31754f4445bf2614da1ad45446",
+ "value": " 1.71G/1.71G [00:14<00:00, 117MB/s]"
+ }
+ },
+ "4ec11a213b0d4fdd8300c0ea5a8f8db7": {
+ "model_module": "@jupyter-widgets/base",
+ "model_module_version": "1.2.0",
+ "model_name": "LayoutModel",
+ "state": {
+ "_model_module": "@jupyter-widgets/base",
+ "_model_module_version": "1.2.0",
+ "_model_name": "LayoutModel",
+ "_view_count": null,
+ "_view_module": "@jupyter-widgets/base",
+ "_view_module_version": "1.2.0",
+ "_view_name": "LayoutView",
+ "align_content": null,
+ "align_items": null,
+ "align_self": null,
+ "border": null,
+ "bottom": null,
+ "display": null,
+ "flex": null,
+ "flex_flow": null,
+ "grid_area": null,
+ "grid_auto_columns": null,
+ "grid_auto_flow": null,
+ "grid_auto_rows": null,
+ "grid_column": null,
+ "grid_gap": null,
+ "grid_row": null,
+ "grid_template_areas": null,
+ "grid_template_columns": null,
+ "grid_template_rows": null,
+ "height": null,
+ "justify_content": null,
+ "justify_items": null,
+ "left": null,
+ "margin": null,
+ "max_height": null,
+ "max_width": null,
+ "min_height": null,
+ "min_width": null,
+ "object_fit": null,
+ "object_position": null,
+ "order": null,
+ "overflow": null,
+ "overflow_x": null,
+ "overflow_y": null,
+ "padding": null,
+ "right": null,
+ "top": null,
+ "visibility": null,
+ "width": null
+ }
+ },
+ "5c1c89c4eafc4dc7888236023a4716fd": {
+ "model_module": "@jupyter-widgets/base",
+ "model_module_version": "1.2.0",
+ "model_name": "LayoutModel",
+ "state": {
+ "_model_module": "@jupyter-widgets/base",
+ "_model_module_version": "1.2.0",
+ "_model_name": "LayoutModel",
+ "_view_count": null,
+ "_view_module": "@jupyter-widgets/base",
+ "_view_module_version": "1.2.0",
+ "_view_name": "LayoutView",
+ "align_content": null,
+ "align_items": null,
+ "align_self": null,
+ "border": null,
+ "bottom": null,
+ "display": null,
+ "flex": null,
+ "flex_flow": null,
+ "grid_area": null,
+ "grid_auto_columns": null,
+ "grid_auto_flow": null,
+ "grid_auto_rows": null,
+ "grid_column": null,
+ "grid_gap": null,
+ "grid_row": null,
+ "grid_template_areas": null,
+ "grid_template_columns": null,
+ "grid_template_rows": null,
+ "height": null,
+ "justify_content": null,
+ "justify_items": null,
+ "left": null,
+ "margin": null,
+ "max_height": null,
+ "max_width": null,
+ "min_height": null,
+ "min_width": null,
+ "object_fit": null,
+ "object_position": null,
+ "order": null,
+ "overflow": null,
+ "overflow_x": null,
+ "overflow_y": null,
+ "padding": null,
+ "right": null,
+ "top": null,
+ "visibility": null,
+ "width": null
+ }
+ },
+ "5c21455d9faa4112ba6f18819f7ef038": {
+ "model_module": "@jupyter-widgets/controls",
+ "model_module_version": "1.5.0",
+ "model_name": "HBoxModel",
+ "state": {
+ "_dom_classes": [],
+ "_model_module": "@jupyter-widgets/controls",
+ "_model_module_version": "1.5.0",
+ "_model_name": "HBoxModel",
+ "_view_count": null,
+ "_view_module": "@jupyter-widgets/controls",
+ "_view_module_version": "1.5.0",
+ "_view_name": "HBoxView",
+ "box_style": "",
+ "children": [
+ "IPY_MODEL_a6bd1a75b94f4809b5d275db402f1751",
+ "IPY_MODEL_10b9c5cf60b04a2c875ffe63adb55fb7",
+ "IPY_MODEL_a6e1fe5e2caf42b2968a19df388daf66"
+ ],
+ "layout": "IPY_MODEL_1978049440924b349939aac789bdf797"
+ }
+ },
+ "5c4391af49964b7c8dc9839fe649376d": {
+ "model_module": "@jupyter-widgets/controls",
+ "model_module_version": "1.5.0",
+ "model_name": "FloatProgressModel",
+ "state": {
+ "_dom_classes": [],
+ "_model_module": "@jupyter-widgets/controls",
+ "_model_module_version": "1.5.0",
+ "_model_name": "FloatProgressModel",
+ "_view_count": null,
+ "_view_module": "@jupyter-widgets/controls",
+ "_view_module_version": "1.5.0",
+ "_view_name": "ProgressView",
+ "bar_style": "success",
+ "description": "",
+ "description_tooltip": null,
+ "layout": "IPY_MODEL_cb386abe77244108b8f98de9ad3f1bdd",
+ "max": 862328,
+ "min": 0,
+ "orientation": "horizontal",
+ "style": "IPY_MODEL_77f3821d937b486e8d1b40c0f7c4c7dd",
+ "value": 862328
+ }
+ },
+ "5c5e2b0d9fa7435a92c95d417ed03956": {
+ "model_module": "@jupyter-widgets/controls",
+ "model_module_version": "1.5.0",
+ "model_name": "ProgressStyleModel",
+ "state": {
+ "_model_module": "@jupyter-widgets/controls",
+ "_model_module_version": "1.5.0",
+ "_model_name": "ProgressStyleModel",
+ "_view_count": null,
+ "_view_module": "@jupyter-widgets/base",
+ "_view_module_version": "1.2.0",
+ "_view_name": "StyleView",
+ "bar_color": null,
+ "description_width": ""
+ }
+ },
+ "5da887b8b4fd4437846c639b3ffb575b": {
+ "model_module": "@jupyter-widgets/controls",
+ "model_module_version": "1.5.0",
+ "model_name": "DescriptionStyleModel",
+ "state": {
+ "_model_module": "@jupyter-widgets/controls",
+ "_model_module_version": "1.5.0",
+ "_model_name": "DescriptionStyleModel",
+ "_view_count": null,
+ "_view_module": "@jupyter-widgets/base",
+ "_view_module_version": "1.2.0",
+ "_view_name": "StyleView",
+ "description_width": ""
+ }
+ },
+ "609cc0908e6f4edd95306f72b40afd0c": {
+ "model_module": "@jupyter-widgets/controls",
+ "model_module_version": "1.5.0",
+ "model_name": "HTMLModel",
+ "state": {
+ "_dom_classes": [],
+ "_model_module": "@jupyter-widgets/controls",
+ "_model_module_version": "1.5.0",
+ "_model_name": "HTMLModel",
+ "_view_count": null,
+ "_view_module": "@jupyter-widgets/controls",
+ "_view_module_version": "1.5.0",
+ "_view_name": "HTMLView",
+ "description": "",
+ "description_tooltip": null,
+ "layout": "IPY_MODEL_b1cc5c487a364d3ba8d33e0aa3b2a305",
+ "placeholder": "",
+ "style": "IPY_MODEL_0d9650ba583e45c18bf7c57cc6c57e4b",
+ "value": "special_tokens_map.json: 100%"
+ }
+ },
+ "6aa0b130877c40f1ab51435705ee1459": {
+ "model_module": "@jupyter-widgets/controls",
+ "model_module_version": "1.5.0",
+ "model_name": "HTMLModel",
+ "state": {
+ "_dom_classes": [],
+ "_model_module": "@jupyter-widgets/controls",
+ "_model_module_version": "1.5.0",
+ "_model_name": "HTMLModel",
+ "_view_count": null,
+ "_view_module": "@jupyter-widgets/controls",
+ "_view_module_version": "1.5.0",
+ "_view_name": "HTMLView",
+ "description": "",
+ "description_tooltip": null,
+ "layout": "IPY_MODEL_a2cd61263e2e41e59d3e32a0bafe149a",
+ "placeholder": "",
+ "style": "IPY_MODEL_9d1ecd1c6e584b7baae967ecba6eaa10",
+ "value": "vocab.json: 100%"
+ }
+ },
+ "6ac5948711754a6c9ef851f6db861e72": {
+ "model_module": "@jupyter-widgets/base",
+ "model_module_version": "1.2.0",
+ "model_name": "LayoutModel",
+ "state": {
+ "_model_module": "@jupyter-widgets/base",
+ "_model_module_version": "1.2.0",
+ "_model_name": "LayoutModel",
+ "_view_count": null,
+ "_view_module": "@jupyter-widgets/base",
+ "_view_module_version": "1.2.0",
+ "_view_name": "LayoutView",
+ "align_content": null,
+ "align_items": null,
+ "align_self": null,
+ "border": null,
+ "bottom": null,
+ "display": null,
+ "flex": null,
+ "flex_flow": null,
+ "grid_area": null,
+ "grid_auto_columns": null,
+ "grid_auto_flow": null,
+ "grid_auto_rows": null,
+ "grid_column": null,
+ "grid_gap": null,
+ "grid_row": null,
+ "grid_template_areas": null,
+ "grid_template_columns": null,
+ "grid_template_rows": null,
+ "height": null,
+ "justify_content": null,
+ "justify_items": null,
+ "left": null,
+ "margin": null,
+ "max_height": null,
+ "max_width": null,
+ "min_height": null,
+ "min_width": null,
+ "object_fit": null,
+ "object_position": null,
+ "order": null,
+ "overflow": null,
+ "overflow_x": null,
+ "overflow_y": null,
+ "padding": null,
+ "right": null,
+ "top": null,
+ "visibility": null,
+ "width": null
+ }
+ },
+ "6f708ee77df84a8cb1bff9df119ca7df": {
+ "model_module": "@jupyter-widgets/base",
+ "model_module_version": "1.2.0",
+ "model_name": "LayoutModel",
+ "state": {
+ "_model_module": "@jupyter-widgets/base",
+ "_model_module_version": "1.2.0",
+ "_model_name": "LayoutModel",
+ "_view_count": null,
+ "_view_module": "@jupyter-widgets/base",
+ "_view_module_version": "1.2.0",
+ "_view_name": "LayoutView",
+ "align_content": null,
+ "align_items": null,
+ "align_self": null,
+ "border": null,
+ "bottom": null,
+ "display": null,
+ "flex": null,
+ "flex_flow": null,
+ "grid_area": null,
+ "grid_auto_columns": null,
+ "grid_auto_flow": null,
+ "grid_auto_rows": null,
+ "grid_column": null,
+ "grid_gap": null,
+ "grid_row": null,
+ "grid_template_areas": null,
+ "grid_template_columns": null,
+ "grid_template_rows": null,
+ "height": null,
+ "justify_content": null,
+ "justify_items": null,
+ "left": null,
+ "margin": null,
+ "max_height": null,
+ "max_width": null,
+ "min_height": null,
+ "min_width": null,
+ "object_fit": null,
+ "object_position": null,
+ "order": null,
+ "overflow": null,
+ "overflow_x": null,
+ "overflow_y": null,
+ "padding": null,
+ "right": null,
+ "top": null,
+ "visibility": null,
+ "width": null
+ }
+ },
+ "718081783f7f411599ba5bac18748697": {
+ "model_module": "@jupyter-widgets/controls",
+ "model_module_version": "1.5.0",
+ "model_name": "HTMLModel",
+ "state": {
+ "_dom_classes": [],
+ "_model_module": "@jupyter-widgets/controls",
+ "_model_module_version": "1.5.0",
+ "_model_name": "HTMLModel",
+ "_view_count": null,
+ "_view_module": "@jupyter-widgets/controls",
+ "_view_module_version": "1.5.0",
+ "_view_name": "HTMLView",
+ "description": "",
+ "description_tooltip": null,
+ "layout": "IPY_MODEL_5c1c89c4eafc4dc7888236023a4716fd",
+ "placeholder": "",
+ "style": "IPY_MODEL_3e48c9a0c27d49829a0637c9c727ed8f",
+ "value": "config.json: 100%"
+ }
+ },
+ "77f3821d937b486e8d1b40c0f7c4c7dd": {
+ "model_module": "@jupyter-widgets/controls",
+ "model_module_version": "1.5.0",
+ "model_name": "ProgressStyleModel",
+ "state": {
+ "_model_module": "@jupyter-widgets/controls",
+ "_model_module_version": "1.5.0",
+ "_model_name": "ProgressStyleModel",
+ "_view_count": null,
+ "_view_module": "@jupyter-widgets/base",
+ "_view_module_version": "1.2.0",
+ "_view_name": "StyleView",
+ "bar_color": null,
+ "description_width": ""
+ }
+ },
+ "79020bd42626472a85bf9047d014830f": {
+ "model_module": "@jupyter-widgets/controls",
+ "model_module_version": "1.5.0",
+ "model_name": "HBoxModel",
+ "state": {
+ "_dom_classes": [],
+ "_model_module": "@jupyter-widgets/controls",
+ "_model_module_version": "1.5.0",
+ "_model_name": "HBoxModel",
+ "_view_count": null,
+ "_view_module": "@jupyter-widgets/controls",
+ "_view_module_version": "1.5.0",
+ "_view_name": "HBoxView",
+ "box_style": "",
+ "children": [
+ "IPY_MODEL_1771b7a0f46e41dbaa5720effb6084ac",
+ "IPY_MODEL_4542b8ce91584e42b7b98518726ab009",
+ "IPY_MODEL_2b5e2622c68a46d2b407c7cfeca32ae5"
+ ],
+ "layout": "IPY_MODEL_b2d0b2f0ec7648b89fc19a1dda8462ba"
+ }
+ },
+ "7d4e0cdb53474d50a0b70a6f2d9a0eba": {
+ "model_module": "@jupyter-widgets/controls",
+ "model_module_version": "1.5.0",
+ "model_name": "HTMLModel",
+ "state": {
+ "_dom_classes": [],
+ "_model_module": "@jupyter-widgets/controls",
+ "_model_module_version": "1.5.0",
+ "_model_name": "HTMLModel",
+ "_view_count": null,
+ "_view_module": "@jupyter-widgets/controls",
+ "_view_module_version": "1.5.0",
+ "_view_name": "HTMLView",
+ "description": "",
+ "description_tooltip": null,
+ "layout": "IPY_MODEL_a5b8a21a005d4ef7b253b782dae3b88d",
+ "placeholder": "",
+ "style": "IPY_MODEL_002207337b4b47c9a6b8ddda823128ba",
+ "value": " 4.76k/4.76k [00:00<00:00, 166kB/s]"
+ }
+ },
+ "837c2d8dd75342a8bbeb1c5ce899e759": {
+ "model_module": "@jupyter-widgets/base",
+ "model_module_version": "1.2.0",
+ "model_name": "LayoutModel",
+ "state": {
+ "_model_module": "@jupyter-widgets/base",
+ "_model_module_version": "1.2.0",
+ "_model_name": "LayoutModel",
+ "_view_count": null,
+ "_view_module": "@jupyter-widgets/base",
+ "_view_module_version": "1.2.0",
+ "_view_name": "LayoutView",
+ "align_content": null,
+ "align_items": null,
+ "align_self": null,
+ "border": null,
+ "bottom": null,
+ "display": null,
+ "flex": null,
+ "flex_flow": null,
+ "grid_area": null,
+ "grid_auto_columns": null,
+ "grid_auto_flow": null,
+ "grid_auto_rows": null,
+ "grid_column": null,
+ "grid_gap": null,
+ "grid_row": null,
+ "grid_template_areas": null,
+ "grid_template_columns": null,
+ "grid_template_rows": null,
+ "height": null,
+ "justify_content": null,
+ "justify_items": null,
+ "left": null,
+ "margin": null,
+ "max_height": null,
+ "max_width": null,
+ "min_height": null,
+ "min_width": null,
+ "object_fit": null,
+ "object_position": null,
+ "order": null,
+ "overflow": null,
+ "overflow_x": null,
+ "overflow_y": null,
+ "padding": null,
+ "right": null,
+ "top": null,
+ "visibility": null,
+ "width": null
+ }
+ },
+ "8b766a31754f4445bf2614da1ad45446": {
+ "model_module": "@jupyter-widgets/controls",
+ "model_module_version": "1.5.0",
+ "model_name": "DescriptionStyleModel",
+ "state": {
+ "_model_module": "@jupyter-widgets/controls",
+ "_model_module_version": "1.5.0",
+ "_model_name": "DescriptionStyleModel",
+ "_view_count": null,
+ "_view_module": "@jupyter-widgets/base",
+ "_view_module_version": "1.2.0",
+ "_view_name": "StyleView",
+ "description_width": ""
+ }
+ },
+ "923748d15c194b93bc71fb1046775903": {
+ "model_module": "@jupyter-widgets/base",
+ "model_module_version": "1.2.0",
+ "model_name": "LayoutModel",
+ "state": {
+ "_model_module": "@jupyter-widgets/base",
+ "_model_module_version": "1.2.0",
+ "_model_name": "LayoutModel",
+ "_view_count": null,
+ "_view_module": "@jupyter-widgets/base",
+ "_view_module_version": "1.2.0",
+ "_view_name": "LayoutView",
+ "align_content": null,
+ "align_items": null,
+ "align_self": null,
+ "border": null,
+ "bottom": null,
+ "display": null,
+ "flex": null,
+ "flex_flow": null,
+ "grid_area": null,
+ "grid_auto_columns": null,
+ "grid_auto_flow": null,
+ "grid_auto_rows": null,
+ "grid_column": null,
+ "grid_gap": null,
+ "grid_row": null,
+ "grid_template_areas": null,
+ "grid_template_columns": null,
+ "grid_template_rows": null,
+ "height": null,
+ "justify_content": null,
+ "justify_items": null,
+ "left": null,
+ "margin": null,
+ "max_height": null,
+ "max_width": null,
+ "min_height": null,
+ "min_width": null,
+ "object_fit": null,
+ "object_position": null,
+ "order": null,
+ "overflow": null,
+ "overflow_x": null,
+ "overflow_y": null,
+ "padding": null,
+ "right": null,
+ "top": null,
+ "visibility": null,
+ "width": null
+ }
+ },
+ "9551ec31f22a4e5fb3c7b6aa618d7f09": {
+ "model_module": "@jupyter-widgets/base",
+ "model_module_version": "1.2.0",
+ "model_name": "LayoutModel",
+ "state": {
+ "_model_module": "@jupyter-widgets/base",
+ "_model_module_version": "1.2.0",
+ "_model_name": "LayoutModel",
+ "_view_count": null,
+ "_view_module": "@jupyter-widgets/base",
+ "_view_module_version": "1.2.0",
+ "_view_name": "LayoutView",
+ "align_content": null,
+ "align_items": null,
+ "align_self": null,
+ "border": null,
+ "bottom": null,
+ "display": null,
+ "flex": null,
+ "flex_flow": null,
+ "grid_area": null,
+ "grid_auto_columns": null,
+ "grid_auto_flow": null,
+ "grid_auto_rows": null,
+ "grid_column": null,
+ "grid_gap": null,
+ "grid_row": null,
+ "grid_template_areas": null,
+ "grid_template_columns": null,
+ "grid_template_rows": null,
+ "height": null,
+ "justify_content": null,
+ "justify_items": null,
+ "left": null,
+ "margin": null,
+ "max_height": null,
+ "max_width": null,
+ "min_height": null,
+ "min_width": null,
+ "object_fit": null,
+ "object_position": null,
+ "order": null,
+ "overflow": null,
+ "overflow_x": null,
+ "overflow_y": null,
+ "padding": null,
+ "right": null,
+ "top": null,
+ "visibility": null,
+ "width": null
+ }
+ },
+ "95649d04b8b144b091bba9e8106a44d6": {
+ "model_module": "@jupyter-widgets/controls",
+ "model_module_version": "1.5.0",
+ "model_name": "ProgressStyleModel",
+ "state": {
+ "_model_module": "@jupyter-widgets/controls",
+ "_model_module_version": "1.5.0",
+ "_model_name": "ProgressStyleModel",
+ "_view_count": null,
+ "_view_module": "@jupyter-widgets/base",
+ "_view_module_version": "1.2.0",
+ "_view_name": "StyleView",
+ "bar_color": null,
+ "description_width": ""
+ }
+ },
+ "9acadb088a75425a8115ffd876e161bf": {
+ "model_module": "@jupyter-widgets/controls",
+ "model_module_version": "1.5.0",
+ "model_name": "HTMLModel",
+ "state": {
+ "_dom_classes": [],
+ "_model_module": "@jupyter-widgets/controls",
+ "_model_module_version": "1.5.0",
+ "_model_name": "HTMLModel",
+ "_view_count": null,
+ "_view_module": "@jupyter-widgets/controls",
+ "_view_module_version": "1.5.0",
+ "_view_name": "HTMLView",
+ "description": "",
+ "description_tooltip": null,
+ "layout": "IPY_MODEL_b8bd9787d9c640e19798be15e94ede04",
+ "placeholder": "",
+ "style": "IPY_MODEL_e191179e7e4048b69b47d3b9b550b459",
+ "value": " 316/316 [00:00<00:00, 19.2kB/s]"
+ }
+ },
+ "9b1db90efb8b4ef096c5254494c063db": {
+ "model_module": "@jupyter-widgets/base",
+ "model_module_version": "1.2.0",
+ "model_name": "LayoutModel",
+ "state": {
+ "_model_module": "@jupyter-widgets/base",
+ "_model_module_version": "1.2.0",
+ "_model_name": "LayoutModel",
+ "_view_count": null,
+ "_view_module": "@jupyter-widgets/base",
+ "_view_module_version": "1.2.0",
+ "_view_name": "LayoutView",
+ "align_content": null,
+ "align_items": null,
+ "align_self": null,
+ "border": null,
+ "bottom": null,
+ "display": null,
+ "flex": null,
+ "flex_flow": null,
+ "grid_area": null,
+ "grid_auto_columns": null,
+ "grid_auto_flow": null,
+ "grid_auto_rows": null,
+ "grid_column": null,
+ "grid_gap": null,
+ "grid_row": null,
+ "grid_template_areas": null,
+ "grid_template_columns": null,
+ "grid_template_rows": null,
+ "height": null,
+ "justify_content": null,
+ "justify_items": null,
+ "left": null,
+ "margin": null,
+ "max_height": null,
+ "max_width": null,
+ "min_height": null,
+ "min_width": null,
+ "object_fit": null,
+ "object_position": null,
+ "order": null,
+ "overflow": null,
+ "overflow_x": null,
+ "overflow_y": null,
+ "padding": null,
+ "right": null,
+ "top": null,
+ "visibility": null,
+ "width": null
+ }
+ },
+ "9d1ecd1c6e584b7baae967ecba6eaa10": {
+ "model_module": "@jupyter-widgets/controls",
+ "model_module_version": "1.5.0",
+ "model_name": "DescriptionStyleModel",
+ "state": {
+ "_model_module": "@jupyter-widgets/controls",
+ "_model_module_version": "1.5.0",
+ "_model_name": "DescriptionStyleModel",
+ "_view_count": null,
+ "_view_module": "@jupyter-widgets/base",
+ "_view_module_version": "1.2.0",
+ "_view_name": "StyleView",
+ "description_width": ""
+ }
+ },
+ "9d61237ba4944593adbfcffd51aa6889": {
+ "model_module": "@jupyter-widgets/base",
+ "model_module_version": "1.2.0",
+ "model_name": "LayoutModel",
+ "state": {
+ "_model_module": "@jupyter-widgets/base",
+ "_model_module_version": "1.2.0",
+ "_model_name": "LayoutModel",
+ "_view_count": null,
+ "_view_module": "@jupyter-widgets/base",
+ "_view_module_version": "1.2.0",
+ "_view_name": "LayoutView",
+ "align_content": null,
+ "align_items": null,
+ "align_self": null,
+ "border": null,
+ "bottom": null,
+ "display": null,
+ "flex": null,
+ "flex_flow": null,
+ "grid_area": null,
+ "grid_auto_columns": null,
+ "grid_auto_flow": null,
+ "grid_auto_rows": null,
+ "grid_column": null,
+ "grid_gap": null,
+ "grid_row": null,
+ "grid_template_areas": null,
+ "grid_template_columns": null,
+ "grid_template_rows": null,
+ "height": null,
+ "justify_content": null,
+ "justify_items": null,
+ "left": null,
+ "margin": null,
+ "max_height": null,
+ "max_width": null,
+ "min_height": null,
+ "min_width": null,
+ "object_fit": null,
+ "object_position": null,
+ "order": null,
+ "overflow": null,
+ "overflow_x": null,
+ "overflow_y": null,
+ "padding": null,
+ "right": null,
+ "top": null,
+ "visibility": null,
+ "width": null
+ }
+ },
+ "a2cd61263e2e41e59d3e32a0bafe149a": {
+ "model_module": "@jupyter-widgets/base",
+ "model_module_version": "1.2.0",
+ "model_name": "LayoutModel",
+ "state": {
+ "_model_module": "@jupyter-widgets/base",
+ "_model_module_version": "1.2.0",
+ "_model_name": "LayoutModel",
+ "_view_count": null,
+ "_view_module": "@jupyter-widgets/base",
+ "_view_module_version": "1.2.0",
+ "_view_name": "LayoutView",
+ "align_content": null,
+ "align_items": null,
+ "align_self": null,
+ "border": null,
+ "bottom": null,
+ "display": null,
+ "flex": null,
+ "flex_flow": null,
+ "grid_area": null,
+ "grid_auto_columns": null,
+ "grid_auto_flow": null,
+ "grid_auto_rows": null,
+ "grid_column": null,
+ "grid_gap": null,
+ "grid_row": null,
+ "grid_template_areas": null,
+ "grid_template_columns": null,
+ "grid_template_rows": null,
+ "height": null,
+ "justify_content": null,
+ "justify_items": null,
+ "left": null,
+ "margin": null,
+ "max_height": null,
+ "max_width": null,
+ "min_height": null,
+ "min_width": null,
+ "object_fit": null,
+ "object_position": null,
+ "order": null,
+ "overflow": null,
+ "overflow_x": null,
+ "overflow_y": null,
+ "padding": null,
+ "right": null,
+ "top": null,
+ "visibility": null,
+ "width": null
+ }
+ },
+ "a45a2501e43448289e482a5713c5fa91": {
+ "model_module": "@jupyter-widgets/controls",
+ "model_module_version": "1.5.0",
+ "model_name": "HTMLModel",
+ "state": {
+ "_dom_classes": [],
+ "_model_module": "@jupyter-widgets/controls",
+ "_model_module_version": "1.5.0",
+ "_model_name": "HTMLModel",
+ "_view_count": null,
+ "_view_module": "@jupyter-widgets/controls",
+ "_view_module_version": "1.5.0",
+ "_view_name": "HTMLView",
+ "description": "",
+ "description_tooltip": null,
+ "layout": "IPY_MODEL_9551ec31f22a4e5fb3c7b6aa618d7f09",
+ "placeholder": "",
+ "style": "IPY_MODEL_de2e8bd2816b4b2990a78bdb5091f097",
+ "value": " 862k/862k [00:00<00:00, 11.3MB/s]"
+ }
+ },
+ "a4eddaf970084d9ba057e7038423be01": {
+ "model_module": "@jupyter-widgets/controls",
+ "model_module_version": "1.5.0",
+ "model_name": "HTMLModel",
+ "state": {
+ "_dom_classes": [],
+ "_model_module": "@jupyter-widgets/controls",
+ "_model_module_version": "1.5.0",
+ "_model_name": "HTMLModel",
+ "_view_count": null,
+ "_view_module": "@jupyter-widgets/controls",
+ "_view_module_version": "1.5.0",
+ "_view_name": "HTMLView",
+ "description": "",
+ "description_tooltip": null,
+ "layout": "IPY_MODEL_af229a4850174254b09f850c67aefe3a",
+ "placeholder": "",
+ "style": "IPY_MODEL_b007afd6777e4364a57a717088356780",
+ "value": "preprocessor_config.json: 100%"
+ }
+ },
+ "a5b8a21a005d4ef7b253b782dae3b88d": {
+ "model_module": "@jupyter-widgets/base",
+ "model_module_version": "1.2.0",
+ "model_name": "LayoutModel",
+ "state": {
+ "_model_module": "@jupyter-widgets/base",
+ "_model_module_version": "1.2.0",
+ "_model_name": "LayoutModel",
+ "_view_count": null,
+ "_view_module": "@jupyter-widgets/base",
+ "_view_module_version": "1.2.0",
+ "_view_name": "LayoutView",
+ "align_content": null,
+ "align_items": null,
+ "align_self": null,
+ "border": null,
+ "bottom": null,
+ "display": null,
+ "flex": null,
+ "flex_flow": null,
+ "grid_area": null,
+ "grid_auto_columns": null,
+ "grid_auto_flow": null,
+ "grid_auto_rows": null,
+ "grid_column": null,
+ "grid_gap": null,
+ "grid_row": null,
+ "grid_template_areas": null,
+ "grid_template_columns": null,
+ "grid_template_rows": null,
+ "height": null,
+ "justify_content": null,
+ "justify_items": null,
+ "left": null,
+ "margin": null,
+ "max_height": null,
+ "max_width": null,
+ "min_height": null,
+ "min_width": null,
+ "object_fit": null,
+ "object_position": null,
+ "order": null,
+ "overflow": null,
+ "overflow_x": null,
+ "overflow_y": null,
+ "padding": null,
+ "right": null,
+ "top": null,
+ "visibility": null,
+ "width": null
+ }
+ },
+ "a6bd1a75b94f4809b5d275db402f1751": {
+ "model_module": "@jupyter-widgets/controls",
+ "model_module_version": "1.5.0",
+ "model_name": "HTMLModel",
+ "state": {
+ "_dom_classes": [],
+ "_model_module": "@jupyter-widgets/controls",
+ "_model_module_version": "1.5.0",
+ "_model_name": "HTMLModel",
+ "_view_count": null,
+ "_view_module": "@jupyter-widgets/controls",
+ "_view_module_version": "1.5.0",
+ "_view_name": "HTMLView",
+ "description": "",
+ "description_tooltip": null,
+ "layout": "IPY_MODEL_6ac5948711754a6c9ef851f6db861e72",
+ "placeholder": "",
+ "style": "IPY_MODEL_096f5fba1a1e4fbe82d0411363b8c477",
+ "value": "tokenizer_config.json: 100%"
+ }
+ },
+ "a6e1fe5e2caf42b2968a19df388daf66": {
+ "model_module": "@jupyter-widgets/controls",
+ "model_module_version": "1.5.0",
+ "model_name": "HTMLModel",
+ "state": {
+ "_dom_classes": [],
+ "_model_module": "@jupyter-widgets/controls",
+ "_model_module_version": "1.5.0",
+ "_model_name": "HTMLModel",
+ "_view_count": null,
+ "_view_module": "@jupyter-widgets/controls",
+ "_view_module_version": "1.5.0",
+ "_view_name": "HTMLView",
+ "description": "",
+ "description_tooltip": null,
+ "layout": "IPY_MODEL_15b5253136ec4e7db56e103960f4d3f6",
+ "placeholder": "",
+ "style": "IPY_MODEL_f0ff7fa2d15f41b4b6fae00cb2936acd",
+ "value": " 844/844 [00:00<00:00, 64.4kB/s]"
+ }
+ },
+ "af229a4850174254b09f850c67aefe3a": {
+ "model_module": "@jupyter-widgets/base",
+ "model_module_version": "1.2.0",
+ "model_name": "LayoutModel",
+ "state": {
+ "_model_module": "@jupyter-widgets/base",
+ "_model_module_version": "1.2.0",
+ "_model_name": "LayoutModel",
+ "_view_count": null,
+ "_view_module": "@jupyter-widgets/base",
+ "_view_module_version": "1.2.0",
+ "_view_name": "LayoutView",
+ "align_content": null,
+ "align_items": null,
+ "align_self": null,
+ "border": null,
+ "bottom": null,
+ "display": null,
+ "flex": null,
+ "flex_flow": null,
+ "grid_area": null,
+ "grid_auto_columns": null,
+ "grid_auto_flow": null,
+ "grid_auto_rows": null,
+ "grid_column": null,
+ "grid_gap": null,
+ "grid_row": null,
+ "grid_template_areas": null,
+ "grid_template_columns": null,
+ "grid_template_rows": null,
+ "height": null,
+ "justify_content": null,
+ "justify_items": null,
+ "left": null,
+ "margin": null,
+ "max_height": null,
+ "max_width": null,
+ "min_height": null,
+ "min_width": null,
+ "object_fit": null,
+ "object_position": null,
+ "order": null,
+ "overflow": null,
+ "overflow_x": null,
+ "overflow_y": null,
+ "padding": null,
+ "right": null,
+ "top": null,
+ "visibility": null,
+ "width": null
+ }
+ },
+ "b007afd6777e4364a57a717088356780": {
+ "model_module": "@jupyter-widgets/controls",
+ "model_module_version": "1.5.0",
+ "model_name": "DescriptionStyleModel",
+ "state": {
+ "_model_module": "@jupyter-widgets/controls",
+ "_model_module_version": "1.5.0",
+ "_model_name": "DescriptionStyleModel",
+ "_view_count": null,
+ "_view_module": "@jupyter-widgets/base",
+ "_view_module_version": "1.2.0",
+ "_view_name": "StyleView",
+ "description_width": ""
+ }
+ },
+ "b1b3b62f8d7545938d1e2d8f8757c589": {
+ "model_module": "@jupyter-widgets/base",
+ "model_module_version": "1.2.0",
+ "model_name": "LayoutModel",
+ "state": {
+ "_model_module": "@jupyter-widgets/base",
+ "_model_module_version": "1.2.0",
+ "_model_name": "LayoutModel",
+ "_view_count": null,
+ "_view_module": "@jupyter-widgets/base",
+ "_view_module_version": "1.2.0",
+ "_view_name": "LayoutView",
+ "align_content": null,
+ "align_items": null,
+ "align_self": null,
+ "border": null,
+ "bottom": null,
+ "display": null,
+ "flex": null,
+ "flex_flow": null,
+ "grid_area": null,
+ "grid_auto_columns": null,
+ "grid_auto_flow": null,
+ "grid_auto_rows": null,
+ "grid_column": null,
+ "grid_gap": null,
+ "grid_row": null,
+ "grid_template_areas": null,
+ "grid_template_columns": null,
+ "grid_template_rows": null,
+ "height": null,
+ "justify_content": null,
+ "justify_items": null,
+ "left": null,
+ "margin": null,
+ "max_height": null,
+ "max_width": null,
+ "min_height": null,
+ "min_width": null,
+ "object_fit": null,
+ "object_position": null,
+ "order": null,
+ "overflow": null,
+ "overflow_x": null,
+ "overflow_y": null,
+ "padding": null,
+ "right": null,
+ "top": null,
+ "visibility": null,
+ "width": null
+ }
+ },
+ "b1cc5c487a364d3ba8d33e0aa3b2a305": {
+ "model_module": "@jupyter-widgets/base",
+ "model_module_version": "1.2.0",
+ "model_name": "LayoutModel",
+ "state": {
+ "_model_module": "@jupyter-widgets/base",
+ "_model_module_version": "1.2.0",
+ "_model_name": "LayoutModel",
+ "_view_count": null,
+ "_view_module": "@jupyter-widgets/base",
+ "_view_module_version": "1.2.0",
+ "_view_name": "LayoutView",
+ "align_content": null,
+ "align_items": null,
+ "align_self": null,
+ "border": null,
+ "bottom": null,
+ "display": null,
+ "flex": null,
+ "flex_flow": null,
+ "grid_area": null,
+ "grid_auto_columns": null,
+ "grid_auto_flow": null,
+ "grid_auto_rows": null,
+ "grid_column": null,
+ "grid_gap": null,
+ "grid_row": null,
+ "grid_template_areas": null,
+ "grid_template_columns": null,
+ "grid_template_rows": null,
+ "height": null,
+ "justify_content": null,
+ "justify_items": null,
+ "left": null,
+ "margin": null,
+ "max_height": null,
+ "max_width": null,
+ "min_height": null,
+ "min_width": null,
+ "object_fit": null,
+ "object_position": null,
+ "order": null,
+ "overflow": null,
+ "overflow_x": null,
+ "overflow_y": null,
+ "padding": null,
+ "right": null,
+ "top": null,
+ "visibility": null,
+ "width": null
+ }
+ },
+ "b2d0b2f0ec7648b89fc19a1dda8462ba": {
+ "model_module": "@jupyter-widgets/base",
+ "model_module_version": "1.2.0",
+ "model_name": "LayoutModel",
+ "state": {
+ "_model_module": "@jupyter-widgets/base",
+ "_model_module_version": "1.2.0",
+ "_model_name": "LayoutModel",
+ "_view_count": null,
+ "_view_module": "@jupyter-widgets/base",
+ "_view_module_version": "1.2.0",
+ "_view_name": "LayoutView",
+ "align_content": null,
+ "align_items": null,
+ "align_self": null,
+ "border": null,
+ "bottom": null,
+ "display": null,
+ "flex": null,
+ "flex_flow": null,
+ "grid_area": null,
+ "grid_auto_columns": null,
+ "grid_auto_flow": null,
+ "grid_auto_rows": null,
+ "grid_column": null,
+ "grid_gap": null,
+ "grid_row": null,
+ "grid_template_areas": null,
+ "grid_template_columns": null,
+ "grid_template_rows": null,
+ "height": null,
+ "justify_content": null,
+ "justify_items": null,
+ "left": null,
+ "margin": null,
+ "max_height": null,
+ "max_width": null,
+ "min_height": null,
+ "min_width": null,
+ "object_fit": null,
+ "object_position": null,
+ "order": null,
+ "overflow": null,
+ "overflow_x": null,
+ "overflow_y": null,
+ "padding": null,
+ "right": null,
+ "top": null,
+ "visibility": null,
+ "width": null
+ }
+ },
+ "b3bf65796de8494e82506f6849316426": {
+ "model_module": "@jupyter-widgets/base",
+ "model_module_version": "1.2.0",
+ "model_name": "LayoutModel",
+ "state": {
+ "_model_module": "@jupyter-widgets/base",
+ "_model_module_version": "1.2.0",
+ "_model_name": "LayoutModel",
+ "_view_count": null,
+ "_view_module": "@jupyter-widgets/base",
+ "_view_module_version": "1.2.0",
+ "_view_name": "LayoutView",
+ "align_content": null,
+ "align_items": null,
+ "align_self": null,
+ "border": null,
+ "bottom": null,
+ "display": null,
+ "flex": null,
+ "flex_flow": null,
+ "grid_area": null,
+ "grid_auto_columns": null,
+ "grid_auto_flow": null,
+ "grid_auto_rows": null,
+ "grid_column": null,
+ "grid_gap": null,
+ "grid_row": null,
+ "grid_template_areas": null,
+ "grid_template_columns": null,
+ "grid_template_rows": null,
+ "height": null,
+ "justify_content": null,
+ "justify_items": null,
+ "left": null,
+ "margin": null,
+ "max_height": null,
+ "max_width": null,
+ "min_height": null,
+ "min_width": null,
+ "object_fit": null,
+ "object_position": null,
+ "order": null,
+ "overflow": null,
+ "overflow_x": null,
+ "overflow_y": null,
+ "padding": null,
+ "right": null,
+ "top": null,
+ "visibility": null,
+ "width": null
+ }
+ },
+ "b8924ff1bbb5409c8dc141e65c4cdcdb": {
+ "model_module": "@jupyter-widgets/controls",
+ "model_module_version": "1.5.0",
+ "model_name": "FloatProgressModel",
+ "state": {
+ "_dom_classes": [],
+ "_model_module": "@jupyter-widgets/controls",
+ "_model_module_version": "1.5.0",
+ "_model_name": "FloatProgressModel",
+ "_view_count": null,
+ "_view_module": "@jupyter-widgets/controls",
+ "_view_module_version": "1.5.0",
+ "_view_name": "ProgressView",
+ "bar_style": "success",
+ "description": "",
+ "description_tooltip": null,
+ "layout": "IPY_MODEL_b1b3b62f8d7545938d1e2d8f8757c589",
+ "max": 1711974081,
+ "min": 0,
+ "orientation": "horizontal",
+ "style": "IPY_MODEL_2d744d00de6745bda5835f0cd66e3909",
+ "value": 1711974081
+ }
+ },
+ "b8bd9787d9c640e19798be15e94ede04": {
+ "model_module": "@jupyter-widgets/base",
+ "model_module_version": "1.2.0",
+ "model_name": "LayoutModel",
+ "state": {
+ "_model_module": "@jupyter-widgets/base",
+ "_model_module_version": "1.2.0",
+ "_model_name": "LayoutModel",
+ "_view_count": null,
+ "_view_module": "@jupyter-widgets/base",
+ "_view_module_version": "1.2.0",
+ "_view_name": "LayoutView",
+ "align_content": null,
+ "align_items": null,
+ "align_self": null,
+ "border": null,
+ "bottom": null,
+ "display": null,
+ "flex": null,
+ "flex_flow": null,
+ "grid_area": null,
+ "grid_auto_columns": null,
+ "grid_auto_flow": null,
+ "grid_auto_rows": null,
+ "grid_column": null,
+ "grid_gap": null,
+ "grid_row": null,
+ "grid_template_areas": null,
+ "grid_template_columns": null,
+ "grid_template_rows": null,
+ "height": null,
+ "justify_content": null,
+ "justify_items": null,
+ "left": null,
+ "margin": null,
+ "max_height": null,
+ "max_width": null,
+ "min_height": null,
+ "min_width": null,
+ "object_fit": null,
+ "object_position": null,
+ "order": null,
+ "overflow": null,
+ "overflow_x": null,
+ "overflow_y": null,
+ "padding": null,
+ "right": null,
+ "top": null,
+ "visibility": null,
+ "width": null
+ }
+ },
+ "c06b5a6588eb42189210d1c20ccba87a": {
+ "model_module": "@jupyter-widgets/controls",
+ "model_module_version": "1.5.0",
+ "model_name": "HBoxModel",
+ "state": {
+ "_dom_classes": [],
+ "_model_module": "@jupyter-widgets/controls",
+ "_model_module_version": "1.5.0",
+ "_model_name": "HBoxModel",
+ "_view_count": null,
+ "_view_module": "@jupyter-widgets/controls",
+ "_view_module_version": "1.5.0",
+ "_view_name": "HBoxView",
+ "box_style": "",
+ "children": [
+ "IPY_MODEL_da46a678b1fc40d7b660de63d9202997",
+ "IPY_MODEL_c0e3b6e7e7304dc9877d6800f924d05e",
+ "IPY_MODEL_e7035db245c7430c92ceb5b61c31ba14"
+ ],
+ "layout": "IPY_MODEL_d0d7ebc4ce264a6b8ae5c6ba4e18a9b3"
+ }
+ },
+ "c0e3b6e7e7304dc9877d6800f924d05e": {
+ "model_module": "@jupyter-widgets/controls",
+ "model_module_version": "1.5.0",
+ "model_name": "FloatProgressModel",
+ "state": {
+ "_dom_classes": [],
+ "_model_module": "@jupyter-widgets/controls",
+ "_model_module_version": "1.5.0",
+ "_model_name": "FloatProgressModel",
+ "_view_count": null,
+ "_view_module": "@jupyter-widgets/controls",
+ "_view_module_version": "1.5.0",
+ "_view_name": "ProgressView",
+ "bar_style": "success",
+ "description": "",
+ "description_tooltip": null,
+ "layout": "IPY_MODEL_837c2d8dd75342a8bbeb1c5ce899e759",
+ "max": 524657,
+ "min": 0,
+ "orientation": "horizontal",
+ "style": "IPY_MODEL_95649d04b8b144b091bba9e8106a44d6",
+ "value": 524657
+ }
+ },
+ "c37415464174453b9ce13466ed6ff20c": {
+ "model_module": "@jupyter-widgets/controls",
+ "model_module_version": "1.5.0",
+ "model_name": "ProgressStyleModel",
+ "state": {
+ "_model_module": "@jupyter-widgets/controls",
+ "_model_module_version": "1.5.0",
+ "_model_name": "ProgressStyleModel",
+ "_view_count": null,
+ "_view_module": "@jupyter-widgets/base",
+ "_view_module_version": "1.2.0",
+ "_view_name": "StyleView",
+ "bar_color": null,
+ "description_width": ""
+ }
+ },
+ "c9fdbe12b7934fd8accc09645df0f214": {
+ "model_module": "@jupyter-widgets/controls",
+ "model_module_version": "1.5.0",
+ "model_name": "ProgressStyleModel",
+ "state": {
+ "_model_module": "@jupyter-widgets/controls",
+ "_model_module_version": "1.5.0",
+ "_model_name": "ProgressStyleModel",
+ "_view_count": null,
+ "_view_module": "@jupyter-widgets/base",
+ "_view_module_version": "1.2.0",
+ "_view_name": "StyleView",
+ "bar_color": null,
+ "description_width": ""
+ }
+ },
+ "cb386abe77244108b8f98de9ad3f1bdd": {
+ "model_module": "@jupyter-widgets/base",
+ "model_module_version": "1.2.0",
+ "model_name": "LayoutModel",
+ "state": {
+ "_model_module": "@jupyter-widgets/base",
+ "_model_module_version": "1.2.0",
+ "_model_name": "LayoutModel",
+ "_view_count": null,
+ "_view_module": "@jupyter-widgets/base",
+ "_view_module_version": "1.2.0",
+ "_view_name": "LayoutView",
+ "align_content": null,
+ "align_items": null,
+ "align_self": null,
+ "border": null,
+ "bottom": null,
+ "display": null,
+ "flex": null,
+ "flex_flow": null,
+ "grid_area": null,
+ "grid_auto_columns": null,
+ "grid_auto_flow": null,
+ "grid_auto_rows": null,
+ "grid_column": null,
+ "grid_gap": null,
+ "grid_row": null,
+ "grid_template_areas": null,
+ "grid_template_columns": null,
+ "grid_template_rows": null,
+ "height": null,
+ "justify_content": null,
+ "justify_items": null,
+ "left": null,
+ "margin": null,
+ "max_height": null,
+ "max_width": null,
+ "min_height": null,
+ "min_width": null,
+ "object_fit": null,
+ "object_position": null,
+ "order": null,
+ "overflow": null,
+ "overflow_x": null,
+ "overflow_y": null,
+ "padding": null,
+ "right": null,
+ "top": null,
+ "visibility": null,
+ "width": null
+ }
+ },
+ "d0d7ebc4ce264a6b8ae5c6ba4e18a9b3": {
+ "model_module": "@jupyter-widgets/base",
+ "model_module_version": "1.2.0",
+ "model_name": "LayoutModel",
+ "state": {
+ "_model_module": "@jupyter-widgets/base",
+ "_model_module_version": "1.2.0",
+ "_model_name": "LayoutModel",
+ "_view_count": null,
+ "_view_module": "@jupyter-widgets/base",
+ "_view_module_version": "1.2.0",
+ "_view_name": "LayoutView",
+ "align_content": null,
+ "align_items": null,
+ "align_self": null,
+ "border": null,
+ "bottom": null,
+ "display": null,
+ "flex": null,
+ "flex_flow": null,
+ "grid_area": null,
+ "grid_auto_columns": null,
+ "grid_auto_flow": null,
+ "grid_auto_rows": null,
+ "grid_column": null,
+ "grid_gap": null,
+ "grid_row": null,
+ "grid_template_areas": null,
+ "grid_template_columns": null,
+ "grid_template_rows": null,
+ "height": null,
+ "justify_content": null,
+ "justify_items": null,
+ "left": null,
+ "margin": null,
+ "max_height": null,
+ "max_width": null,
+ "min_height": null,
+ "min_width": null,
+ "object_fit": null,
+ "object_position": null,
+ "order": null,
+ "overflow": null,
+ "overflow_x": null,
+ "overflow_y": null,
+ "padding": null,
+ "right": null,
+ "top": null,
+ "visibility": null,
+ "width": null
+ }
+ },
+ "d5611bb67e8d49f19e2700652d5309c1": {
+ "model_module": "@jupyter-widgets/controls",
+ "model_module_version": "1.5.0",
+ "model_name": "FloatProgressModel",
+ "state": {
+ "_dom_classes": [],
+ "_model_module": "@jupyter-widgets/controls",
+ "_model_module_version": "1.5.0",
+ "_model_name": "FloatProgressModel",
+ "_view_count": null,
+ "_view_module": "@jupyter-widgets/controls",
+ "_view_module_version": "1.5.0",
+ "_view_name": "ProgressView",
+ "bar_style": "success",
+ "description": "",
+ "description_tooltip": null,
+ "layout": "IPY_MODEL_380b18596be246d6bc6fd4412fd20379",
+ "max": 389,
+ "min": 0,
+ "orientation": "horizontal",
+ "style": "IPY_MODEL_2d6ea61d0fa84510b44fff80ab10e553",
+ "value": 389
+ }
+ },
+ "da46a678b1fc40d7b660de63d9202997": {
+ "model_module": "@jupyter-widgets/controls",
+ "model_module_version": "1.5.0",
+ "model_name": "HTMLModel",
+ "state": {
+ "_dom_classes": [],
+ "_model_module": "@jupyter-widgets/controls",
+ "_model_module_version": "1.5.0",
+ "_model_name": "HTMLModel",
+ "_view_count": null,
+ "_view_module": "@jupyter-widgets/controls",
+ "_view_module_version": "1.5.0",
+ "_view_name": "HTMLView",
+ "description": "",
+ "description_tooltip": null,
+ "layout": "IPY_MODEL_0c46bf3c0a1840cfba66afef11e16cd2",
+ "placeholder": "",
+ "style": "IPY_MODEL_2a1f21cd845e44c89197edc86b482b71",
+ "value": "merges.txt: 100%"
+ }
+ },
+ "de2e8bd2816b4b2990a78bdb5091f097": {
+ "model_module": "@jupyter-widgets/controls",
+ "model_module_version": "1.5.0",
+ "model_name": "DescriptionStyleModel",
+ "state": {
+ "_model_module": "@jupyter-widgets/controls",
+ "_model_module_version": "1.5.0",
+ "_model_name": "DescriptionStyleModel",
+ "_view_count": null,
+ "_view_module": "@jupyter-widgets/base",
+ "_view_module_version": "1.2.0",
+ "_view_name": "StyleView",
+ "description_width": ""
+ }
+ },
+ "dfbe3622fad14e798c9f413a134fd107": {
+ "model_module": "@jupyter-widgets/base",
+ "model_module_version": "1.2.0",
+ "model_name": "LayoutModel",
+ "state": {
+ "_model_module": "@jupyter-widgets/base",
+ "_model_module_version": "1.2.0",
+ "_model_name": "LayoutModel",
+ "_view_count": null,
+ "_view_module": "@jupyter-widgets/base",
+ "_view_module_version": "1.2.0",
+ "_view_name": "LayoutView",
+ "align_content": null,
+ "align_items": null,
+ "align_self": null,
+ "border": null,
+ "bottom": null,
+ "display": null,
+ "flex": null,
+ "flex_flow": null,
+ "grid_area": null,
+ "grid_auto_columns": null,
+ "grid_auto_flow": null,
+ "grid_auto_rows": null,
+ "grid_column": null,
+ "grid_gap": null,
+ "grid_row": null,
+ "grid_template_areas": null,
+ "grid_template_columns": null,
+ "grid_template_rows": null,
+ "height": null,
+ "justify_content": null,
+ "justify_items": null,
+ "left": null,
+ "margin": null,
+ "max_height": null,
+ "max_width": null,
+ "min_height": null,
+ "min_width": null,
+ "object_fit": null,
+ "object_position": null,
+ "order": null,
+ "overflow": null,
+ "overflow_x": null,
+ "overflow_y": null,
+ "padding": null,
+ "right": null,
+ "top": null,
+ "visibility": null,
+ "width": null
+ }
+ },
+ "e191179e7e4048b69b47d3b9b550b459": {
+ "model_module": "@jupyter-widgets/controls",
+ "model_module_version": "1.5.0",
+ "model_name": "DescriptionStyleModel",
+ "state": {
+ "_model_module": "@jupyter-widgets/controls",
+ "_model_module_version": "1.5.0",
+ "_model_name": "DescriptionStyleModel",
+ "_view_count": null,
+ "_view_module": "@jupyter-widgets/base",
+ "_view_module_version": "1.2.0",
+ "_view_name": "StyleView",
+ "description_width": ""
+ }
+ },
+ "e2bc0bc25b5044abb8bb1c752935922e": {
+ "model_module": "@jupyter-widgets/controls",
+ "model_module_version": "1.5.0",
+ "model_name": "DescriptionStyleModel",
+ "state": {
+ "_model_module": "@jupyter-widgets/controls",
+ "_model_module_version": "1.5.0",
+ "_model_name": "DescriptionStyleModel",
+ "_view_count": null,
+ "_view_module": "@jupyter-widgets/base",
+ "_view_module_version": "1.2.0",
+ "_view_name": "StyleView",
+ "description_width": ""
+ }
+ },
+ "e7035db245c7430c92ceb5b61c31ba14": {
+ "model_module": "@jupyter-widgets/controls",
+ "model_module_version": "1.5.0",
+ "model_name": "HTMLModel",
+ "state": {
+ "_dom_classes": [],
+ "_model_module": "@jupyter-widgets/controls",
+ "_model_module_version": "1.5.0",
+ "_model_name": "HTMLModel",
+ "_view_count": null,
+ "_view_module": "@jupyter-widgets/controls",
+ "_view_module_version": "1.5.0",
+ "_view_name": "HTMLView",
+ "description": "",
+ "description_tooltip": null,
+ "layout": "IPY_MODEL_081d380b0c52402abfd57337726b1aa3",
+ "placeholder": "",
+ "style": "IPY_MODEL_5da887b8b4fd4437846c639b3ffb575b",
+ "value": " 525k/525k [00:00<00:00, 11.2MB/s]"
+ }
+ },
+ "e7e79c91380c478dabb2b1e7ddca647e": {
+ "model_module": "@jupyter-widgets/controls",
+ "model_module_version": "1.5.0",
+ "model_name": "DescriptionStyleModel",
+ "state": {
+ "_model_module": "@jupyter-widgets/controls",
+ "_model_module_version": "1.5.0",
+ "_model_name": "DescriptionStyleModel",
+ "_view_count": null,
+ "_view_module": "@jupyter-widgets/base",
+ "_view_module_version": "1.2.0",
+ "_view_name": "StyleView",
+ "description_width": ""
+ }
+ },
+ "ec7bc6e82f2042b8b29a6f21e6db1709": {
+ "model_module": "@jupyter-widgets/controls",
+ "model_module_version": "1.5.0",
+ "model_name": "HBoxModel",
+ "state": {
+ "_dom_classes": [],
+ "_model_module": "@jupyter-widgets/controls",
+ "_model_module_version": "1.5.0",
+ "_model_name": "HBoxModel",
+ "_view_count": null,
+ "_view_module": "@jupyter-widgets/controls",
+ "_view_module_version": "1.5.0",
+ "_view_name": "HBoxView",
+ "box_style": "",
+ "children": [
+ "IPY_MODEL_609cc0908e6f4edd95306f72b40afd0c",
+ "IPY_MODEL_d5611bb67e8d49f19e2700652d5309c1",
+ "IPY_MODEL_fed0f8954a6b4e1194c63ccc9fba1238"
+ ],
+ "layout": "IPY_MODEL_174aacf5b59048b6ad27a6dffeb87950"
+ }
+ },
+ "ef6fd54de3aa46af868c8c810068d9ad": {
+ "model_module": "@jupyter-widgets/base",
+ "model_module_version": "1.2.0",
+ "model_name": "LayoutModel",
+ "state": {
+ "_model_module": "@jupyter-widgets/base",
+ "_model_module_version": "1.2.0",
+ "_model_name": "LayoutModel",
+ "_view_count": null,
+ "_view_module": "@jupyter-widgets/base",
+ "_view_module_version": "1.2.0",
+ "_view_name": "LayoutView",
+ "align_content": null,
+ "align_items": null,
+ "align_self": null,
+ "border": null,
+ "bottom": null,
+ "display": null,
+ "flex": null,
+ "flex_flow": null,
+ "grid_area": null,
+ "grid_auto_columns": null,
+ "grid_auto_flow": null,
+ "grid_auto_rows": null,
+ "grid_column": null,
+ "grid_gap": null,
+ "grid_row": null,
+ "grid_template_areas": null,
+ "grid_template_columns": null,
+ "grid_template_rows": null,
+ "height": null,
+ "justify_content": null,
+ "justify_items": null,
+ "left": null,
+ "margin": null,
+ "max_height": null,
+ "max_width": null,
+ "min_height": null,
+ "min_width": null,
+ "object_fit": null,
+ "object_position": null,
+ "order": null,
+ "overflow": null,
+ "overflow_x": null,
+ "overflow_y": null,
+ "padding": null,
+ "right": null,
+ "top": null,
+ "visibility": null,
+ "width": null
+ }
+ },
+ "effcd9cbd407405cbbffe4e76b19df72": {
+ "model_module": "@jupyter-widgets/controls",
+ "model_module_version": "1.5.0",
+ "model_name": "FloatProgressModel",
+ "state": {
+ "_dom_classes": [],
+ "_model_module": "@jupyter-widgets/controls",
+ "_model_module_version": "1.5.0",
+ "_model_name": "FloatProgressModel",
+ "_view_count": null,
+ "_view_module": "@jupyter-widgets/controls",
+ "_view_module_version": "1.5.0",
+ "_view_name": "ProgressView",
+ "bar_style": "success",
+ "description": "",
+ "description_tooltip": null,
+ "layout": "IPY_MODEL_9b1db90efb8b4ef096c5254494c063db",
+ "max": 4757,
+ "min": 0,
+ "orientation": "horizontal",
+ "style": "IPY_MODEL_c9fdbe12b7934fd8accc09645df0f214",
+ "value": 4757
+ }
+ },
+ "f0af662a1a884fb78c693ccf0d0b6d8e": {
+ "model_module": "@jupyter-widgets/controls",
+ "model_module_version": "1.5.0",
+ "model_name": "FloatProgressModel",
+ "state": {
+ "_dom_classes": [],
+ "_model_module": "@jupyter-widgets/controls",
+ "_model_module_version": "1.5.0",
+ "_model_name": "FloatProgressModel",
+ "_view_count": null,
+ "_view_module": "@jupyter-widgets/controls",
+ "_view_module_version": "1.5.0",
+ "_view_name": "ProgressView",
+ "bar_style": "success",
+ "description": "",
+ "description_tooltip": null,
+ "layout": "IPY_MODEL_4609b1b46de5441a9632985833bd0b05",
+ "max": 316,
+ "min": 0,
+ "orientation": "horizontal",
+ "style": "IPY_MODEL_5c5e2b0d9fa7435a92c95d417ed03956",
+ "value": 316
+ }
+ },
+ "f0ff7fa2d15f41b4b6fae00cb2936acd": {
+ "model_module": "@jupyter-widgets/controls",
+ "model_module_version": "1.5.0",
+ "model_name": "DescriptionStyleModel",
+ "state": {
+ "_model_module": "@jupyter-widgets/controls",
+ "_model_module_version": "1.5.0",
+ "_model_name": "DescriptionStyleModel",
+ "_view_count": null,
+ "_view_module": "@jupyter-widgets/base",
+ "_view_module_version": "1.2.0",
+ "_view_name": "StyleView",
+ "description_width": ""
+ }
+ },
+ "f359ba8ef0cf4841b40acafcd770480c": {
+ "model_module": "@jupyter-widgets/controls",
+ "model_module_version": "1.5.0",
+ "model_name": "HBoxModel",
+ "state": {
+ "_dom_classes": [],
+ "_model_module": "@jupyter-widgets/controls",
+ "_model_module_version": "1.5.0",
+ "_model_name": "HBoxModel",
+ "_view_count": null,
+ "_view_module": "@jupyter-widgets/controls",
+ "_view_module_version": "1.5.0",
+ "_view_name": "HBoxView",
+ "box_style": "",
+ "children": [
+ "IPY_MODEL_0861f873665f4514abfd2b09ad944ab8",
+ "IPY_MODEL_b8924ff1bbb5409c8dc141e65c4cdcdb",
+ "IPY_MODEL_4b62b742aacb45c7a286a5d85a0194da"
+ ],
+ "layout": "IPY_MODEL_dfbe3622fad14e798c9f413a134fd107"
+ }
+ },
+ "fa6ed2fba5bf4abdaceefc180e4f9a41": {
+ "model_module": "@jupyter-widgets/base",
+ "model_module_version": "1.2.0",
+ "model_name": "LayoutModel",
+ "state": {
+ "_model_module": "@jupyter-widgets/base",
+ "_model_module_version": "1.2.0",
+ "_model_name": "LayoutModel",
+ "_view_count": null,
+ "_view_module": "@jupyter-widgets/base",
+ "_view_module_version": "1.2.0",
+ "_view_name": "LayoutView",
+ "align_content": null,
+ "align_items": null,
+ "align_self": null,
+ "border": null,
+ "bottom": null,
+ "display": null,
+ "flex": null,
+ "flex_flow": null,
+ "grid_area": null,
+ "grid_auto_columns": null,
+ "grid_auto_flow": null,
+ "grid_auto_rows": null,
+ "grid_column": null,
+ "grid_gap": null,
+ "grid_row": null,
+ "grid_template_areas": null,
+ "grid_template_columns": null,
+ "grid_template_rows": null,
+ "height": null,
+ "justify_content": null,
+ "justify_items": null,
+ "left": null,
+ "margin": null,
+ "max_height": null,
+ "max_width": null,
+ "min_height": null,
+ "min_width": null,
+ "object_fit": null,
+ "object_position": null,
+ "order": null,
+ "overflow": null,
+ "overflow_x": null,
+ "overflow_y": null,
+ "padding": null,
+ "right": null,
+ "top": null,
+ "visibility": null,
+ "width": null
+ }
+ },
+ "fc83fdb519174250ae312080e2918abe": {
+ "model_module": "@jupyter-widgets/controls",
+ "model_module_version": "1.5.0",
+ "model_name": "ProgressStyleModel",
+ "state": {
+ "_model_module": "@jupyter-widgets/controls",
+ "_model_module_version": "1.5.0",
+ "_model_name": "ProgressStyleModel",
+ "_view_count": null,
+ "_view_module": "@jupyter-widgets/base",
+ "_view_module_version": "1.2.0",
+ "_view_name": "StyleView",
+ "bar_color": null,
+ "description_width": ""
+ }
+ },
+ "fed0f8954a6b4e1194c63ccc9fba1238": {
+ "model_module": "@jupyter-widgets/controls",
+ "model_module_version": "1.5.0",
+ "model_name": "HTMLModel",
+ "state": {
+ "_dom_classes": [],
+ "_model_module": "@jupyter-widgets/controls",
+ "_model_module_version": "1.5.0",
+ "_model_name": "HTMLModel",
+ "_view_count": null,
+ "_view_module": "@jupyter-widgets/controls",
+ "_view_module_version": "1.5.0",
+ "_view_name": "HTMLView",
+ "description": "",
+ "description_tooltip": null,
+ "layout": "IPY_MODEL_098832b366c6410b824d2c210222dc24",
+ "placeholder": "",
+ "style": "IPY_MODEL_e7e79c91380c478dabb2b1e7ddca647e",
+ "value": " 389/389 [00:00<00:00, 27.5kB/s]"
+ }
+ }
+ }
+ }
+ },
+ "nbformat": 4,
+ "nbformat_minor": 0
+}
diff --git a/keras_cv/tools/training_scipts/Training_YOLOv8.ipynb b/keras_cv/tools/training_scipts/Training_YOLOv8.ipynb
new file mode 100644
index 0000000000..dc0cf695e2
--- /dev/null
+++ b/keras_cv/tools/training_scipts/Training_YOLOv8.ipynb
@@ -0,0 +1,3321 @@
+{
+ "nbformat": 4,
+ "nbformat_minor": 0,
+ "metadata": {
+ "colab": {
+ "provenance": [],
+ "gpuType": "A100",
+ "machine_shape": "hm"
+ },
+ "kernelspec": {
+ "name": "python3",
+ "display_name": "Python 3"
+ },
+ "language_info": {
+ "name": "python"
+ },
+ "accelerator": "GPU"
+ },
+ "cells": [
+ {
+ "cell_type": "code",
+ "execution_count": null,
+ "metadata": {
+ "id": "rtDJ7E2lv01f"
+ },
+ "outputs": [],
+ "source": [
+ "!pip install keras-cv keras-core"
+ ]
+ },
+ {
+ "cell_type": "code",
+ "source": [
+ "!pip uninstall -y keras-cv\n",
+ "!pip install git+https://github.com/ianstenbit/keras-cv.git@task-aligned-assignment"
+ ],
+ "metadata": {
+ "id": "0D0rrgB5vJVj"
+ },
+ "execution_count": null,
+ "outputs": []
+ },
+ {
+ "cell_type": "code",
+ "source": [
+ "# Copyright 2022 The KerasCV Authors\n",
+ "#\n",
+ "# Licensed under the Apache License, Version 2.0 (the \"License\");\n",
+ "# you may not use this file except in compliance with the License.\n",
+ "# You may obtain a copy of the License at\n",
+ "#\n",
+ "# https://www.apache.org/licenses/LICENSE-2.0\n",
+ "#\n",
+ "# Unless required by applicable law or agreed to in writing, software\n",
+ "# distributed under the License is distributed on an \"AS IS\" BASIS,\n",
+ "# WITHOUT WARRANTIES OR CONDITIONS OF ANY KIND, either express or implied.\n",
+ "# See the License for the specific language governing permissions and\n",
+ "# limitations under the License.\n",
+ "\"\"\"\n",
+ "Title: Train an Object Detection Model on Pascal VOC 2007 using KerasCV\n",
+ "Author: [lukewood](https://github.com/LukeWood), [tanzhenyu](https://github.com/tanzhenyu)\n",
+ "Date created: 2022/09/27\n",
+ "Last modified: 2023/03/29\n",
+ "Description: Use KerasCV to train a RetinaNet on Pascal VOC 2007.\n",
+ "\"\"\"\n",
+ "import resource\n",
+ "import sys\n",
+ "\n",
+ "import tensorflow as tf\n",
+ "import tensorflow_datasets as tfds\n",
+ "import tqdm\n",
+ "from tensorflow import keras\n",
+ "\n",
+ "import keras_cv\n",
+ "\n",
+ "# Temporarily need PyCOCOCallback to verify\n",
+ "# a 1:1 comparison with the PyMetrics version.\n",
+ "from keras_cv.callbacks import PyCOCOCallback\n",
+ "\n",
+ "low, high = resource.getrlimit(resource.RLIMIT_NOFILE)\n",
+ "resource.setrlimit(resource.RLIMIT_NOFILE, (high, high))"
+ ],
+ "metadata": {
+ "id": "eWYAJolSwMZ3",
+ "colab": {
+ "base_uri": "https://localhost:8080/"
+ },
+ "outputId": "31435123-f99a-4c32-c374-93f38fc35e69"
+ },
+ "execution_count": null,
+ "outputs": [
+ {
+ "output_type": "stream",
+ "name": "stdout",
+ "text": [
+ "Using TensorFlow backend\n"
+ ]
+ }
+ ]
+ },
+ {
+ "cell_type": "code",
+ "source": [
+ "from google.colab import auth\n",
+ "\n",
+ "auth.authenticate_user()"
+ ],
+ "metadata": {
+ "id": "bZ_jp2X1PKM5"
+ },
+ "execution_count": null,
+ "outputs": []
+ },
+ {
+ "cell_type": "code",
+ "source": [
+ "try:\n",
+ " tpu = tf.distribute.cluster_resolver.TPUClusterResolver.connect()\n",
+ " strategy = tf.distribute.TPUStrategy(tpu)\n",
+ "except ValueError:\n",
+ " # MirroredStrategy is best for a single machine with one or multiple GPUs\n",
+ " strategy = tf.distribute.MirroredStrategy()\n",
+ "\n",
+ "BATCH_SIZE = 4\n",
+ "GLOBAL_BATCH_SIZE = BATCH_SIZE * strategy.num_replicas_in_sync\n",
+ "BASE_LR = 0.01 * GLOBAL_BATCH_SIZE / 64\n",
+ "print(\"Number of accelerators: \", strategy.num_replicas_in_sync)\n",
+ "print(\"Global Batch Size: \", GLOBAL_BATCH_SIZE)\n",
+ "\n",
+ "IMG_SIZE = 640\n",
+ "image_size = [IMG_SIZE, IMG_SIZE, 3]\n",
+ "\n",
+ "# data_dir=\"gs://kerascv-dataset\"\n",
+ "train_ds = tfds.load(\n",
+ " \"voc/2007\",\n",
+ " split=\"train+validation\",\n",
+ " with_info=False,\n",
+ " shuffle_files=True, # , data_dir=\"gs://kerascv-dataset\"\n",
+ ")\n",
+ "train_ds = train_ds.concatenate(\n",
+ " tfds.load(\n",
+ " \"voc/2012\",\n",
+ " split=\"train+validation\",\n",
+ " with_info=False,\n",
+ " shuffle_files=True,\n",
+ " # data_dir=\"gs://kerascv-dataset\"\n",
+ " )\n",
+ ")\n",
+ "eval_ds = tfds.load(\n",
+ " \"voc/2007\", split=\"test\", with_info=False\n",
+ ") # , data_dir=\"gs://kerascv-dataset\")\n",
+ "\n",
+ "\n",
+ "def unpackage_tfds_inputs(inputs, bounding_box_format):\n",
+ " image = inputs[\"image\"]\n",
+ " boxes = keras_cv.bounding_box.convert_format(\n",
+ " inputs[\"objects\"][\"bbox\"],\n",
+ " images=image,\n",
+ " source=\"rel_yxyx\",\n",
+ " target=bounding_box_format,\n",
+ " )\n",
+ " bounding_boxes = {\n",
+ " \"classes\": tf.cast(inputs[\"objects\"][\"label\"], dtype=tf.float32),\n",
+ " \"boxes\": tf.cast(boxes, dtype=tf.float32),\n",
+ " }\n",
+ " return {\n",
+ " \"images\": tf.cast(image, tf.float32),\n",
+ " \"bounding_boxes\": bounding_boxes,\n",
+ " }\n",
+ "\n",
+ "\n",
+ "train_ds = train_ds.map(\n",
+ " lambda inputs: unpackage_tfds_inputs(inputs, bounding_box_format=\"xywh\"),\n",
+ " num_parallel_calls=tf.data.AUTOTUNE,\n",
+ ")\n",
+ "eval_ds = eval_ds.map(\n",
+ " lambda inputs: unpackage_tfds_inputs(inputs, bounding_box_format=\"xywh\"),\n",
+ " num_parallel_calls=tf.data.AUTOTUNE,\n",
+ ")\n",
+ "\n",
+ "augmenter = keras.Sequential(\n",
+ " layers=[\n",
+ " keras_cv.layers.RandomFlip(\n",
+ " mode=\"horizontal\", bounding_box_format=\"xywh\"\n",
+ " ),\n",
+ " keras_cv.layers.JitteredResize(\n",
+ " target_size=(640, 640),\n",
+ " scale_factor=(0.8, 1.25),\n",
+ " bounding_box_format=\"xywh\",\n",
+ " ),\n",
+ " ]\n",
+ ")\n",
+ "train_ds = train_ds.apply(\n",
+ " tf.data.experimental.dense_to_ragged_batch(BATCH_SIZE)\n",
+ ")\n",
+ "train_ds = train_ds.map(augmenter, num_parallel_calls=tf.data.AUTOTUNE)\n",
+ "\n",
+ "\n",
+ "def pad_fn(inputs):\n",
+ " inputs[\"bounding_boxes\"] = keras_cv.bounding_box.to_dense(\n",
+ " inputs[\"bounding_boxes\"], max_boxes=32\n",
+ " )\n",
+ " return inputs\n",
+ "\n",
+ "\n",
+ "train_ds = train_ds.shuffle(8 * strategy.num_replicas_in_sync)\n",
+ "train_ds = train_ds.map(pad_fn, num_parallel_calls=tf.data.AUTOTUNE)\n",
+ "train_ds = train_ds.prefetch(tf.data.AUTOTUNE)\n",
+ "\n",
+ "eval_resizing = keras_cv.layers.Resizing(\n",
+ " 640, 640, pad_to_aspect_ratio=True, bounding_box_format=\"xywh\"\n",
+ ")\n",
+ "eval_ds = eval_ds.map(\n",
+ " eval_resizing,\n",
+ " num_parallel_calls=tf.data.AUTOTUNE,\n",
+ ")\n",
+ "eval_ds = eval_ds.apply(tf.data.experimental.dense_to_ragged_batch(BATCH_SIZE))\n",
+ "eval_ds = eval_ds.map(pad_fn, num_parallel_calls=tf.data.AUTOTUNE)\n",
+ "eval_ds = eval_ds.prefetch(tf.data.AUTOTUNE)"
+ ],
+ "metadata": {
+ "id": "96w4OHJgMseo",
+ "colab": {
+ "base_uri": "https://localhost:8080/"
+ },
+ "outputId": "b5a9d0f9-3730-4d5e-c6c3-47b8eaeae0e7"
+ },
+ "execution_count": null,
+ "outputs": [
+ {
+ "output_type": "stream",
+ "name": "stdout",
+ "text": [
+ "Number of accelerators: 1\n",
+ "Global Batch Size: 4\n"
+ ]
+ },
+ {
+ "output_type": "stream",
+ "name": "stderr",
+ "text": [
+ "WARNING:tensorflow:From :73: dense_to_ragged_batch (from tensorflow.python.data.experimental.ops.batching) is deprecated and will be removed in a future version.\n",
+ "Instructions for updating:\n",
+ "Use `tf.data.Dataset.ragged_batch` instead.\n",
+ "WARNING:tensorflow:Layers in a Sequential model should only have a single input tensor. Received: inputs={'images': tf.RaggedTensor(values=tf.RaggedTensor(values=Tensor(\"RaggedFromVariant_2/RaggedTensorFromVariant:2\", shape=(None, 3), dtype=float32), row_splits=Tensor(\"RaggedFromVariant_2/RaggedTensorFromVariant:1\", shape=(None,), dtype=int64)), row_splits=Tensor(\"RaggedFromVariant_2/RaggedTensorFromVariant:0\", shape=(None,), dtype=int64)), 'bounding_boxes': {'classes': tf.RaggedTensor(values=Tensor(\"RaggedFromVariant_1/RaggedTensorFromVariant:1\", shape=(None,), dtype=float32), row_splits=Tensor(\"RaggedFromVariant_1/RaggedTensorFromVariant:0\", shape=(None,), dtype=int64)), 'boxes': tf.RaggedTensor(values=Tensor(\"RaggedFromVariant/RaggedTensorFromVariant:1\", shape=(None, 4), dtype=float32), row_splits=Tensor(\"RaggedFromVariant/RaggedTensorFromVariant:0\", shape=(None,), dtype=int64))}}. Consider rewriting this model with the Functional API.\n"
+ ]
+ }
+ ]
+ },
+ {
+ "cell_type": "code",
+ "source": [
+ "with strategy.scope():\n",
+ " model = keras_cv.models.YOLOV8Detector(\n",
+ " num_classes=20,\n",
+ " backbone=keras_cv.models.YOLOV8Backbone.from_preset(\n",
+ " \"yolo_v8_m_backbone_coco\"\n",
+ " ),\n",
+ " fpn_depth=2,\n",
+ " bounding_box_format=\"xywh\",\n",
+ " )\n",
+ " lr_schedule = keras.optimizers.schedules.PolynomialDecay(\n",
+ " initial_learning_rate=BASE_LR,\n",
+ " decay_steps=train_ds.cardinality() * 120,\n",
+ " )\n",
+ " optimizer = tf.keras.optimizers.SGD(\n",
+ " learning_rate=lr_schedule,\n",
+ " momentum=0.937,\n",
+ " clipnorm=5.0,\n",
+ " weight_decay=5e-4,\n",
+ " use_ema=True,\n",
+ " ema_momentum=0.9999,\n",
+ " )\n",
+ "\n",
+ "model.compile(\n",
+ " optimizer=optimizer,\n",
+ " box_loss=\"ciou\",\n",
+ " classification_loss=\"binary_crossentropy\",\n",
+ ")\n",
+ "model.backbone.trainable = True\n",
+ "\n",
+ "callbacks = [\n",
+ " keras_cv.callbacks.PyCOCOCallback(eval_ds, bounding_box_format=\"xywh\"),\n",
+ " keras.callbacks.TensorBoard(\"gs://ian-kerascv/yolov8-gpu-logs-v4\"),\n",
+ " keras.callbacks.ModelCheckpoint(\n",
+ " \"./weights.h5\", save_best_only=True, save_weights_only=True\n",
+ " ),\n",
+ "]\n",
+ "\n",
+ "history = model.fit(\n",
+ " train_ds,\n",
+ " validation_data=eval_ds,\n",
+ " epochs=120,\n",
+ " callbacks=callbacks,\n",
+ ")"
+ ],
+ "metadata": {
+ "colab": {
+ "base_uri": "https://localhost:8080/"
+ },
+ "id": "3kQ4z0AwMyEi",
+ "outputId": "e8131d4a-c12f-438b-8642-7bce0abeee7f"
+ },
+ "execution_count": null,
+ "outputs": [
+ {
+ "metadata": {
+ "tags": null
+ },
+ "name": "stdout",
+ "output_type": "stream",
+ "text": [
+ "Epoch 1/120\n",
+ " 6/4138 [..............................] - ETA: 7:32 - loss: 543.9800 - box_loss: 2.9202 - class_loss: 541.0598"
+ ]
+ },
+ {
+ "metadata": {
+ "tags": null
+ },
+ "name": "stderr",
+ "output_type": "stream",
+ "text": [
+ "WARNING:tensorflow:Callback method `on_train_batch_end` is slow compared to the batch time (batch time: 0.1021s vs `on_train_batch_end` time: 0.3079s). Check your callbacks.\n"
+ ]
+ },
+ {
+ "metadata": {
+ "tags": null
+ },
+ "name": "stdout",
+ "output_type": "stream",
+ "text": [
+ "1238/1238 [==============================] - 131s 101ms/step\n",
+ "creating index...\n",
+ "index created!\n",
+ "creating index...\n",
+ "index created!\n",
+ "Running per image evaluation...\n",
+ "Evaluate annotation type *bbox*\n",
+ "DONE (t=0.04s).\n",
+ "Accumulating evaluation results...\n",
+ "DONE (t=0.06s).\n",
+ " Average Precision (AP) @[ IoU=0.50:0.95 | area= all | maxDets=100 ] = 0.005\n",
+ " Average Precision (AP) @[ IoU=0.50 | area= all | maxDets=100 ] = 0.010\n",
+ " Average Precision (AP) @[ IoU=0.75 | area= all | maxDets=100 ] = 0.004\n",
+ " Average Precision (AP) @[ IoU=0.50:0.95 | area= small | maxDets=100 ] = 0.000\n",
+ " Average Precision (AP) @[ IoU=0.50:0.95 | area=medium | maxDets=100 ] = 0.000\n",
+ " Average Precision (AP) @[ IoU=0.50:0.95 | area= large | maxDets=100 ] = 0.006\n",
+ " Average Recall (AR) @[ IoU=0.50:0.95 | area= all | maxDets= 1 ] = 0.008\n",
+ " Average Recall (AR) @[ IoU=0.50:0.95 | area= all | maxDets= 10 ] = 0.009\n",
+ " Average Recall (AR) @[ IoU=0.50:0.95 | area= all | maxDets=100 ] = 0.009\n",
+ " Average Recall (AR) @[ IoU=0.50:0.95 | area= small | maxDets=100 ] = 0.000\n",
+ " Average Recall (AR) @[ IoU=0.50:0.95 | area=medium | maxDets=100 ] = 0.000\n",
+ " Average Recall (AR) @[ IoU=0.50:0.95 | area= large | maxDets=100 ] = 0.012\n",
+ "4138/4138 [==============================] - 691s 151ms/step - loss: 6.6185 - box_loss: 2.2628 - class_loss: 4.3558 - val_loss: 2.1278 - val_box_loss: 1.9264 - val_class_loss: 0.2014 - val_AP: 0.0047 - val_AP50: 0.0099 - val_AP75: 0.0042 - val_APs: 0.0000e+00 - val_APm: 0.0000e+00 - val_APl: 0.0065 - val_ARmax1: 0.0075 - val_ARmax10: 0.0085 - val_ARmax100: 0.0085 - val_ARs: 0.0000e+00 - val_ARm: 0.0000e+00 - val_ARl: 0.0120\n",
+ "Epoch 2/120\n",
+ "1238/1238 [==============================] - 111s 89ms/step\n",
+ "creating index...\n",
+ "index created!\n",
+ "creating index...\n",
+ "index created!\n",
+ "Running per image evaluation...\n",
+ "Evaluate annotation type *bbox*\n",
+ "DONE (t=0.89s).\n",
+ "Accumulating evaluation results...\n",
+ "DONE (t=0.27s).\n",
+ " Average Precision (AP) @[ IoU=0.50:0.95 | area= all | maxDets=100 ] = 0.010\n",
+ " Average Precision (AP) @[ IoU=0.50 | area= all | maxDets=100 ] = 0.021\n",
+ " Average Precision (AP) @[ IoU=0.75 | area= all | maxDets=100 ] = 0.009\n",
+ " Average Precision (AP) @[ IoU=0.50:0.95 | area= small | maxDets=100 ] = 0.004\n",
+ " Average Precision (AP) @[ IoU=0.50:0.95 | area=medium | maxDets=100 ] = 0.005\n",
+ " Average Precision (AP) @[ IoU=0.50:0.95 | area= large | maxDets=100 ] = 0.015\n",
+ " Average Recall (AR) @[ IoU=0.50:0.95 | area= all | maxDets= 1 ] = 0.010\n",
+ " Average Recall (AR) @[ IoU=0.50:0.95 | area= all | maxDets= 10 ] = 0.014\n",
+ " Average Recall (AR) @[ IoU=0.50:0.95 | area= all | maxDets=100 ] = 0.014\n",
+ " Average Recall (AR) @[ IoU=0.50:0.95 | area= small | maxDets=100 ] = 0.004\n",
+ " Average Recall (AR) @[ IoU=0.50:0.95 | area=medium | maxDets=100 ] = 0.008\n",
+ " Average Recall (AR) @[ IoU=0.50:0.95 | area= large | maxDets=100 ] = 0.020\n",
+ "4138/4138 [==============================] - 602s 145ms/step - loss: 1.9417 - box_loss: 1.7593 - class_loss: 0.1824 - val_loss: 1.8599 - val_box_loss: 1.6905 - val_class_loss: 0.1694 - val_AP: 0.0103 - val_AP50: 0.0209 - val_AP75: 0.0091 - val_APs: 0.0037 - val_APm: 0.0054 - val_APl: 0.0146 - val_ARmax1: 0.0100 - val_ARmax10: 0.0142 - val_ARmax100: 0.0142 - val_ARs: 0.0043 - val_ARm: 0.0079 - val_ARl: 0.0196\n",
+ "Epoch 3/120\n",
+ "1238/1238 [==============================] - 110s 89ms/step\n",
+ "creating index...\n",
+ "index created!\n",
+ "creating index...\n",
+ "index created!\n",
+ "Running per image evaluation...\n",
+ "Evaluate annotation type *bbox*\n",
+ "DONE (t=2.04s).\n",
+ "Accumulating evaluation results...\n",
+ "DONE (t=0.42s).\n",
+ " Average Precision (AP) @[ IoU=0.50:0.95 | area= all | maxDets=100 ] = 0.018\n",
+ " Average Precision (AP) @[ IoU=0.50 | area= all | maxDets=100 ] = 0.033\n",
+ " Average Precision (AP) @[ IoU=0.75 | area= all | maxDets=100 ] = 0.018\n",
+ " Average Precision (AP) @[ IoU=0.50:0.95 | area= small | maxDets=100 ] = 0.005\n",
+ " Average Precision (AP) @[ IoU=0.50:0.95 | area=medium | maxDets=100 ] = 0.012\n",
+ " Average Precision (AP) @[ IoU=0.50:0.95 | area= large | maxDets=100 ] = 0.024\n",
+ " Average Recall (AR) @[ IoU=0.50:0.95 | area= all | maxDets= 1 ] = 0.024\n",
+ " Average Recall (AR) @[ IoU=0.50:0.95 | area= all | maxDets= 10 ] = 0.032\n",
+ " Average Recall (AR) @[ IoU=0.50:0.95 | area= all | maxDets=100 ] = 0.032\n",
+ " Average Recall (AR) @[ IoU=0.50:0.95 | area= small | maxDets=100 ] = 0.007\n",
+ " Average Recall (AR) @[ IoU=0.50:0.95 | area=medium | maxDets=100 ] = 0.018\n",
+ " Average Recall (AR) @[ IoU=0.50:0.95 | area= large | maxDets=100 ] = 0.039\n",
+ "4138/4138 [==============================] - 598s 144ms/step - loss: 1.7546 - box_loss: 1.5924 - class_loss: 0.1622 - val_loss: 1.7456 - val_box_loss: 1.5875 - val_class_loss: 0.1581 - val_AP: 0.0181 - val_AP50: 0.0327 - val_AP75: 0.0175 - val_APs: 0.0052 - val_APm: 0.0123 - val_APl: 0.0238 - val_ARmax1: 0.0245 - val_ARmax10: 0.0320 - val_ARmax100: 0.0320 - val_ARs: 0.0070 - val_ARm: 0.0183 - val_ARl: 0.0391\n",
+ "Epoch 4/120\n",
+ "1238/1238 [==============================] - 110s 89ms/step\n",
+ "creating index...\n",
+ "index created!\n",
+ "creating index...\n",
+ "index created!\n",
+ "Running per image evaluation...\n",
+ "Evaluate annotation type *bbox*\n",
+ "DONE (t=2.55s).\n",
+ "Accumulating evaluation results...\n",
+ "DONE (t=0.54s).\n",
+ " Average Precision (AP) @[ IoU=0.50:0.95 | area= all | maxDets=100 ] = 0.022\n",
+ " Average Precision (AP) @[ IoU=0.50 | area= all | maxDets=100 ] = 0.039\n",
+ " Average Precision (AP) @[ IoU=0.75 | area= all | maxDets=100 ] = 0.021\n",
+ " Average Precision (AP) @[ IoU=0.50:0.95 | area= small | maxDets=100 ] = 0.004\n",
+ " Average Precision (AP) @[ IoU=0.50:0.95 | area=medium | maxDets=100 ] = 0.013\n",
+ " Average Precision (AP) @[ IoU=0.50:0.95 | area= large | maxDets=100 ] = 0.029\n",
+ " Average Recall (AR) @[ IoU=0.50:0.95 | area= all | maxDets= 1 ] = 0.030\n",
+ " Average Recall (AR) @[ IoU=0.50:0.95 | area= all | maxDets= 10 ] = 0.040\n",
+ " Average Recall (AR) @[ IoU=0.50:0.95 | area= all | maxDets=100 ] = 0.040\n",
+ " Average Recall (AR) @[ IoU=0.50:0.95 | area= small | maxDets=100 ] = 0.005\n",
+ " Average Recall (AR) @[ IoU=0.50:0.95 | area=medium | maxDets=100 ] = 0.019\n",
+ " Average Recall (AR) @[ IoU=0.50:0.95 | area= large | maxDets=100 ] = 0.050\n",
+ "4138/4138 [==============================] - 600s 145ms/step - loss: 1.6476 - box_loss: 1.4961 - class_loss: 0.1515 - val_loss: 1.6936 - val_box_loss: 1.5437 - val_class_loss: 0.1498 - val_AP: 0.0215 - val_AP50: 0.0388 - val_AP75: 0.0210 - val_APs: 0.0045 - val_APm: 0.0125 - val_APl: 0.0286 - val_ARmax1: 0.0298 - val_ARmax10: 0.0399 - val_ARmax100: 0.0402 - val_ARs: 0.0054 - val_ARm: 0.0191 - val_ARl: 0.0502\n",
+ "Epoch 5/120\n",
+ "1238/1238 [==============================] - 111s 89ms/step\n",
+ "creating index...\n",
+ "index created!\n",
+ "creating index...\n",
+ "index created!\n",
+ "Running per image evaluation...\n",
+ "Evaluate annotation type *bbox*\n",
+ "DONE (t=2.24s).\n",
+ "Accumulating evaluation results...\n",
+ "DONE (t=0.59s).\n",
+ " Average Precision (AP) @[ IoU=0.50:0.95 | area= all | maxDets=100 ] = 0.029\n",
+ " Average Precision (AP) @[ IoU=0.50 | area= all | maxDets=100 ] = 0.050\n",
+ " Average Precision (AP) @[ IoU=0.75 | area= all | maxDets=100 ] = 0.031\n",
+ " Average Precision (AP) @[ IoU=0.50:0.95 | area= small | maxDets=100 ] = 0.006\n",
+ " Average Precision (AP) @[ IoU=0.50:0.95 | area=medium | maxDets=100 ] = 0.020\n",
+ " Average Precision (AP) @[ IoU=0.50:0.95 | area= large | maxDets=100 ] = 0.036\n",
+ " Average Recall (AR) @[ IoU=0.50:0.95 | area= all | maxDets= 1 ] = 0.047\n",
+ " Average Recall (AR) @[ IoU=0.50:0.95 | area= all | maxDets= 10 ] = 0.060\n",
+ " Average Recall (AR) @[ IoU=0.50:0.95 | area= all | maxDets=100 ] = 0.061\n",
+ " Average Recall (AR) @[ IoU=0.50:0.95 | area= small | maxDets=100 ] = 0.010\n",
+ " Average Recall (AR) @[ IoU=0.50:0.95 | area=medium | maxDets=100 ] = 0.028\n",
+ " Average Recall (AR) @[ IoU=0.50:0.95 | area= large | maxDets=100 ] = 0.070\n",
+ "4138/4138 [==============================] - 599s 145ms/step - loss: 1.5638 - box_loss: 1.4202 - class_loss: 0.1436 - val_loss: 1.6210 - val_box_loss: 1.4787 - val_class_loss: 0.1422 - val_AP: 0.0291 - val_AP50: 0.0499 - val_AP75: 0.0308 - val_APs: 0.0062 - val_APm: 0.0197 - val_APl: 0.0355 - val_ARmax1: 0.0471 - val_ARmax10: 0.0603 - val_ARmax100: 0.0606 - val_ARs: 0.0104 - val_ARm: 0.0280 - val_ARl: 0.0703\n",
+ "Epoch 6/120\n",
+ "1238/1238 [==============================] - 111s 89ms/step\n",
+ "creating index...\n",
+ "index created!\n",
+ "creating index...\n",
+ "index created!\n",
+ "Running per image evaluation...\n",
+ "Evaluate annotation type *bbox*\n",
+ "DONE (t=2.84s).\n",
+ "Accumulating evaluation results...\n",
+ "DONE (t=0.61s).\n",
+ " Average Precision (AP) @[ IoU=0.50:0.95 | area= all | maxDets=100 ] = 0.042\n",
+ " Average Precision (AP) @[ IoU=0.50 | area= all | maxDets=100 ] = 0.068\n",
+ " Average Precision (AP) @[ IoU=0.75 | area= all | maxDets=100 ] = 0.044\n",
+ " Average Precision (AP) @[ IoU=0.50:0.95 | area= small | maxDets=100 ] = 0.009\n",
+ " Average Precision (AP) @[ IoU=0.50:0.95 | area=medium | maxDets=100 ] = 0.024\n",
+ " Average Precision (AP) @[ IoU=0.50:0.95 | area= large | maxDets=100 ] = 0.052\n",
+ " Average Recall (AR) @[ IoU=0.50:0.95 | area= all | maxDets= 1 ] = 0.061\n",
+ " Average Recall (AR) @[ IoU=0.50:0.95 | area= all | maxDets= 10 ] = 0.077\n",
+ " Average Recall (AR) @[ IoU=0.50:0.95 | area= all | maxDets=100 ] = 0.077\n",
+ " Average Recall (AR) @[ IoU=0.50:0.95 | area= small | maxDets=100 ] = 0.015\n",
+ " Average Recall (AR) @[ IoU=0.50:0.95 | area=medium | maxDets=100 ] = 0.037\n",
+ " Average Recall (AR) @[ IoU=0.50:0.95 | area= large | maxDets=100 ] = 0.090\n",
+ "4138/4138 [==============================] - 600s 145ms/step - loss: 1.5040 - box_loss: 1.3672 - class_loss: 0.1368 - val_loss: 1.5693 - val_box_loss: 1.4325 - val_class_loss: 0.1368 - val_AP: 0.0419 - val_AP50: 0.0681 - val_AP75: 0.0437 - val_APs: 0.0093 - val_APm: 0.0240 - val_APl: 0.0522 - val_ARmax1: 0.0608 - val_ARmax10: 0.0770 - val_ARmax100: 0.0773 - val_ARs: 0.0149 - val_ARm: 0.0368 - val_ARl: 0.0904\n",
+ "Epoch 7/120\n",
+ "1238/1238 [==============================] - 112s 90ms/step\n",
+ "creating index...\n",
+ "index created!\n",
+ "creating index...\n",
+ "index created!\n",
+ "Running per image evaluation...\n",
+ "Evaluate annotation type *bbox*\n",
+ "DONE (t=2.87s).\n",
+ "Accumulating evaluation results...\n",
+ "DONE (t=0.61s).\n",
+ " Average Precision (AP) @[ IoU=0.50:0.95 | area= all | maxDets=100 ] = 0.046\n",
+ " Average Precision (AP) @[ IoU=0.50 | area= all | maxDets=100 ] = 0.074\n",
+ " Average Precision (AP) @[ IoU=0.75 | area= all | maxDets=100 ] = 0.050\n",
+ " Average Precision (AP) @[ IoU=0.50:0.95 | area= small | maxDets=100 ] = 0.007\n",
+ " Average Precision (AP) @[ IoU=0.50:0.95 | area=medium | maxDets=100 ] = 0.024\n",
+ " Average Precision (AP) @[ IoU=0.50:0.95 | area= large | maxDets=100 ] = 0.056\n",
+ " Average Recall (AR) @[ IoU=0.50:0.95 | area= all | maxDets= 1 ] = 0.073\n",
+ " Average Recall (AR) @[ IoU=0.50:0.95 | area= all | maxDets= 10 ] = 0.089\n",
+ " Average Recall (AR) @[ IoU=0.50:0.95 | area= all | maxDets=100 ] = 0.089\n",
+ " Average Recall (AR) @[ IoU=0.50:0.95 | area= small | maxDets=100 ] = 0.012\n",
+ " Average Recall (AR) @[ IoU=0.50:0.95 | area=medium | maxDets=100 ] = 0.036\n",
+ " Average Recall (AR) @[ IoU=0.50:0.95 | area= large | maxDets=100 ] = 0.102\n",
+ "4138/4138 [==============================] - 605s 146ms/step - loss: 1.4514 - box_loss: 1.3209 - class_loss: 0.1305 - val_loss: 1.5627 - val_box_loss: 1.4287 - val_class_loss: 0.1340 - val_AP: 0.0463 - val_AP50: 0.0745 - val_AP75: 0.0501 - val_APs: 0.0068 - val_APm: 0.0244 - val_APl: 0.0561 - val_ARmax1: 0.0731 - val_ARmax10: 0.0886 - val_ARmax100: 0.0888 - val_ARs: 0.0122 - val_ARm: 0.0364 - val_ARl: 0.1023\n",
+ "Epoch 8/120\n",
+ "1238/1238 [==============================] - 112s 90ms/step\n",
+ "creating index...\n",
+ "index created!\n",
+ "creating index...\n",
+ "index created!\n",
+ "Running per image evaluation...\n",
+ "Evaluate annotation type *bbox*\n",
+ "DONE (t=3.06s).\n",
+ "Accumulating evaluation results...\n",
+ "DONE (t=0.65s).\n",
+ " Average Precision (AP) @[ IoU=0.50:0.95 | area= all | maxDets=100 ] = 0.065\n",
+ " Average Precision (AP) @[ IoU=0.50 | area= all | maxDets=100 ] = 0.102\n",
+ " Average Precision (AP) @[ IoU=0.75 | area= all | maxDets=100 ] = 0.070\n",
+ " Average Precision (AP) @[ IoU=0.50:0.95 | area= small | maxDets=100 ] = 0.011\n",
+ " Average Precision (AP) @[ IoU=0.50:0.95 | area=medium | maxDets=100 ] = 0.034\n",
+ " Average Precision (AP) @[ IoU=0.50:0.95 | area= large | maxDets=100 ] = 0.077\n",
+ " Average Recall (AR) @[ IoU=0.50:0.95 | area= all | maxDets= 1 ] = 0.094\n",
+ " Average Recall (AR) @[ IoU=0.50:0.95 | area= all | maxDets= 10 ] = 0.114\n",
+ " Average Recall (AR) @[ IoU=0.50:0.95 | area= all | maxDets=100 ] = 0.115\n",
+ " Average Recall (AR) @[ IoU=0.50:0.95 | area= small | maxDets=100 ] = 0.015\n",
+ " Average Recall (AR) @[ IoU=0.50:0.95 | area=medium | maxDets=100 ] = 0.052\n",
+ " Average Recall (AR) @[ IoU=0.50:0.95 | area= large | maxDets=100 ] = 0.129\n",
+ "4138/4138 [==============================] - 606s 146ms/step - loss: 1.4050 - box_loss: 1.2801 - class_loss: 0.1249 - val_loss: 1.5070 - val_box_loss: 1.3815 - val_class_loss: 0.1255 - val_AP: 0.0653 - val_AP50: 0.1017 - val_AP75: 0.0695 - val_APs: 0.0106 - val_APm: 0.0342 - val_APl: 0.0768 - val_ARmax1: 0.0936 - val_ARmax10: 0.1144 - val_ARmax100: 0.1146 - val_ARs: 0.0149 - val_ARm: 0.0523 - val_ARl: 0.1287\n",
+ "Epoch 9/120\n",
+ "1238/1238 [==============================] - 111s 90ms/step\n",
+ "creating index...\n",
+ "index created!\n",
+ "creating index...\n",
+ "index created!\n",
+ "Running per image evaluation...\n",
+ "Evaluate annotation type *bbox*\n",
+ "DONE (t=3.57s).\n",
+ "Accumulating evaluation results...\n",
+ "DONE (t=0.77s).\n",
+ " Average Precision (AP) @[ IoU=0.50:0.95 | area= all | maxDets=100 ] = 0.072\n",
+ " Average Precision (AP) @[ IoU=0.50 | area= all | maxDets=100 ] = 0.115\n",
+ " Average Precision (AP) @[ IoU=0.75 | area= all | maxDets=100 ] = 0.076\n",
+ " Average Precision (AP) @[ IoU=0.50:0.95 | area= small | maxDets=100 ] = 0.012\n",
+ " Average Precision (AP) @[ IoU=0.50:0.95 | area=medium | maxDets=100 ] = 0.044\n",
+ " Average Precision (AP) @[ IoU=0.50:0.95 | area= large | maxDets=100 ] = 0.084\n",
+ " Average Recall (AR) @[ IoU=0.50:0.95 | area= all | maxDets= 1 ] = 0.105\n",
+ " Average Recall (AR) @[ IoU=0.50:0.95 | area= all | maxDets= 10 ] = 0.132\n",
+ " Average Recall (AR) @[ IoU=0.50:0.95 | area= all | maxDets=100 ] = 0.132\n",
+ " Average Recall (AR) @[ IoU=0.50:0.95 | area= small | maxDets=100 ] = 0.018\n",
+ " Average Recall (AR) @[ IoU=0.50:0.95 | area=medium | maxDets=100 ] = 0.066\n",
+ " Average Recall (AR) @[ IoU=0.50:0.95 | area= large | maxDets=100 ] = 0.144\n",
+ "4138/4138 [==============================] - 606s 146ms/step - loss: 1.3625 - box_loss: 1.2422 - class_loss: 0.1203 - val_loss: 1.4845 - val_box_loss: 1.3633 - val_class_loss: 0.1212 - val_AP: 0.0721 - val_AP50: 0.1148 - val_AP75: 0.0762 - val_APs: 0.0118 - val_APm: 0.0445 - val_APl: 0.0836 - val_ARmax1: 0.1051 - val_ARmax10: 0.1315 - val_ARmax100: 0.1322 - val_ARs: 0.0185 - val_ARm: 0.0664 - val_ARl: 0.1444\n",
+ "Epoch 10/120\n",
+ "1238/1238 [==============================] - 112s 90ms/step\n",
+ "creating index...\n",
+ "index created!\n",
+ "creating index...\n",
+ "index created!\n",
+ "Running per image evaluation...\n",
+ "Evaluate annotation type *bbox*\n",
+ "DONE (t=3.61s).\n",
+ "Accumulating evaluation results...\n",
+ "DONE (t=0.78s).\n",
+ " Average Precision (AP) @[ IoU=0.50:0.95 | area= all | maxDets=100 ] = 0.082\n",
+ " Average Precision (AP) @[ IoU=0.50 | area= all | maxDets=100 ] = 0.129\n",
+ " Average Precision (AP) @[ IoU=0.75 | area= all | maxDets=100 ] = 0.088\n",
+ " Average Precision (AP) @[ IoU=0.50:0.95 | area= small | maxDets=100 ] = 0.008\n",
+ " Average Precision (AP) @[ IoU=0.50:0.95 | area=medium | maxDets=100 ] = 0.045\n",
+ " Average Precision (AP) @[ IoU=0.50:0.95 | area= large | maxDets=100 ] = 0.094\n",
+ " Average Recall (AR) @[ IoU=0.50:0.95 | area= all | maxDets= 1 ] = 0.117\n",
+ " Average Recall (AR) @[ IoU=0.50:0.95 | area= all | maxDets= 10 ] = 0.144\n",
+ " Average Recall (AR) @[ IoU=0.50:0.95 | area= all | maxDets=100 ] = 0.144\n",
+ " Average Recall (AR) @[ IoU=0.50:0.95 | area= small | maxDets=100 ] = 0.013\n",
+ " Average Recall (AR) @[ IoU=0.50:0.95 | area=medium | maxDets=100 ] = 0.072\n",
+ " Average Recall (AR) @[ IoU=0.50:0.95 | area= large | maxDets=100 ] = 0.157\n",
+ "4138/4138 [==============================] - 607s 146ms/step - loss: 1.3286 - box_loss: 1.2130 - class_loss: 0.1156 - val_loss: 1.4917 - val_box_loss: 1.3721 - val_class_loss: 0.1197 - val_AP: 0.0815 - val_AP50: 0.1286 - val_AP75: 0.0876 - val_APs: 0.0083 - val_APm: 0.0448 - val_APl: 0.0937 - val_ARmax1: 0.1170 - val_ARmax10: 0.1436 - val_ARmax100: 0.1445 - val_ARs: 0.0134 - val_ARm: 0.0724 - val_ARl: 0.1566\n",
+ "Epoch 11/120\n",
+ "1238/1238 [==============================] - 112s 90ms/step\n",
+ "creating index...\n",
+ "index created!\n",
+ "creating index...\n",
+ "index created!\n",
+ "Running per image evaluation...\n",
+ "Evaluate annotation type *bbox*\n",
+ "DONE (t=3.60s).\n",
+ "Accumulating evaluation results...\n",
+ "DONE (t=1.40s).\n",
+ " Average Precision (AP) @[ IoU=0.50:0.95 | area= all | maxDets=100 ] = 0.105\n",
+ " Average Precision (AP) @[ IoU=0.50 | area= all | maxDets=100 ] = 0.162\n",
+ " Average Precision (AP) @[ IoU=0.75 | area= all | maxDets=100 ] = 0.112\n",
+ " Average Precision (AP) @[ IoU=0.50:0.95 | area= small | maxDets=100 ] = 0.012\n",
+ " Average Precision (AP) @[ IoU=0.50:0.95 | area=medium | maxDets=100 ] = 0.052\n",
+ " Average Precision (AP) @[ IoU=0.50:0.95 | area= large | maxDets=100 ] = 0.122\n",
+ " Average Recall (AR) @[ IoU=0.50:0.95 | area= all | maxDets= 1 ] = 0.143\n",
+ " Average Recall (AR) @[ IoU=0.50:0.95 | area= all | maxDets= 10 ] = 0.172\n",
+ " Average Recall (AR) @[ IoU=0.50:0.95 | area= all | maxDets=100 ] = 0.173\n",
+ " Average Recall (AR) @[ IoU=0.50:0.95 | area= small | maxDets=100 ] = 0.020\n",
+ " Average Recall (AR) @[ IoU=0.50:0.95 | area=medium | maxDets=100 ] = 0.080\n",
+ " Average Recall (AR) @[ IoU=0.50:0.95 | area= large | maxDets=100 ] = 0.191\n",
+ "4138/4138 [==============================] - 609s 147ms/step - loss: 1.2937 - box_loss: 1.1823 - class_loss: 0.1115 - val_loss: 1.4541 - val_box_loss: 1.3397 - val_class_loss: 0.1145 - val_AP: 0.1046 - val_AP50: 0.1617 - val_AP75: 0.1123 - val_APs: 0.0123 - val_APm: 0.0518 - val_APl: 0.1221 - val_ARmax1: 0.1429 - val_ARmax10: 0.1721 - val_ARmax100: 0.1732 - val_ARs: 0.0201 - val_ARm: 0.0803 - val_ARl: 0.1909\n",
+ "Epoch 12/120\n",
+ "1238/1238 [==============================] - 111s 90ms/step\n",
+ "creating index...\n",
+ "index created!\n",
+ "creating index...\n",
+ "index created!\n",
+ "Running per image evaluation...\n",
+ "Evaluate annotation type *bbox*\n",
+ "DONE (t=3.85s).\n",
+ "Accumulating evaluation results...\n",
+ "DONE (t=0.83s).\n",
+ " Average Precision (AP) @[ IoU=0.50:0.95 | area= all | maxDets=100 ] = 0.113\n",
+ " Average Precision (AP) @[ IoU=0.50 | area= all | maxDets=100 ] = 0.175\n",
+ " Average Precision (AP) @[ IoU=0.75 | area= all | maxDets=100 ] = 0.121\n",
+ " Average Precision (AP) @[ IoU=0.50:0.95 | area= small | maxDets=100 ] = 0.016\n",
+ " Average Precision (AP) @[ IoU=0.50:0.95 | area=medium | maxDets=100 ] = 0.057\n",
+ " Average Precision (AP) @[ IoU=0.50:0.95 | area= large | maxDets=100 ] = 0.133\n",
+ " Average Recall (AR) @[ IoU=0.50:0.95 | area= all | maxDets= 1 ] = 0.155\n",
+ " Average Recall (AR) @[ IoU=0.50:0.95 | area= all | maxDets= 10 ] = 0.188\n",
+ " Average Recall (AR) @[ IoU=0.50:0.95 | area= all | maxDets=100 ] = 0.190\n",
+ " Average Recall (AR) @[ IoU=0.50:0.95 | area= small | maxDets=100 ] = 0.027\n",
+ " Average Recall (AR) @[ IoU=0.50:0.95 | area=medium | maxDets=100 ] = 0.090\n",
+ " Average Recall (AR) @[ IoU=0.50:0.95 | area= large | maxDets=100 ] = 0.209\n",
+ "4138/4138 [==============================] - 609s 147ms/step - loss: 1.2626 - box_loss: 1.1547 - class_loss: 0.1079 - val_loss: 1.4111 - val_box_loss: 1.3020 - val_class_loss: 0.1091 - val_AP: 0.1134 - val_AP50: 0.1750 - val_AP75: 0.1213 - val_APs: 0.0162 - val_APm: 0.0569 - val_APl: 0.1331 - val_ARmax1: 0.1548 - val_ARmax10: 0.1882 - val_ARmax100: 0.1899 - val_ARs: 0.0272 - val_ARm: 0.0898 - val_ARl: 0.2093\n",
+ "Epoch 13/120\n",
+ "1238/1238 [==============================] - 111s 90ms/step\n",
+ "creating index...\n",
+ "index created!\n",
+ "creating index...\n",
+ "index created!\n",
+ "Running per image evaluation...\n",
+ "Evaluate annotation type *bbox*\n",
+ "DONE (t=3.99s).\n",
+ "Accumulating evaluation results...\n",
+ "DONE (t=0.88s).\n",
+ " Average Precision (AP) @[ IoU=0.50:0.95 | area= all | maxDets=100 ] = 0.124\n",
+ " Average Precision (AP) @[ IoU=0.50 | area= all | maxDets=100 ] = 0.195\n",
+ " Average Precision (AP) @[ IoU=0.75 | area= all | maxDets=100 ] = 0.133\n",
+ " Average Precision (AP) @[ IoU=0.50:0.95 | area= small | maxDets=100 ] = 0.014\n",
+ " Average Precision (AP) @[ IoU=0.50:0.95 | area=medium | maxDets=100 ] = 0.065\n",
+ " Average Precision (AP) @[ IoU=0.50:0.95 | area= large | maxDets=100 ] = 0.146\n",
+ " Average Recall (AR) @[ IoU=0.50:0.95 | area= all | maxDets= 1 ] = 0.165\n",
+ " Average Recall (AR) @[ IoU=0.50:0.95 | area= all | maxDets= 10 ] = 0.206\n",
+ " Average Recall (AR) @[ IoU=0.50:0.95 | area= all | maxDets=100 ] = 0.208\n",
+ " Average Recall (AR) @[ IoU=0.50:0.95 | area= small | maxDets=100 ] = 0.023\n",
+ " Average Recall (AR) @[ IoU=0.50:0.95 | area=medium | maxDets=100 ] = 0.103\n",
+ " Average Recall (AR) @[ IoU=0.50:0.95 | area= large | maxDets=100 ] = 0.230\n",
+ "4138/4138 [==============================] - 607s 146ms/step - loss: 1.2352 - box_loss: 1.1307 - class_loss: 0.1045 - val_loss: 1.4255 - val_box_loss: 1.3185 - val_class_loss: 0.1070 - val_AP: 0.1244 - val_AP50: 0.1953 - val_AP75: 0.1332 - val_APs: 0.0138 - val_APm: 0.0646 - val_APl: 0.1459 - val_ARmax1: 0.1654 - val_ARmax10: 0.2064 - val_ARmax100: 0.2077 - val_ARs: 0.0229 - val_ARm: 0.1026 - val_ARl: 0.2300\n",
+ "Epoch 14/120\n",
+ "1238/1238 [==============================] - 112s 90ms/step\n",
+ "creating index...\n",
+ "index created!\n",
+ "creating index...\n",
+ "index created!\n",
+ "Running per image evaluation...\n",
+ "Evaluate annotation type *bbox*\n",
+ "DONE (t=3.86s).\n",
+ "Accumulating evaluation results...\n",
+ "DONE (t=0.83s).\n",
+ " Average Precision (AP) @[ IoU=0.50:0.95 | area= all | maxDets=100 ] = 0.132\n",
+ " Average Precision (AP) @[ IoU=0.50 | area= all | maxDets=100 ] = 0.203\n",
+ " Average Precision (AP) @[ IoU=0.75 | area= all | maxDets=100 ] = 0.142\n",
+ " Average Precision (AP) @[ IoU=0.50:0.95 | area= small | maxDets=100 ] = 0.013\n",
+ " Average Precision (AP) @[ IoU=0.50:0.95 | area=medium | maxDets=100 ] = 0.062\n",
+ " Average Precision (AP) @[ IoU=0.50:0.95 | area= large | maxDets=100 ] = 0.156\n",
+ " Average Recall (AR) @[ IoU=0.50:0.95 | area= all | maxDets= 1 ] = 0.178\n",
+ " Average Recall (AR) @[ IoU=0.50:0.95 | area= all | maxDets= 10 ] = 0.216\n",
+ " Average Recall (AR) @[ IoU=0.50:0.95 | area= all | maxDets=100 ] = 0.218\n",
+ " Average Recall (AR) @[ IoU=0.50:0.95 | area= small | maxDets=100 ] = 0.023\n",
+ " Average Recall (AR) @[ IoU=0.50:0.95 | area=medium | maxDets=100 ] = 0.096\n",
+ " Average Recall (AR) @[ IoU=0.50:0.95 | area= large | maxDets=100 ] = 0.243\n",
+ "4138/4138 [==============================] - 608s 147ms/step - loss: 1.2154 - box_loss: 1.1132 - class_loss: 0.1022 - val_loss: 1.4007 - val_box_loss: 1.2956 - val_class_loss: 0.1051 - val_AP: 0.1324 - val_AP50: 0.2034 - val_AP75: 0.1419 - val_APs: 0.0131 - val_APm: 0.0619 - val_APl: 0.1560 - val_ARmax1: 0.1782 - val_ARmax10: 0.2162 - val_ARmax100: 0.2178 - val_ARs: 0.0225 - val_ARm: 0.0956 - val_ARl: 0.2432\n",
+ "Epoch 15/120\n",
+ "1238/1238 [==============================] - 111s 90ms/step\n",
+ "creating index...\n",
+ "index created!\n",
+ "creating index...\n",
+ "index created!\n",
+ "Running per image evaluation...\n",
+ "Evaluate annotation type *bbox*\n",
+ "DONE (t=3.96s).\n",
+ "Accumulating evaluation results...\n",
+ "DONE (t=0.87s).\n",
+ " Average Precision (AP) @[ IoU=0.50:0.95 | area= all | maxDets=100 ] = 0.133\n",
+ " Average Precision (AP) @[ IoU=0.50 | area= all | maxDets=100 ] = 0.206\n",
+ " Average Precision (AP) @[ IoU=0.75 | area= all | maxDets=100 ] = 0.141\n",
+ " Average Precision (AP) @[ IoU=0.50:0.95 | area= small | maxDets=100 ] = 0.014\n",
+ " Average Precision (AP) @[ IoU=0.50:0.95 | area=medium | maxDets=100 ] = 0.065\n",
+ " Average Precision (AP) @[ IoU=0.50:0.95 | area= large | maxDets=100 ] = 0.156\n",
+ " Average Recall (AR) @[ IoU=0.50:0.95 | area= all | maxDets= 1 ] = 0.177\n",
+ " Average Recall (AR) @[ IoU=0.50:0.95 | area= all | maxDets= 10 ] = 0.216\n",
+ " Average Recall (AR) @[ IoU=0.50:0.95 | area= all | maxDets=100 ] = 0.217\n",
+ " Average Recall (AR) @[ IoU=0.50:0.95 | area= small | maxDets=100 ] = 0.021\n",
+ " Average Recall (AR) @[ IoU=0.50:0.95 | area=medium | maxDets=100 ] = 0.106\n",
+ " Average Recall (AR) @[ IoU=0.50:0.95 | area= large | maxDets=100 ] = 0.239\n",
+ "4138/4138 [==============================] - 608s 147ms/step - loss: 1.1902 - box_loss: 1.0915 - class_loss: 0.0987 - val_loss: 1.3892 - val_box_loss: 1.2849 - val_class_loss: 0.1043 - val_AP: 0.1328 - val_AP50: 0.2063 - val_AP75: 0.1407 - val_APs: 0.0145 - val_APm: 0.0651 - val_APl: 0.1556 - val_ARmax1: 0.1765 - val_ARmax10: 0.2159 - val_ARmax100: 0.2173 - val_ARs: 0.0214 - val_ARm: 0.1058 - val_ARl: 0.2389\n",
+ "Epoch 16/120\n",
+ "1238/1238 [==============================] - 112s 90ms/step\n",
+ "creating index...\n",
+ "index created!\n",
+ "creating index...\n",
+ "index created!\n",
+ "Running per image evaluation...\n",
+ "Evaluate annotation type *bbox*\n",
+ "DONE (t=4.21s).\n",
+ "Accumulating evaluation results...\n",
+ "DONE (t=0.91s).\n",
+ " Average Precision (AP) @[ IoU=0.50:0.95 | area= all | maxDets=100 ] = 0.158\n",
+ " Average Precision (AP) @[ IoU=0.50 | area= all | maxDets=100 ] = 0.241\n",
+ " Average Precision (AP) @[ IoU=0.75 | area= all | maxDets=100 ] = 0.169\n",
+ " Average Precision (AP) @[ IoU=0.50:0.95 | area= small | maxDets=100 ] = 0.015\n",
+ " Average Precision (AP) @[ IoU=0.50:0.95 | area=medium | maxDets=100 ] = 0.084\n",
+ " Average Precision (AP) @[ IoU=0.50:0.95 | area= large | maxDets=100 ] = 0.184\n",
+ " Average Recall (AR) @[ IoU=0.50:0.95 | area= all | maxDets= 1 ] = 0.194\n",
+ " Average Recall (AR) @[ IoU=0.50:0.95 | area= all | maxDets= 10 ] = 0.244\n",
+ " Average Recall (AR) @[ IoU=0.50:0.95 | area= all | maxDets=100 ] = 0.246\n",
+ " Average Recall (AR) @[ IoU=0.50:0.95 | area= small | maxDets=100 ] = 0.028\n",
+ " Average Recall (AR) @[ IoU=0.50:0.95 | area=medium | maxDets=100 ] = 0.133\n",
+ " Average Recall (AR) @[ IoU=0.50:0.95 | area= large | maxDets=100 ] = 0.270\n",
+ "4138/4138 [==============================] - 609s 147ms/step - loss: 1.1717 - box_loss: 1.0749 - class_loss: 0.0968 - val_loss: 1.3653 - val_box_loss: 1.2657 - val_class_loss: 0.0995 - val_AP: 0.1579 - val_AP50: 0.2411 - val_AP75: 0.1686 - val_APs: 0.0151 - val_APm: 0.0837 - val_APl: 0.1844 - val_ARmax1: 0.1943 - val_ARmax10: 0.2435 - val_ARmax100: 0.2456 - val_ARs: 0.0282 - val_ARm: 0.1330 - val_ARl: 0.2696\n",
+ "Epoch 17/120\n",
+ "1238/1238 [==============================] - 112s 90ms/step\n",
+ "creating index...\n",
+ "index created!\n",
+ "creating index...\n",
+ "index created!\n",
+ "Running per image evaluation...\n",
+ "Evaluate annotation type *bbox*\n",
+ "DONE (t=4.29s).\n",
+ "Accumulating evaluation results...\n",
+ "DONE (t=0.94s).\n",
+ " Average Precision (AP) @[ IoU=0.50:0.95 | area= all | maxDets=100 ] = 0.161\n",
+ " Average Precision (AP) @[ IoU=0.50 | area= all | maxDets=100 ] = 0.247\n",
+ " Average Precision (AP) @[ IoU=0.75 | area= all | maxDets=100 ] = 0.172\n",
+ " Average Precision (AP) @[ IoU=0.50:0.95 | area= small | maxDets=100 ] = 0.020\n",
+ " Average Precision (AP) @[ IoU=0.50:0.95 | area=medium | maxDets=100 ] = 0.081\n",
+ " Average Precision (AP) @[ IoU=0.50:0.95 | area= large | maxDets=100 ] = 0.190\n",
+ " Average Recall (AR) @[ IoU=0.50:0.95 | area= all | maxDets= 1 ] = 0.201\n",
+ " Average Recall (AR) @[ IoU=0.50:0.95 | area= all | maxDets= 10 ] = 0.252\n",
+ " Average Recall (AR) @[ IoU=0.50:0.95 | area= all | maxDets=100 ] = 0.254\n",
+ " Average Recall (AR) @[ IoU=0.50:0.95 | area= small | maxDets=100 ] = 0.038\n",
+ " Average Recall (AR) @[ IoU=0.50:0.95 | area=medium | maxDets=100 ] = 0.125\n",
+ " Average Recall (AR) @[ IoU=0.50:0.95 | area= large | maxDets=100 ] = 0.283\n",
+ "4138/4138 [==============================] - 609s 147ms/step - loss: 1.1573 - box_loss: 1.0629 - class_loss: 0.0944 - val_loss: 1.3572 - val_box_loss: 1.2598 - val_class_loss: 0.0975 - val_AP: 0.1610 - val_AP50: 0.2467 - val_AP75: 0.1725 - val_APs: 0.0197 - val_APm: 0.0810 - val_APl: 0.1901 - val_ARmax1: 0.2014 - val_ARmax10: 0.2520 - val_ARmax100: 0.2542 - val_ARs: 0.0384 - val_ARm: 0.1252 - val_ARl: 0.2830\n",
+ "Epoch 18/120\n",
+ "1238/1238 [==============================] - 111s 89ms/step\n",
+ "creating index...\n",
+ "index created!\n",
+ "creating index...\n",
+ "index created!\n",
+ "Running per image evaluation...\n",
+ "Evaluate annotation type *bbox*\n",
+ "DONE (t=4.34s).\n",
+ "Accumulating evaluation results...\n",
+ "DONE (t=0.95s).\n",
+ " Average Precision (AP) @[ IoU=0.50:0.95 | area= all | maxDets=100 ] = 0.159\n",
+ " Average Precision (AP) @[ IoU=0.50 | area= all | maxDets=100 ] = 0.247\n",
+ " Average Precision (AP) @[ IoU=0.75 | area= all | maxDets=100 ] = 0.170\n",
+ " Average Precision (AP) @[ IoU=0.50:0.95 | area= small | maxDets=100 ] = 0.020\n",
+ " Average Precision (AP) @[ IoU=0.50:0.95 | area=medium | maxDets=100 ] = 0.080\n",
+ " Average Precision (AP) @[ IoU=0.50:0.95 | area= large | maxDets=100 ] = 0.186\n",
+ " Average Recall (AR) @[ IoU=0.50:0.95 | area= all | maxDets= 1 ] = 0.201\n",
+ " Average Recall (AR) @[ IoU=0.50:0.95 | area= all | maxDets= 10 ] = 0.256\n",
+ " Average Recall (AR) @[ IoU=0.50:0.95 | area= all | maxDets=100 ] = 0.258\n",
+ " Average Recall (AR) @[ IoU=0.50:0.95 | area= small | maxDets=100 ] = 0.038\n",
+ " Average Recall (AR) @[ IoU=0.50:0.95 | area=medium | maxDets=100 ] = 0.126\n",
+ " Average Recall (AR) @[ IoU=0.50:0.95 | area= large | maxDets=100 ] = 0.287\n",
+ "4138/4138 [==============================] - 604s 146ms/step - loss: 1.1259 - box_loss: 1.0339 - class_loss: 0.0919 - val_loss: 1.3639 - val_box_loss: 1.2669 - val_class_loss: 0.0970 - val_AP: 0.1592 - val_AP50: 0.2474 - val_AP75: 0.1698 - val_APs: 0.0199 - val_APm: 0.0800 - val_APl: 0.1865 - val_ARmax1: 0.2013 - val_ARmax10: 0.2562 - val_ARmax100: 0.2577 - val_ARs: 0.0376 - val_ARm: 0.1261 - val_ARl: 0.2873\n",
+ "Epoch 19/120\n",
+ "1238/1238 [==============================] - 111s 89ms/step\n",
+ "creating index...\n",
+ "index created!\n",
+ "creating index...\n",
+ "index created!\n",
+ "Running per image evaluation...\n",
+ "Evaluate annotation type *bbox*\n",
+ "DONE (t=4.31s).\n",
+ "Accumulating evaluation results...\n",
+ "DONE (t=0.94s).\n",
+ " Average Precision (AP) @[ IoU=0.50:0.95 | area= all | maxDets=100 ] = 0.176\n",
+ " Average Precision (AP) @[ IoU=0.50 | area= all | maxDets=100 ] = 0.266\n",
+ " Average Precision (AP) @[ IoU=0.75 | area= all | maxDets=100 ] = 0.188\n",
+ " Average Precision (AP) @[ IoU=0.50:0.95 | area= small | maxDets=100 ] = 0.019\n",
+ " Average Precision (AP) @[ IoU=0.50:0.95 | area=medium | maxDets=100 ] = 0.089\n",
+ " Average Precision (AP) @[ IoU=0.50:0.95 | area= large | maxDets=100 ] = 0.203\n",
+ " Average Recall (AR) @[ IoU=0.50:0.95 | area= all | maxDets= 1 ] = 0.212\n",
+ " Average Recall (AR) @[ IoU=0.50:0.95 | area= all | maxDets= 10 ] = 0.268\n",
+ " Average Recall (AR) @[ IoU=0.50:0.95 | area= all | maxDets=100 ] = 0.271\n",
+ " Average Recall (AR) @[ IoU=0.50:0.95 | area= small | maxDets=100 ] = 0.032\n",
+ " Average Recall (AR) @[ IoU=0.50:0.95 | area=medium | maxDets=100 ] = 0.136\n",
+ " Average Recall (AR) @[ IoU=0.50:0.95 | area= large | maxDets=100 ] = 0.300\n",
+ "4138/4138 [==============================] - 602s 145ms/step - loss: 1.1077 - box_loss: 1.0178 - class_loss: 0.0900 - val_loss: 1.3421 - val_box_loss: 1.2475 - val_class_loss: 0.0945 - val_AP: 0.1757 - val_AP50: 0.2661 - val_AP75: 0.1880 - val_APs: 0.0188 - val_APm: 0.0893 - val_APl: 0.2034 - val_ARmax1: 0.2120 - val_ARmax10: 0.2684 - val_ARmax100: 0.2708 - val_ARs: 0.0320 - val_ARm: 0.1356 - val_ARl: 0.2999\n",
+ "Epoch 20/120\n",
+ "1238/1238 [==============================] - 110s 89ms/step\n",
+ "creating index...\n",
+ "index created!\n",
+ "creating index...\n",
+ "index created!\n",
+ "Running per image evaluation...\n",
+ "Evaluate annotation type *bbox*\n",
+ "DONE (t=4.21s).\n",
+ "Accumulating evaluation results...\n",
+ "DONE (t=0.93s).\n",
+ " Average Precision (AP) @[ IoU=0.50:0.95 | area= all | maxDets=100 ] = 0.177\n",
+ " Average Precision (AP) @[ IoU=0.50 | area= all | maxDets=100 ] = 0.270\n",
+ " Average Precision (AP) @[ IoU=0.75 | area= all | maxDets=100 ] = 0.191\n",
+ " Average Precision (AP) @[ IoU=0.50:0.95 | area= small | maxDets=100 ] = 0.021\n",
+ " Average Precision (AP) @[ IoU=0.50:0.95 | area=medium | maxDets=100 ] = 0.091\n",
+ " Average Precision (AP) @[ IoU=0.50:0.95 | area= large | maxDets=100 ] = 0.206\n",
+ " Average Recall (AR) @[ IoU=0.50:0.95 | area= all | maxDets= 1 ] = 0.212\n",
+ " Average Recall (AR) @[ IoU=0.50:0.95 | area= all | maxDets= 10 ] = 0.269\n",
+ " Average Recall (AR) @[ IoU=0.50:0.95 | area= all | maxDets=100 ] = 0.271\n",
+ " Average Recall (AR) @[ IoU=0.50:0.95 | area= small | maxDets=100 ] = 0.037\n",
+ " Average Recall (AR) @[ IoU=0.50:0.95 | area=medium | maxDets=100 ] = 0.140\n",
+ " Average Recall (AR) @[ IoU=0.50:0.95 | area= large | maxDets=100 ] = 0.299\n",
+ "4138/4138 [==============================] - 602s 145ms/step - loss: 1.0830 - box_loss: 0.9950 - class_loss: 0.0881 - val_loss: 1.3855 - val_box_loss: 1.2876 - val_class_loss: 0.0979 - val_AP: 0.1770 - val_AP50: 0.2697 - val_AP75: 0.1908 - val_APs: 0.0213 - val_APm: 0.0914 - val_APl: 0.2056 - val_ARmax1: 0.2124 - val_ARmax10: 0.2694 - val_ARmax100: 0.2712 - val_ARs: 0.0366 - val_ARm: 0.1404 - val_ARl: 0.2993\n",
+ "Epoch 21/120\n",
+ "1238/1238 [==============================] - 111s 89ms/step\n",
+ "creating index...\n",
+ "index created!\n",
+ "creating index...\n",
+ "index created!\n",
+ "Running per image evaluation...\n",
+ "Evaluate annotation type *bbox*\n",
+ "DONE (t=4.97s).\n",
+ "Accumulating evaluation results...\n",
+ "DONE (t=0.96s).\n",
+ " Average Precision (AP) @[ IoU=0.50:0.95 | area= all | maxDets=100 ] = 0.190\n",
+ " Average Precision (AP) @[ IoU=0.50 | area= all | maxDets=100 ] = 0.284\n",
+ " Average Precision (AP) @[ IoU=0.75 | area= all | maxDets=100 ] = 0.207\n",
+ " Average Precision (AP) @[ IoU=0.50:0.95 | area= small | maxDets=100 ] = 0.018\n",
+ " Average Precision (AP) @[ IoU=0.50:0.95 | area=medium | maxDets=100 ] = 0.099\n",
+ " Average Precision (AP) @[ IoU=0.50:0.95 | area= large | maxDets=100 ] = 0.217\n",
+ " Average Recall (AR) @[ IoU=0.50:0.95 | area= all | maxDets= 1 ] = 0.229\n",
+ " Average Recall (AR) @[ IoU=0.50:0.95 | area= all | maxDets= 10 ] = 0.287\n",
+ " Average Recall (AR) @[ IoU=0.50:0.95 | area= all | maxDets=100 ] = 0.289\n",
+ " Average Recall (AR) @[ IoU=0.50:0.95 | area= small | maxDets=100 ] = 0.031\n",
+ " Average Recall (AR) @[ IoU=0.50:0.95 | area=medium | maxDets=100 ] = 0.145\n",
+ " Average Recall (AR) @[ IoU=0.50:0.95 | area= large | maxDets=100 ] = 0.319\n",
+ "4138/4138 [==============================] - 603s 145ms/step - loss: 1.0674 - box_loss: 0.9810 - class_loss: 0.0863 - val_loss: 1.3425 - val_box_loss: 1.2493 - val_class_loss: 0.0931 - val_AP: 0.1900 - val_AP50: 0.2840 - val_AP75: 0.2069 - val_APs: 0.0183 - val_APm: 0.0995 - val_APl: 0.2173 - val_ARmax1: 0.2290 - val_ARmax10: 0.2867 - val_ARmax100: 0.2886 - val_ARs: 0.0306 - val_ARm: 0.1447 - val_ARl: 0.3187\n",
+ "Epoch 22/120\n",
+ "1238/1238 [==============================] - 111s 89ms/step\n",
+ "creating index...\n",
+ "index created!\n",
+ "creating index...\n",
+ "index created!\n",
+ "Running per image evaluation...\n",
+ "Evaluate annotation type *bbox*\n",
+ "DONE (t=4.41s).\n",
+ "Accumulating evaluation results...\n",
+ "DONE (t=0.95s).\n",
+ " Average Precision (AP) @[ IoU=0.50:0.95 | area= all | maxDets=100 ] = 0.194\n",
+ " Average Precision (AP) @[ IoU=0.50 | area= all | maxDets=100 ] = 0.293\n",
+ " Average Precision (AP) @[ IoU=0.75 | area= all | maxDets=100 ] = 0.209\n",
+ " Average Precision (AP) @[ IoU=0.50:0.95 | area= small | maxDets=100 ] = 0.023\n",
+ " Average Precision (AP) @[ IoU=0.50:0.95 | area=medium | maxDets=100 ] = 0.096\n",
+ " Average Precision (AP) @[ IoU=0.50:0.95 | area= large | maxDets=100 ] = 0.225\n",
+ " Average Recall (AR) @[ IoU=0.50:0.95 | area= all | maxDets= 1 ] = 0.231\n",
+ " Average Recall (AR) @[ IoU=0.50:0.95 | area= all | maxDets= 10 ] = 0.291\n",
+ " Average Recall (AR) @[ IoU=0.50:0.95 | area= all | maxDets=100 ] = 0.293\n",
+ " Average Recall (AR) @[ IoU=0.50:0.95 | area= small | maxDets=100 ] = 0.040\n",
+ " Average Recall (AR) @[ IoU=0.50:0.95 | area=medium | maxDets=100 ] = 0.150\n",
+ " Average Recall (AR) @[ IoU=0.50:0.95 | area= large | maxDets=100 ] = 0.324\n",
+ "4138/4138 [==============================] - 602s 145ms/step - loss: 1.0508 - box_loss: 0.9663 - class_loss: 0.0845 - val_loss: 1.3495 - val_box_loss: 1.2578 - val_class_loss: 0.0917 - val_AP: 0.1935 - val_AP50: 0.2931 - val_AP75: 0.2091 - val_APs: 0.0234 - val_APm: 0.0964 - val_APl: 0.2253 - val_ARmax1: 0.2311 - val_ARmax10: 0.2913 - val_ARmax100: 0.2934 - val_ARs: 0.0398 - val_ARm: 0.1498 - val_ARl: 0.3241\n",
+ "Epoch 23/120\n",
+ "1238/1238 [==============================] - 111s 89ms/step\n",
+ "creating index...\n",
+ "index created!\n",
+ "creating index...\n",
+ "index created!\n",
+ "Running per image evaluation...\n",
+ "Evaluate annotation type *bbox*\n",
+ "DONE (t=4.42s).\n",
+ "Accumulating evaluation results...\n",
+ "DONE (t=0.97s).\n",
+ " Average Precision (AP) @[ IoU=0.50:0.95 | area= all | maxDets=100 ] = 0.204\n",
+ " Average Precision (AP) @[ IoU=0.50 | area= all | maxDets=100 ] = 0.306\n",
+ " Average Precision (AP) @[ IoU=0.75 | area= all | maxDets=100 ] = 0.218\n",
+ " Average Precision (AP) @[ IoU=0.50:0.95 | area= small | maxDets=100 ] = 0.019\n",
+ " Average Precision (AP) @[ IoU=0.50:0.95 | area=medium | maxDets=100 ] = 0.102\n",
+ " Average Precision (AP) @[ IoU=0.50:0.95 | area= large | maxDets=100 ] = 0.235\n",
+ " Average Recall (AR) @[ IoU=0.50:0.95 | area= all | maxDets= 1 ] = 0.242\n",
+ " Average Recall (AR) @[ IoU=0.50:0.95 | area= all | maxDets= 10 ] = 0.307\n",
+ " Average Recall (AR) @[ IoU=0.50:0.95 | area= all | maxDets=100 ] = 0.309\n",
+ " Average Recall (AR) @[ IoU=0.50:0.95 | area= small | maxDets=100 ] = 0.037\n",
+ " Average Recall (AR) @[ IoU=0.50:0.95 | area=medium | maxDets=100 ] = 0.169\n",
+ " Average Recall (AR) @[ IoU=0.50:0.95 | area= large | maxDets=100 ] = 0.341\n",
+ "4138/4138 [==============================] - 604s 146ms/step - loss: 1.0350 - box_loss: 0.9521 - class_loss: 0.0828 - val_loss: 1.3130 - val_box_loss: 1.2233 - val_class_loss: 0.0898 - val_AP: 0.2039 - val_AP50: 0.3057 - val_AP75: 0.2180 - val_APs: 0.0193 - val_APm: 0.1022 - val_APl: 0.2348 - val_ARmax1: 0.2422 - val_ARmax10: 0.3070 - val_ARmax100: 0.3091 - val_ARs: 0.0375 - val_ARm: 0.1691 - val_ARl: 0.3412\n",
+ "Epoch 24/120\n",
+ "1238/1238 [==============================] - 111s 89ms/step\n",
+ "creating index...\n",
+ "index created!\n",
+ "creating index...\n",
+ "index created!\n",
+ "Running per image evaluation...\n",
+ "Evaluate annotation type *bbox*\n",
+ "DONE (t=4.48s).\n",
+ "Accumulating evaluation results...\n",
+ "DONE (t=0.98s).\n",
+ " Average Precision (AP) @[ IoU=0.50:0.95 | area= all | maxDets=100 ] = 0.212\n",
+ " Average Precision (AP) @[ IoU=0.50 | area= all | maxDets=100 ] = 0.316\n",
+ " Average Precision (AP) @[ IoU=0.75 | area= all | maxDets=100 ] = 0.229\n",
+ " Average Precision (AP) @[ IoU=0.50:0.95 | area= small | maxDets=100 ] = 0.023\n",
+ " Average Precision (AP) @[ IoU=0.50:0.95 | area=medium | maxDets=100 ] = 0.098\n",
+ " Average Precision (AP) @[ IoU=0.50:0.95 | area= large | maxDets=100 ] = 0.250\n",
+ " Average Recall (AR) @[ IoU=0.50:0.95 | area= all | maxDets= 1 ] = 0.252\n",
+ " Average Recall (AR) @[ IoU=0.50:0.95 | area= all | maxDets= 10 ] = 0.316\n",
+ " Average Recall (AR) @[ IoU=0.50:0.95 | area= all | maxDets=100 ] = 0.317\n",
+ " Average Recall (AR) @[ IoU=0.50:0.95 | area= small | maxDets=100 ] = 0.040\n",
+ " Average Recall (AR) @[ IoU=0.50:0.95 | area=medium | maxDets=100 ] = 0.154\n",
+ " Average Recall (AR) @[ IoU=0.50:0.95 | area= large | maxDets=100 ] = 0.357\n",
+ "4138/4138 [==============================] - 604s 146ms/step - loss: 1.0194 - box_loss: 0.9381 - class_loss: 0.0814 - val_loss: 1.3040 - val_box_loss: 1.2155 - val_class_loss: 0.0885 - val_AP: 0.2125 - val_AP50: 0.3158 - val_AP75: 0.2290 - val_APs: 0.0232 - val_APm: 0.0982 - val_APl: 0.2502 - val_ARmax1: 0.2524 - val_ARmax10: 0.3155 - val_ARmax100: 0.3172 - val_ARs: 0.0405 - val_ARm: 0.1543 - val_ARl: 0.3571\n",
+ "Epoch 25/120\n",
+ "1238/1238 [==============================] - 111s 89ms/step\n",
+ "creating index...\n",
+ "index created!\n",
+ "creating index...\n",
+ "index created!\n",
+ "Running per image evaluation...\n",
+ "Evaluate annotation type *bbox*\n",
+ "DONE (t=4.45s).\n",
+ "Accumulating evaluation results...\n",
+ "DONE (t=0.97s).\n",
+ " Average Precision (AP) @[ IoU=0.50:0.95 | area= all | maxDets=100 ] = 0.218\n",
+ " Average Precision (AP) @[ IoU=0.50 | area= all | maxDets=100 ] = 0.327\n",
+ " Average Precision (AP) @[ IoU=0.75 | area= all | maxDets=100 ] = 0.236\n",
+ " Average Precision (AP) @[ IoU=0.50:0.95 | area= small | maxDets=100 ] = 0.021\n",
+ " Average Precision (AP) @[ IoU=0.50:0.95 | area=medium | maxDets=100 ] = 0.103\n",
+ " Average Precision (AP) @[ IoU=0.50:0.95 | area= large | maxDets=100 ] = 0.254\n",
+ " Average Recall (AR) @[ IoU=0.50:0.95 | area= all | maxDets= 1 ] = 0.251\n",
+ " Average Recall (AR) @[ IoU=0.50:0.95 | area= all | maxDets= 10 ] = 0.320\n",
+ " Average Recall (AR) @[ IoU=0.50:0.95 | area= all | maxDets=100 ] = 0.323\n",
+ " Average Recall (AR) @[ IoU=0.50:0.95 | area= small | maxDets=100 ] = 0.038\n",
+ " Average Recall (AR) @[ IoU=0.50:0.95 | area=medium | maxDets=100 ] = 0.157\n",
+ " Average Recall (AR) @[ IoU=0.50:0.95 | area= large | maxDets=100 ] = 0.361\n",
+ "4138/4138 [==============================] - 603s 146ms/step - loss: 1.0019 - box_loss: 0.9219 - class_loss: 0.0800 - val_loss: 1.3165 - val_box_loss: 1.2270 - val_class_loss: 0.0894 - val_AP: 0.2179 - val_AP50: 0.3266 - val_AP75: 0.2365 - val_APs: 0.0208 - val_APm: 0.1035 - val_APl: 0.2541 - val_ARmax1: 0.2513 - val_ARmax10: 0.3197 - val_ARmax100: 0.3226 - val_ARs: 0.0379 - val_ARm: 0.1568 - val_ARl: 0.3611\n",
+ "Epoch 26/120\n",
+ "1238/1238 [==============================] - 111s 89ms/step\n",
+ "creating index...\n",
+ "index created!\n",
+ "creating index...\n",
+ "index created!\n",
+ "Running per image evaluation...\n",
+ "Evaluate annotation type *bbox*\n",
+ "DONE (t=4.56s).\n",
+ "Accumulating evaluation results...\n",
+ "DONE (t=0.99s).\n",
+ " Average Precision (AP) @[ IoU=0.50:0.95 | area= all | maxDets=100 ] = 0.225\n",
+ " Average Precision (AP) @[ IoU=0.50 | area= all | maxDets=100 ] = 0.332\n",
+ " Average Precision (AP) @[ IoU=0.75 | area= all | maxDets=100 ] = 0.244\n",
+ " Average Precision (AP) @[ IoU=0.50:0.95 | area= small | maxDets=100 ] = 0.022\n",
+ " Average Precision (AP) @[ IoU=0.50:0.95 | area=medium | maxDets=100 ] = 0.108\n",
+ " Average Precision (AP) @[ IoU=0.50:0.95 | area= large | maxDets=100 ] = 0.261\n",
+ " Average Recall (AR) @[ IoU=0.50:0.95 | area= all | maxDets= 1 ] = 0.257\n",
+ " Average Recall (AR) @[ IoU=0.50:0.95 | area= all | maxDets= 10 ] = 0.326\n",
+ " Average Recall (AR) @[ IoU=0.50:0.95 | area= all | maxDets=100 ] = 0.328\n",
+ " Average Recall (AR) @[ IoU=0.50:0.95 | area= small | maxDets=100 ] = 0.041\n",
+ " Average Recall (AR) @[ IoU=0.50:0.95 | area=medium | maxDets=100 ] = 0.165\n",
+ " Average Recall (AR) @[ IoU=0.50:0.95 | area= large | maxDets=100 ] = 0.368\n",
+ "4138/4138 [==============================] - 604s 146ms/step - loss: 0.9869 - box_loss: 0.9086 - class_loss: 0.0784 - val_loss: 1.2998 - val_box_loss: 1.2129 - val_class_loss: 0.0870 - val_AP: 0.2245 - val_AP50: 0.3321 - val_AP75: 0.2440 - val_APs: 0.0215 - val_APm: 0.1084 - val_APl: 0.2607 - val_ARmax1: 0.2570 - val_ARmax10: 0.3265 - val_ARmax100: 0.3285 - val_ARs: 0.0409 - val_ARm: 0.1651 - val_ARl: 0.3679\n",
+ "Epoch 27/120\n",
+ "1238/1238 [==============================] - 111s 89ms/step\n",
+ "creating index...\n",
+ "index created!\n",
+ "creating index...\n",
+ "index created!\n",
+ "Running per image evaluation...\n",
+ "Evaluate annotation type *bbox*\n",
+ "DONE (t=4.59s).\n",
+ "Accumulating evaluation results...\n",
+ "DONE (t=1.00s).\n",
+ " Average Precision (AP) @[ IoU=0.50:0.95 | area= all | maxDets=100 ] = 0.219\n",
+ " Average Precision (AP) @[ IoU=0.50 | area= all | maxDets=100 ] = 0.330\n",
+ " Average Precision (AP) @[ IoU=0.75 | area= all | maxDets=100 ] = 0.237\n",
+ " Average Precision (AP) @[ IoU=0.50:0.95 | area= small | maxDets=100 ] = 0.021\n",
+ " Average Precision (AP) @[ IoU=0.50:0.95 | area=medium | maxDets=100 ] = 0.110\n",
+ " Average Precision (AP) @[ IoU=0.50:0.95 | area= large | maxDets=100 ] = 0.254\n",
+ " Average Recall (AR) @[ IoU=0.50:0.95 | area= all | maxDets= 1 ] = 0.250\n",
+ " Average Recall (AR) @[ IoU=0.50:0.95 | area= all | maxDets= 10 ] = 0.321\n",
+ " Average Recall (AR) @[ IoU=0.50:0.95 | area= all | maxDets=100 ] = 0.324\n",
+ " Average Recall (AR) @[ IoU=0.50:0.95 | area= small | maxDets=100 ] = 0.038\n",
+ " Average Recall (AR) @[ IoU=0.50:0.95 | area=medium | maxDets=100 ] = 0.160\n",
+ " Average Recall (AR) @[ IoU=0.50:0.95 | area= large | maxDets=100 ] = 0.362\n",
+ "4138/4138 [==============================] - 603s 146ms/step - loss: 0.9912 - box_loss: 0.9128 - class_loss: 0.0784 - val_loss: 1.3152 - val_box_loss: 1.2280 - val_class_loss: 0.0871 - val_AP: 0.2189 - val_AP50: 0.3299 - val_AP75: 0.2372 - val_APs: 0.0215 - val_APm: 0.1100 - val_APl: 0.2543 - val_ARmax1: 0.2501 - val_ARmax10: 0.3212 - val_ARmax100: 0.3235 - val_ARs: 0.0384 - val_ARm: 0.1604 - val_ARl: 0.3620\n",
+ "Epoch 28/120\n",
+ "1238/1238 [==============================] - 111s 89ms/step\n",
+ "creating index...\n",
+ "index created!\n",
+ "creating index...\n",
+ "index created!\n",
+ "Running per image evaluation...\n",
+ "Evaluate annotation type *bbox*\n",
+ "DONE (t=4.50s).\n",
+ "Accumulating evaluation results...\n",
+ "DONE (t=0.98s).\n",
+ " Average Precision (AP) @[ IoU=0.50:0.95 | area= all | maxDets=100 ] = 0.226\n",
+ " Average Precision (AP) @[ IoU=0.50 | area= all | maxDets=100 ] = 0.337\n",
+ " Average Precision (AP) @[ IoU=0.75 | area= all | maxDets=100 ] = 0.244\n",
+ " Average Precision (AP) @[ IoU=0.50:0.95 | area= small | maxDets=100 ] = 0.032\n",
+ " Average Precision (AP) @[ IoU=0.50:0.95 | area=medium | maxDets=100 ] = 0.111\n",
+ " Average Precision (AP) @[ IoU=0.50:0.95 | area= large | maxDets=100 ] = 0.263\n",
+ " Average Recall (AR) @[ IoU=0.50:0.95 | area= all | maxDets= 1 ] = 0.265\n",
+ " Average Recall (AR) @[ IoU=0.50:0.95 | area= all | maxDets= 10 ] = 0.336\n",
+ " Average Recall (AR) @[ IoU=0.50:0.95 | area= all | maxDets=100 ] = 0.339\n",
+ " Average Recall (AR) @[ IoU=0.50:0.95 | area= small | maxDets=100 ] = 0.053\n",
+ " Average Recall (AR) @[ IoU=0.50:0.95 | area=medium | maxDets=100 ] = 0.170\n",
+ " Average Recall (AR) @[ IoU=0.50:0.95 | area= large | maxDets=100 ] = 0.378\n",
+ "4138/4138 [==============================] - 603s 146ms/step - loss: 0.9677 - box_loss: 0.8910 - class_loss: 0.0767 - val_loss: 1.2900 - val_box_loss: 1.2043 - val_class_loss: 0.0857 - val_AP: 0.2261 - val_AP50: 0.3373 - val_AP75: 0.2444 - val_APs: 0.0319 - val_APm: 0.1110 - val_APl: 0.2632 - val_ARmax1: 0.2649 - val_ARmax10: 0.3365 - val_ARmax100: 0.3386 - val_ARs: 0.0533 - val_ARm: 0.1699 - val_ARl: 0.3782\n",
+ "Epoch 29/120\n",
+ "1238/1238 [==============================] - 111s 89ms/step\n",
+ "creating index...\n",
+ "index created!\n",
+ "creating index...\n",
+ "index created!\n",
+ "Running per image evaluation...\n",
+ "Evaluate annotation type *bbox*\n",
+ "DONE (t=4.59s).\n",
+ "Accumulating evaluation results...\n",
+ "DONE (t=1.00s).\n",
+ " Average Precision (AP) @[ IoU=0.50:0.95 | area= all | maxDets=100 ] = 0.236\n",
+ " Average Precision (AP) @[ IoU=0.50 | area= all | maxDets=100 ] = 0.349\n",
+ " Average Precision (AP) @[ IoU=0.75 | area= all | maxDets=100 ] = 0.253\n",
+ " Average Precision (AP) @[ IoU=0.50:0.95 | area= small | maxDets=100 ] = 0.026\n",
+ " Average Precision (AP) @[ IoU=0.50:0.95 | area=medium | maxDets=100 ] = 0.110\n",
+ " Average Precision (AP) @[ IoU=0.50:0.95 | area= large | maxDets=100 ] = 0.279\n",
+ " Average Recall (AR) @[ IoU=0.50:0.95 | area= all | maxDets= 1 ] = 0.265\n",
+ " Average Recall (AR) @[ IoU=0.50:0.95 | area= all | maxDets= 10 ] = 0.341\n",
+ " Average Recall (AR) @[ IoU=0.50:0.95 | area= all | maxDets=100 ] = 0.343\n",
+ " Average Recall (AR) @[ IoU=0.50:0.95 | area= small | maxDets=100 ] = 0.044\n",
+ " Average Recall (AR) @[ IoU=0.50:0.95 | area=medium | maxDets=100 ] = 0.168\n",
+ " Average Recall (AR) @[ IoU=0.50:0.95 | area= large | maxDets=100 ] = 0.387\n",
+ "4138/4138 [==============================] - 605s 146ms/step - loss: 0.9463 - box_loss: 0.8713 - class_loss: 0.0750 - val_loss: 1.2881 - val_box_loss: 1.2031 - val_class_loss: 0.0850 - val_AP: 0.2356 - val_AP50: 0.3489 - val_AP75: 0.2529 - val_APs: 0.0257 - val_APm: 0.1103 - val_APl: 0.2786 - val_ARmax1: 0.2648 - val_ARmax10: 0.3408 - val_ARmax100: 0.3434 - val_ARs: 0.0444 - val_ARm: 0.1682 - val_ARl: 0.3865\n",
+ "Epoch 30/120\n",
+ "1238/1238 [==============================] - 111s 89ms/step\n",
+ "creating index...\n",
+ "index created!\n",
+ "creating index...\n",
+ "index created!\n",
+ "Running per image evaluation...\n",
+ "Evaluate annotation type *bbox*\n",
+ "DONE (t=4.57s).\n",
+ "Accumulating evaluation results...\n",
+ "DONE (t=1.00s).\n",
+ " Average Precision (AP) @[ IoU=0.50:0.95 | area= all | maxDets=100 ] = 0.242\n",
+ " Average Precision (AP) @[ IoU=0.50 | area= all | maxDets=100 ] = 0.359\n",
+ " Average Precision (AP) @[ IoU=0.75 | area= all | maxDets=100 ] = 0.262\n",
+ " Average Precision (AP) @[ IoU=0.50:0.95 | area= small | maxDets=100 ] = 0.025\n",
+ " Average Precision (AP) @[ IoU=0.50:0.95 | area=medium | maxDets=100 ] = 0.118\n",
+ " Average Precision (AP) @[ IoU=0.50:0.95 | area= large | maxDets=100 ] = 0.283\n",
+ " Average Recall (AR) @[ IoU=0.50:0.95 | area= all | maxDets= 1 ] = 0.267\n",
+ " Average Recall (AR) @[ IoU=0.50:0.95 | area= all | maxDets= 10 ] = 0.346\n",
+ " Average Recall (AR) @[ IoU=0.50:0.95 | area= all | maxDets=100 ] = 0.349\n",
+ " Average Recall (AR) @[ IoU=0.50:0.95 | area= small | maxDets=100 ] = 0.047\n",
+ " Average Recall (AR) @[ IoU=0.50:0.95 | area=medium | maxDets=100 ] = 0.193\n",
+ " Average Recall (AR) @[ IoU=0.50:0.95 | area= large | maxDets=100 ] = 0.390\n",
+ "4138/4138 [==============================] - 605s 146ms/step - loss: 0.9327 - box_loss: 0.8588 - class_loss: 0.0739 - val_loss: 1.2842 - val_box_loss: 1.2005 - val_class_loss: 0.0837 - val_AP: 0.2424 - val_AP50: 0.3590 - val_AP75: 0.2625 - val_APs: 0.0253 - val_APm: 0.1184 - val_APl: 0.2826 - val_ARmax1: 0.2675 - val_ARmax10: 0.3460 - val_ARmax100: 0.3487 - val_ARs: 0.0470 - val_ARm: 0.1929 - val_ARl: 0.3903\n",
+ "Epoch 31/120\n",
+ "1238/1238 [==============================] - 111s 89ms/step\n",
+ "creating index...\n",
+ "index created!\n",
+ "creating index...\n",
+ "index created!\n",
+ "Running per image evaluation...\n",
+ "Evaluate annotation type *bbox*\n",
+ "DONE (t=5.17s).\n",
+ "Accumulating evaluation results...\n",
+ "DONE (t=0.98s).\n",
+ " Average Precision (AP) @[ IoU=0.50:0.95 | area= all | maxDets=100 ] = 0.240\n",
+ " Average Precision (AP) @[ IoU=0.50 | area= all | maxDets=100 ] = 0.354\n",
+ " Average Precision (AP) @[ IoU=0.75 | area= all | maxDets=100 ] = 0.259\n",
+ " Average Precision (AP) @[ IoU=0.50:0.95 | area= small | maxDets=100 ] = 0.021\n",
+ " Average Precision (AP) @[ IoU=0.50:0.95 | area=medium | maxDets=100 ] = 0.123\n",
+ " Average Precision (AP) @[ IoU=0.50:0.95 | area= large | maxDets=100 ] = 0.276\n",
+ " Average Recall (AR) @[ IoU=0.50:0.95 | area= all | maxDets= 1 ] = 0.270\n",
+ " Average Recall (AR) @[ IoU=0.50:0.95 | area= all | maxDets= 10 ] = 0.344\n",
+ " Average Recall (AR) @[ IoU=0.50:0.95 | area= all | maxDets=100 ] = 0.346\n",
+ " Average Recall (AR) @[ IoU=0.50:0.95 | area= small | maxDets=100 ] = 0.043\n",
+ " Average Recall (AR) @[ IoU=0.50:0.95 | area=medium | maxDets=100 ] = 0.182\n",
+ " Average Recall (AR) @[ IoU=0.50:0.95 | area= large | maxDets=100 ] = 0.384\n",
+ "4138/4138 [==============================] - 604s 146ms/step - loss: 0.9352 - box_loss: 0.8616 - class_loss: 0.0736 - val_loss: 1.2895 - val_box_loss: 1.2052 - val_class_loss: 0.0843 - val_AP: 0.2398 - val_AP50: 0.3543 - val_AP75: 0.2587 - val_APs: 0.0212 - val_APm: 0.1228 - val_APl: 0.2762 - val_ARmax1: 0.2696 - val_ARmax10: 0.3438 - val_ARmax100: 0.3463 - val_ARs: 0.0434 - val_ARm: 0.1816 - val_ARl: 0.3837\n",
+ "Epoch 32/120\n",
+ "1238/1238 [==============================] - 111s 89ms/step\n",
+ "creating index...\n",
+ "index created!\n",
+ "creating index...\n",
+ "index created!\n",
+ "Running per image evaluation...\n",
+ "Evaluate annotation type *bbox*\n",
+ "DONE (t=4.50s).\n",
+ "Accumulating evaluation results...\n",
+ "DONE (t=0.98s).\n",
+ " Average Precision (AP) @[ IoU=0.50:0.95 | area= all | maxDets=100 ] = 0.249\n",
+ " Average Precision (AP) @[ IoU=0.50 | area= all | maxDets=100 ] = 0.365\n",
+ " Average Precision (AP) @[ IoU=0.75 | area= all | maxDets=100 ] = 0.272\n",
+ " Average Precision (AP) @[ IoU=0.50:0.95 | area= small | maxDets=100 ] = 0.026\n",
+ " Average Precision (AP) @[ IoU=0.50:0.95 | area=medium | maxDets=100 ] = 0.121\n",
+ " Average Precision (AP) @[ IoU=0.50:0.95 | area= large | maxDets=100 ] = 0.290\n",
+ " Average Recall (AR) @[ IoU=0.50:0.95 | area= all | maxDets= 1 ] = 0.276\n",
+ " Average Recall (AR) @[ IoU=0.50:0.95 | area= all | maxDets= 10 ] = 0.355\n",
+ " Average Recall (AR) @[ IoU=0.50:0.95 | area= all | maxDets=100 ] = 0.357\n",
+ " Average Recall (AR) @[ IoU=0.50:0.95 | area= small | maxDets=100 ] = 0.045\n",
+ " Average Recall (AR) @[ IoU=0.50:0.95 | area=medium | maxDets=100 ] = 0.183\n",
+ " Average Recall (AR) @[ IoU=0.50:0.95 | area= large | maxDets=100 ] = 0.399\n",
+ "4138/4138 [==============================] - 604s 146ms/step - loss: 0.9127 - box_loss: 0.8407 - class_loss: 0.0720 - val_loss: 1.2811 - val_box_loss: 1.1979 - val_class_loss: 0.0832 - val_AP: 0.2487 - val_AP50: 0.3646 - val_AP75: 0.2717 - val_APs: 0.0258 - val_APm: 0.1215 - val_APl: 0.2905 - val_ARmax1: 0.2761 - val_ARmax10: 0.3550 - val_ARmax100: 0.3570 - val_ARs: 0.0448 - val_ARm: 0.1827 - val_ARl: 0.3985\n",
+ "Epoch 33/120\n",
+ "1238/1238 [==============================] - 111s 89ms/step\n",
+ "creating index...\n",
+ "index created!\n",
+ "creating index...\n",
+ "index created!\n",
+ "Running per image evaluation...\n",
+ "Evaluate annotation type *bbox*\n",
+ "DONE (t=5.17s).\n",
+ "Accumulating evaluation results...\n",
+ "DONE (t=0.97s).\n",
+ " Average Precision (AP) @[ IoU=0.50:0.95 | area= all | maxDets=100 ] = 0.251\n",
+ " Average Precision (AP) @[ IoU=0.50 | area= all | maxDets=100 ] = 0.371\n",
+ " Average Precision (AP) @[ IoU=0.75 | area= all | maxDets=100 ] = 0.276\n",
+ " Average Precision (AP) @[ IoU=0.50:0.95 | area= small | maxDets=100 ] = 0.033\n",
+ " Average Precision (AP) @[ IoU=0.50:0.95 | area=medium | maxDets=100 ] = 0.118\n",
+ " Average Precision (AP) @[ IoU=0.50:0.95 | area= large | maxDets=100 ] = 0.297\n",
+ " Average Recall (AR) @[ IoU=0.50:0.95 | area= all | maxDets= 1 ] = 0.279\n",
+ " Average Recall (AR) @[ IoU=0.50:0.95 | area= all | maxDets= 10 ] = 0.356\n",
+ " Average Recall (AR) @[ IoU=0.50:0.95 | area= all | maxDets=100 ] = 0.359\n",
+ " Average Recall (AR) @[ IoU=0.50:0.95 | area= small | maxDets=100 ] = 0.050\n",
+ " Average Recall (AR) @[ IoU=0.50:0.95 | area=medium | maxDets=100 ] = 0.196\n",
+ " Average Recall (AR) @[ IoU=0.50:0.95 | area= large | maxDets=100 ] = 0.405\n",
+ "4138/4138 [==============================] - 604s 146ms/step - loss: 0.8977 - box_loss: 0.8271 - class_loss: 0.0706 - val_loss: 1.2887 - val_box_loss: 1.2060 - val_class_loss: 0.0827 - val_AP: 0.2508 - val_AP50: 0.3712 - val_AP75: 0.2756 - val_APs: 0.0326 - val_APm: 0.1178 - val_APl: 0.2970 - val_ARmax1: 0.2792 - val_ARmax10: 0.3563 - val_ARmax100: 0.3586 - val_ARs: 0.0503 - val_ARm: 0.1955 - val_ARl: 0.4048\n",
+ "Epoch 34/120\n",
+ "1238/1238 [==============================] - 111s 89ms/step\n",
+ "creating index...\n",
+ "index created!\n",
+ "creating index...\n",
+ "index created!\n",
+ "Running per image evaluation...\n",
+ "Evaluate annotation type *bbox*\n",
+ "DONE (t=4.65s).\n",
+ "Accumulating evaluation results...\n",
+ "DONE (t=1.01s).\n",
+ " Average Precision (AP) @[ IoU=0.50:0.95 | area= all | maxDets=100 ] = 0.255\n",
+ " Average Precision (AP) @[ IoU=0.50 | area= all | maxDets=100 ] = 0.375\n",
+ " Average Precision (AP) @[ IoU=0.75 | area= all | maxDets=100 ] = 0.278\n",
+ " Average Precision (AP) @[ IoU=0.50:0.95 | area= small | maxDets=100 ] = 0.027\n",
+ " Average Precision (AP) @[ IoU=0.50:0.95 | area=medium | maxDets=100 ] = 0.130\n",
+ " Average Precision (AP) @[ IoU=0.50:0.95 | area= large | maxDets=100 ] = 0.295\n",
+ " Average Recall (AR) @[ IoU=0.50:0.95 | area= all | maxDets= 1 ] = 0.273\n",
+ " Average Recall (AR) @[ IoU=0.50:0.95 | area= all | maxDets= 10 ] = 0.354\n",
+ " Average Recall (AR) @[ IoU=0.50:0.95 | area= all | maxDets=100 ] = 0.356\n",
+ " Average Recall (AR) @[ IoU=0.50:0.95 | area= small | maxDets=100 ] = 0.052\n",
+ " Average Recall (AR) @[ IoU=0.50:0.95 | area=medium | maxDets=100 ] = 0.189\n",
+ " Average Recall (AR) @[ IoU=0.50:0.95 | area= large | maxDets=100 ] = 0.398\n",
+ "4138/4138 [==============================] - 603s 146ms/step - loss: 0.9026 - box_loss: 0.8319 - class_loss: 0.0707 - val_loss: 1.2777 - val_box_loss: 1.1964 - val_class_loss: 0.0813 - val_AP: 0.2549 - val_AP50: 0.3751 - val_AP75: 0.2778 - val_APs: 0.0273 - val_APm: 0.1301 - val_APl: 0.2946 - val_ARmax1: 0.2730 - val_ARmax10: 0.3539 - val_ARmax100: 0.3564 - val_ARs: 0.0517 - val_ARm: 0.1891 - val_ARl: 0.3976\n",
+ "Epoch 35/120\n",
+ "1238/1238 [==============================] - 110s 89ms/step\n",
+ "creating index...\n",
+ "index created!\n",
+ "creating index...\n",
+ "index created!\n",
+ "Running per image evaluation...\n",
+ "Evaluate annotation type *bbox*\n",
+ "DONE (t=5.30s).\n",
+ "Accumulating evaluation results...\n",
+ "DONE (t=1.02s).\n",
+ " Average Precision (AP) @[ IoU=0.50:0.95 | area= all | maxDets=100 ] = 0.262\n",
+ " Average Precision (AP) @[ IoU=0.50 | area= all | maxDets=100 ] = 0.384\n",
+ " Average Precision (AP) @[ IoU=0.75 | area= all | maxDets=100 ] = 0.285\n",
+ " Average Precision (AP) @[ IoU=0.50:0.95 | area= small | maxDets=100 ] = 0.030\n",
+ " Average Precision (AP) @[ IoU=0.50:0.95 | area=medium | maxDets=100 ] = 0.132\n",
+ " Average Precision (AP) @[ IoU=0.50:0.95 | area= large | maxDets=100 ] = 0.305\n",
+ " Average Recall (AR) @[ IoU=0.50:0.95 | area= all | maxDets= 1 ] = 0.286\n",
+ " Average Recall (AR) @[ IoU=0.50:0.95 | area= all | maxDets= 10 ] = 0.368\n",
+ " Average Recall (AR) @[ IoU=0.50:0.95 | area= all | maxDets=100 ] = 0.371\n",
+ " Average Recall (AR) @[ IoU=0.50:0.95 | area= small | maxDets=100 ] = 0.056\n",
+ " Average Recall (AR) @[ IoU=0.50:0.95 | area=medium | maxDets=100 ] = 0.190\n",
+ " Average Recall (AR) @[ IoU=0.50:0.95 | area= large | maxDets=100 ] = 0.418\n",
+ "4138/4138 [==============================] - 604s 146ms/step - loss: 0.8759 - box_loss: 0.8072 - class_loss: 0.0687 - val_loss: 1.2667 - val_box_loss: 1.1857 - val_class_loss: 0.0810 - val_AP: 0.2618 - val_AP50: 0.3838 - val_AP75: 0.2846 - val_APs: 0.0297 - val_APm: 0.1319 - val_APl: 0.3055 - val_ARmax1: 0.2858 - val_ARmax10: 0.3679 - val_ARmax100: 0.3710 - val_ARs: 0.0557 - val_ARm: 0.1896 - val_ARl: 0.4176\n",
+ "Epoch 36/120\n",
+ "1238/1238 [==============================] - 111s 89ms/step\n",
+ "creating index...\n",
+ "index created!\n",
+ "creating index...\n",
+ "index created!\n",
+ "Running per image evaluation...\n",
+ "Evaluate annotation type *bbox*\n",
+ "DONE (t=4.58s).\n",
+ "Accumulating evaluation results...\n",
+ "DONE (t=0.98s).\n",
+ " Average Precision (AP) @[ IoU=0.50:0.95 | area= all | maxDets=100 ] = 0.264\n",
+ " Average Precision (AP) @[ IoU=0.50 | area= all | maxDets=100 ] = 0.386\n",
+ " Average Precision (AP) @[ IoU=0.75 | area= all | maxDets=100 ] = 0.292\n",
+ " Average Precision (AP) @[ IoU=0.50:0.95 | area= small | maxDets=100 ] = 0.026\n",
+ " Average Precision (AP) @[ IoU=0.50:0.95 | area=medium | maxDets=100 ] = 0.131\n",
+ " Average Precision (AP) @[ IoU=0.50:0.95 | area= large | maxDets=100 ] = 0.309\n",
+ " Average Recall (AR) @[ IoU=0.50:0.95 | area= all | maxDets= 1 ] = 0.284\n",
+ " Average Recall (AR) @[ IoU=0.50:0.95 | area= all | maxDets= 10 ] = 0.366\n",
+ " Average Recall (AR) @[ IoU=0.50:0.95 | area= all | maxDets=100 ] = 0.369\n",
+ " Average Recall (AR) @[ IoU=0.50:0.95 | area= small | maxDets=100 ] = 0.052\n",
+ " Average Recall (AR) @[ IoU=0.50:0.95 | area=medium | maxDets=100 ] = 0.204\n",
+ " Average Recall (AR) @[ IoU=0.50:0.95 | area= large | maxDets=100 ] = 0.416\n",
+ "4138/4138 [==============================] - 604s 146ms/step - loss: 0.8657 - box_loss: 0.7976 - class_loss: 0.0681 - val_loss: 1.2801 - val_box_loss: 1.1996 - val_class_loss: 0.0806 - val_AP: 0.2638 - val_AP50: 0.3863 - val_AP75: 0.2921 - val_APs: 0.0264 - val_APm: 0.1312 - val_APl: 0.3094 - val_ARmax1: 0.2841 - val_ARmax10: 0.3660 - val_ARmax100: 0.3688 - val_ARs: 0.0525 - val_ARm: 0.2043 - val_ARl: 0.4160\n",
+ "Epoch 37/120\n",
+ "1238/1238 [==============================] - 111s 89ms/step\n",
+ "creating index...\n",
+ "index created!\n",
+ "creating index...\n",
+ "index created!\n",
+ "Running per image evaluation...\n",
+ "Evaluate annotation type *bbox*\n",
+ "DONE (t=5.28s).\n",
+ "Accumulating evaluation results...\n",
+ "DONE (t=1.01s).\n",
+ " Average Precision (AP) @[ IoU=0.50:0.95 | area= all | maxDets=100 ] = 0.270\n",
+ " Average Precision (AP) @[ IoU=0.50 | area= all | maxDets=100 ] = 0.394\n",
+ " Average Precision (AP) @[ IoU=0.75 | area= all | maxDets=100 ] = 0.295\n",
+ " Average Precision (AP) @[ IoU=0.50:0.95 | area= small | maxDets=100 ] = 0.026\n",
+ " Average Precision (AP) @[ IoU=0.50:0.95 | area=medium | maxDets=100 ] = 0.130\n",
+ " Average Precision (AP) @[ IoU=0.50:0.95 | area= large | maxDets=100 ] = 0.319\n",
+ " Average Recall (AR) @[ IoU=0.50:0.95 | area= all | maxDets= 1 ] = 0.293\n",
+ " Average Recall (AR) @[ IoU=0.50:0.95 | area= all | maxDets= 10 ] = 0.375\n",
+ " Average Recall (AR) @[ IoU=0.50:0.95 | area= all | maxDets=100 ] = 0.377\n",
+ " Average Recall (AR) @[ IoU=0.50:0.95 | area= small | maxDets=100 ] = 0.049\n",
+ " Average Recall (AR) @[ IoU=0.50:0.95 | area=medium | maxDets=100 ] = 0.188\n",
+ " Average Recall (AR) @[ IoU=0.50:0.95 | area= large | maxDets=100 ] = 0.429\n",
+ "4138/4138 [==============================] - 604s 146ms/step - loss: 0.8567 - box_loss: 0.7897 - class_loss: 0.0669 - val_loss: 1.2754 - val_box_loss: 1.1958 - val_class_loss: 0.0796 - val_AP: 0.2705 - val_AP50: 0.3944 - val_AP75: 0.2952 - val_APs: 0.0261 - val_APm: 0.1305 - val_APl: 0.3192 - val_ARmax1: 0.2927 - val_ARmax10: 0.3745 - val_ARmax100: 0.3773 - val_ARs: 0.0494 - val_ARm: 0.1877 - val_ARl: 0.4288\n",
+ "Epoch 38/120\n",
+ "1238/1238 [==============================] - 111s 89ms/step\n",
+ "creating index...\n",
+ "index created!\n",
+ "creating index...\n",
+ "index created!\n",
+ "Running per image evaluation...\n",
+ "Evaluate annotation type *bbox*\n",
+ "DONE (t=4.65s).\n",
+ "Accumulating evaluation results...\n",
+ "DONE (t=1.01s).\n",
+ " Average Precision (AP) @[ IoU=0.50:0.95 | area= all | maxDets=100 ] = 0.265\n",
+ " Average Precision (AP) @[ IoU=0.50 | area= all | maxDets=100 ] = 0.390\n",
+ " Average Precision (AP) @[ IoU=0.75 | area= all | maxDets=100 ] = 0.288\n",
+ " Average Precision (AP) @[ IoU=0.50:0.95 | area= small | maxDets=100 ] = 0.027\n",
+ " Average Precision (AP) @[ IoU=0.50:0.95 | area=medium | maxDets=100 ] = 0.131\n",
+ " Average Precision (AP) @[ IoU=0.50:0.95 | area= large | maxDets=100 ] = 0.310\n",
+ " Average Recall (AR) @[ IoU=0.50:0.95 | area= all | maxDets= 1 ] = 0.288\n",
+ " Average Recall (AR) @[ IoU=0.50:0.95 | area= all | maxDets= 10 ] = 0.368\n",
+ " Average Recall (AR) @[ IoU=0.50:0.95 | area= all | maxDets=100 ] = 0.371\n",
+ " Average Recall (AR) @[ IoU=0.50:0.95 | area= small | maxDets=100 ] = 0.051\n",
+ " Average Recall (AR) @[ IoU=0.50:0.95 | area=medium | maxDets=100 ] = 0.193\n",
+ " Average Recall (AR) @[ IoU=0.50:0.95 | area= large | maxDets=100 ] = 0.417\n",
+ "4138/4138 [==============================] - 604s 146ms/step - loss: 0.8617 - box_loss: 0.7948 - class_loss: 0.0668 - val_loss: 1.2780 - val_box_loss: 1.1982 - val_class_loss: 0.0798 - val_AP: 0.2647 - val_AP50: 0.3895 - val_AP75: 0.2877 - val_APs: 0.0271 - val_APm: 0.1308 - val_APl: 0.3099 - val_ARmax1: 0.2877 - val_ARmax10: 0.3683 - val_ARmax100: 0.3712 - val_ARs: 0.0512 - val_ARm: 0.1928 - val_ARl: 0.4172\n",
+ "Epoch 39/120\n",
+ "1238/1238 [==============================] - 110s 89ms/step\n",
+ "creating index...\n",
+ "index created!\n",
+ "creating index...\n",
+ "index created!\n",
+ "Running per image evaluation...\n",
+ "Evaluate annotation type *bbox*\n",
+ "DONE (t=4.67s).\n",
+ "Accumulating evaluation results...\n",
+ "DONE (t=1.01s).\n",
+ " Average Precision (AP) @[ IoU=0.50:0.95 | area= all | maxDets=100 ] = 0.278\n",
+ " Average Precision (AP) @[ IoU=0.50 | area= all | maxDets=100 ] = 0.408\n",
+ " Average Precision (AP) @[ IoU=0.75 | area= all | maxDets=100 ] = 0.301\n",
+ " Average Precision (AP) @[ IoU=0.50:0.95 | area= small | maxDets=100 ] = 0.032\n",
+ " Average Precision (AP) @[ IoU=0.50:0.95 | area=medium | maxDets=100 ] = 0.137\n",
+ " Average Precision (AP) @[ IoU=0.50:0.95 | area= large | maxDets=100 ] = 0.326\n",
+ " Average Recall (AR) @[ IoU=0.50:0.95 | area= all | maxDets= 1 ] = 0.302\n",
+ " Average Recall (AR) @[ IoU=0.50:0.95 | area= all | maxDets= 10 ] = 0.386\n",
+ " Average Recall (AR) @[ IoU=0.50:0.95 | area= all | maxDets=100 ] = 0.389\n",
+ " Average Recall (AR) @[ IoU=0.50:0.95 | area= small | maxDets=100 ] = 0.059\n",
+ " Average Recall (AR) @[ IoU=0.50:0.95 | area=medium | maxDets=100 ] = 0.220\n",
+ " Average Recall (AR) @[ IoU=0.50:0.95 | area= large | maxDets=100 ] = 0.439\n",
+ "4138/4138 [==============================] - 605s 146ms/step - loss: 0.8383 - box_loss: 0.7730 - class_loss: 0.0653 - val_loss: 1.2666 - val_box_loss: 1.1879 - val_class_loss: 0.0787 - val_AP: 0.2784 - val_AP50: 0.4080 - val_AP75: 0.3013 - val_APs: 0.0319 - val_APm: 0.1374 - val_APl: 0.3264 - val_ARmax1: 0.3018 - val_ARmax10: 0.3864 - val_ARmax100: 0.3893 - val_ARs: 0.0592 - val_ARm: 0.2203 - val_ARl: 0.4393\n",
+ "Epoch 40/120\n",
+ "1238/1238 [==============================] - 111s 89ms/step\n",
+ "creating index...\n",
+ "index created!\n",
+ "creating index...\n",
+ "index created!\n",
+ "Running per image evaluation...\n",
+ "Evaluate annotation type *bbox*\n",
+ "DONE (t=4.68s).\n",
+ "Accumulating evaluation results...\n",
+ "DONE (t=1.01s).\n",
+ " Average Precision (AP) @[ IoU=0.50:0.95 | area= all | maxDets=100 ] = 0.275\n",
+ " Average Precision (AP) @[ IoU=0.50 | area= all | maxDets=100 ] = 0.405\n",
+ " Average Precision (AP) @[ IoU=0.75 | area= all | maxDets=100 ] = 0.298\n",
+ " Average Precision (AP) @[ IoU=0.50:0.95 | area= small | maxDets=100 ] = 0.030\n",
+ " Average Precision (AP) @[ IoU=0.50:0.95 | area=medium | maxDets=100 ] = 0.138\n",
+ " Average Precision (AP) @[ IoU=0.50:0.95 | area= large | maxDets=100 ] = 0.322\n",
+ " Average Recall (AR) @[ IoU=0.50:0.95 | area= all | maxDets= 1 ] = 0.295\n",
+ " Average Recall (AR) @[ IoU=0.50:0.95 | area= all | maxDets= 10 ] = 0.381\n",
+ " Average Recall (AR) @[ IoU=0.50:0.95 | area= all | maxDets=100 ] = 0.384\n",
+ " Average Recall (AR) @[ IoU=0.50:0.95 | area= small | maxDets=100 ] = 0.056\n",
+ " Average Recall (AR) @[ IoU=0.50:0.95 | area=medium | maxDets=100 ] = 0.220\n",
+ " Average Recall (AR) @[ IoU=0.50:0.95 | area= large | maxDets=100 ] = 0.432\n",
+ "4138/4138 [==============================] - 604s 146ms/step - loss: 0.8355 - box_loss: 0.7700 - class_loss: 0.0654 - val_loss: 1.2627 - val_box_loss: 1.1846 - val_class_loss: 0.0781 - val_AP: 0.2753 - val_AP50: 0.4052 - val_AP75: 0.2975 - val_APs: 0.0302 - val_APm: 0.1379 - val_APl: 0.3224 - val_ARmax1: 0.2946 - val_ARmax10: 0.3813 - val_ARmax100: 0.3843 - val_ARs: 0.0557 - val_ARm: 0.2196 - val_ARl: 0.4316\n",
+ "Epoch 41/120\n",
+ "1238/1238 [==============================] - 111s 89ms/step\n",
+ "creating index...\n",
+ "index created!\n",
+ "creating index...\n",
+ "index created!\n",
+ "Running per image evaluation...\n",
+ "Evaluate annotation type *bbox*\n",
+ "DONE (t=4.76s).\n",
+ "Accumulating evaluation results...\n",
+ "DONE (t=1.03s).\n",
+ " Average Precision (AP) @[ IoU=0.50:0.95 | area= all | maxDets=100 ] = 0.280\n",
+ " Average Precision (AP) @[ IoU=0.50 | area= all | maxDets=100 ] = 0.408\n",
+ " Average Precision (AP) @[ IoU=0.75 | area= all | maxDets=100 ] = 0.305\n",
+ " Average Precision (AP) @[ IoU=0.50:0.95 | area= small | maxDets=100 ] = 0.028\n",
+ " Average Precision (AP) @[ IoU=0.50:0.95 | area=medium | maxDets=100 ] = 0.136\n",
+ " Average Precision (AP) @[ IoU=0.50:0.95 | area= large | maxDets=100 ] = 0.327\n",
+ " Average Recall (AR) @[ IoU=0.50:0.95 | area= all | maxDets= 1 ] = 0.298\n",
+ " Average Recall (AR) @[ IoU=0.50:0.95 | area= all | maxDets= 10 ] = 0.386\n",
+ " Average Recall (AR) @[ IoU=0.50:0.95 | area= all | maxDets=100 ] = 0.388\n",
+ " Average Recall (AR) @[ IoU=0.50:0.95 | area= small | maxDets=100 ] = 0.054\n",
+ " Average Recall (AR) @[ IoU=0.50:0.95 | area=medium | maxDets=100 ] = 0.201\n",
+ " Average Recall (AR) @[ IoU=0.50:0.95 | area= large | maxDets=100 ] = 0.439\n",
+ "4138/4138 [==============================] - 605s 146ms/step - loss: 0.8167 - box_loss: 0.7530 - class_loss: 0.0637 - val_loss: 1.2644 - val_box_loss: 1.1860 - val_class_loss: 0.0784 - val_AP: 0.2801 - val_AP50: 0.4076 - val_AP75: 0.3049 - val_APs: 0.0284 - val_APm: 0.1360 - val_APl: 0.3274 - val_ARmax1: 0.2981 - val_ARmax10: 0.3858 - val_ARmax100: 0.3884 - val_ARs: 0.0537 - val_ARm: 0.2014 - val_ARl: 0.4390\n",
+ "Epoch 42/120\n",
+ "1238/1238 [==============================] - 111s 89ms/step\n",
+ "creating index...\n",
+ "index created!\n",
+ "creating index...\n",
+ "index created!\n",
+ "Running per image evaluation...\n",
+ "Evaluate annotation type *bbox*\n",
+ "DONE (t=4.71s).\n",
+ "Accumulating evaluation results...\n",
+ "DONE (t=1.03s).\n",
+ " Average Precision (AP) @[ IoU=0.50:0.95 | area= all | maxDets=100 ] = 0.280\n",
+ " Average Precision (AP) @[ IoU=0.50 | area= all | maxDets=100 ] = 0.411\n",
+ " Average Precision (AP) @[ IoU=0.75 | area= all | maxDets=100 ] = 0.305\n",
+ " Average Precision (AP) @[ IoU=0.50:0.95 | area= small | maxDets=100 ] = 0.029\n",
+ " Average Precision (AP) @[ IoU=0.50:0.95 | area=medium | maxDets=100 ] = 0.145\n",
+ " Average Precision (AP) @[ IoU=0.50:0.95 | area= large | maxDets=100 ] = 0.325\n",
+ " Average Recall (AR) @[ IoU=0.50:0.95 | area= all | maxDets= 1 ] = 0.304\n",
+ " Average Recall (AR) @[ IoU=0.50:0.95 | area= all | maxDets= 10 ] = 0.393\n",
+ " Average Recall (AR) @[ IoU=0.50:0.95 | area= all | maxDets=100 ] = 0.396\n",
+ " Average Recall (AR) @[ IoU=0.50:0.95 | area= small | maxDets=100 ] = 0.053\n",
+ " Average Recall (AR) @[ IoU=0.50:0.95 | area=medium | maxDets=100 ] = 0.227\n",
+ " Average Recall (AR) @[ IoU=0.50:0.95 | area= large | maxDets=100 ] = 0.443\n",
+ "4138/4138 [==============================] - 604s 146ms/step - loss: 0.8121 - box_loss: 0.7488 - class_loss: 0.0632 - val_loss: 1.2774 - val_box_loss: 1.1994 - val_class_loss: 0.0780 - val_AP: 0.2802 - val_AP50: 0.4109 - val_AP75: 0.3048 - val_APs: 0.0288 - val_APm: 0.1448 - val_APl: 0.3250 - val_ARmax1: 0.3042 - val_ARmax10: 0.3932 - val_ARmax100: 0.3957 - val_ARs: 0.0531 - val_ARm: 0.2267 - val_ARl: 0.4429\n",
+ "Epoch 43/120\n",
+ "1238/1238 [==============================] - 111s 89ms/step\n",
+ "creating index...\n",
+ "index created!\n",
+ "creating index...\n",
+ "index created!\n",
+ "Running per image evaluation...\n",
+ "Evaluate annotation type *bbox*\n",
+ "DONE (t=4.68s).\n",
+ "Accumulating evaluation results...\n",
+ "DONE (t=1.00s).\n",
+ " Average Precision (AP) @[ IoU=0.50:0.95 | area= all | maxDets=100 ] = 0.283\n",
+ " Average Precision (AP) @[ IoU=0.50 | area= all | maxDets=100 ] = 0.411\n",
+ " Average Precision (AP) @[ IoU=0.75 | area= all | maxDets=100 ] = 0.311\n",
+ " Average Precision (AP) @[ IoU=0.50:0.95 | area= small | maxDets=100 ] = 0.033\n",
+ " Average Precision (AP) @[ IoU=0.50:0.95 | area=medium | maxDets=100 ] = 0.143\n",
+ " Average Precision (AP) @[ IoU=0.50:0.95 | area= large | maxDets=100 ] = 0.330\n",
+ " Average Recall (AR) @[ IoU=0.50:0.95 | area= all | maxDets= 1 ] = 0.303\n",
+ " Average Recall (AR) @[ IoU=0.50:0.95 | area= all | maxDets= 10 ] = 0.388\n",
+ " Average Recall (AR) @[ IoU=0.50:0.95 | area= all | maxDets=100 ] = 0.391\n",
+ " Average Recall (AR) @[ IoU=0.50:0.95 | area= small | maxDets=100 ] = 0.057\n",
+ " Average Recall (AR) @[ IoU=0.50:0.95 | area=medium | maxDets=100 ] = 0.201\n",
+ " Average Recall (AR) @[ IoU=0.50:0.95 | area= large | maxDets=100 ] = 0.441\n",
+ "4138/4138 [==============================] - 604s 146ms/step - loss: 0.7997 - box_loss: 0.7371 - class_loss: 0.0626 - val_loss: 1.2725 - val_box_loss: 1.1946 - val_class_loss: 0.0779 - val_AP: 0.2833 - val_AP50: 0.4112 - val_AP75: 0.3107 - val_APs: 0.0331 - val_APm: 0.1429 - val_APl: 0.3305 - val_ARmax1: 0.3029 - val_ARmax10: 0.3885 - val_ARmax100: 0.3910 - val_ARs: 0.0567 - val_ARm: 0.2009 - val_ARl: 0.4407\n",
+ "Epoch 44/120\n",
+ "1238/1238 [==============================] - 111s 89ms/step\n",
+ "creating index...\n",
+ "index created!\n",
+ "creating index...\n",
+ "index created!\n",
+ "Running per image evaluation...\n",
+ "Evaluate annotation type *bbox*\n",
+ "DONE (t=4.77s).\n",
+ "Accumulating evaluation results...\n",
+ "DONE (t=1.03s).\n",
+ " Average Precision (AP) @[ IoU=0.50:0.95 | area= all | maxDets=100 ] = 0.290\n",
+ " Average Precision (AP) @[ IoU=0.50 | area= all | maxDets=100 ] = 0.424\n",
+ " Average Precision (AP) @[ IoU=0.75 | area= all | maxDets=100 ] = 0.314\n",
+ " Average Precision (AP) @[ IoU=0.50:0.95 | area= small | maxDets=100 ] = 0.036\n",
+ " Average Precision (AP) @[ IoU=0.50:0.95 | area=medium | maxDets=100 ] = 0.148\n",
+ " Average Precision (AP) @[ IoU=0.50:0.95 | area= large | maxDets=100 ] = 0.338\n",
+ " Average Recall (AR) @[ IoU=0.50:0.95 | area= all | maxDets= 1 ] = 0.309\n",
+ " Average Recall (AR) @[ IoU=0.50:0.95 | area= all | maxDets= 10 ] = 0.397\n",
+ " Average Recall (AR) @[ IoU=0.50:0.95 | area= all | maxDets=100 ] = 0.400\n",
+ " Average Recall (AR) @[ IoU=0.50:0.95 | area= small | maxDets=100 ] = 0.064\n",
+ " Average Recall (AR) @[ IoU=0.50:0.95 | area=medium | maxDets=100 ] = 0.230\n",
+ " Average Recall (AR) @[ IoU=0.50:0.95 | area= large | maxDets=100 ] = 0.450\n",
+ "4138/4138 [==============================] - 605s 146ms/step - loss: 0.7893 - box_loss: 0.7278 - class_loss: 0.0614 - val_loss: 1.2544 - val_box_loss: 1.1779 - val_class_loss: 0.0765 - val_AP: 0.2903 - val_AP50: 0.4244 - val_AP75: 0.3143 - val_APs: 0.0358 - val_APm: 0.1483 - val_APl: 0.3378 - val_ARmax1: 0.3086 - val_ARmax10: 0.3971 - val_ARmax100: 0.4001 - val_ARs: 0.0640 - val_ARm: 0.2296 - val_ARl: 0.4495\n",
+ "Epoch 45/120\n",
+ "1238/1238 [==============================] - 111s 89ms/step\n",
+ "creating index...\n",
+ "index created!\n",
+ "creating index...\n",
+ "index created!\n",
+ "Running per image evaluation...\n",
+ "Evaluate annotation type *bbox*\n",
+ "DONE (t=4.70s).\n",
+ "Accumulating evaluation results...\n",
+ "DONE (t=1.00s).\n",
+ " Average Precision (AP) @[ IoU=0.50:0.95 | area= all | maxDets=100 ] = 0.290\n",
+ " Average Precision (AP) @[ IoU=0.50 | area= all | maxDets=100 ] = 0.423\n",
+ " Average Precision (AP) @[ IoU=0.75 | area= all | maxDets=100 ] = 0.316\n",
+ " Average Precision (AP) @[ IoU=0.50:0.95 | area= small | maxDets=100 ] = 0.029\n",
+ " Average Precision (AP) @[ IoU=0.50:0.95 | area=medium | maxDets=100 ] = 0.139\n",
+ " Average Precision (AP) @[ IoU=0.50:0.95 | area= large | maxDets=100 ] = 0.341\n",
+ " Average Recall (AR) @[ IoU=0.50:0.95 | area= all | maxDets= 1 ] = 0.308\n",
+ " Average Recall (AR) @[ IoU=0.50:0.95 | area= all | maxDets= 10 ] = 0.398\n",
+ " Average Recall (AR) @[ IoU=0.50:0.95 | area= all | maxDets=100 ] = 0.401\n",
+ " Average Recall (AR) @[ IoU=0.50:0.95 | area= small | maxDets=100 ] = 0.055\n",
+ " Average Recall (AR) @[ IoU=0.50:0.95 | area=medium | maxDets=100 ] = 0.224\n",
+ " Average Recall (AR) @[ IoU=0.50:0.95 | area= large | maxDets=100 ] = 0.454\n",
+ "4138/4138 [==============================] - 604s 146ms/step - loss: 0.7990 - box_loss: 0.7371 - class_loss: 0.0619 - val_loss: 1.2662 - val_box_loss: 1.1904 - val_class_loss: 0.0758 - val_AP: 0.2895 - val_AP50: 0.4227 - val_AP75: 0.3165 - val_APs: 0.0289 - val_APm: 0.1391 - val_APl: 0.3410 - val_ARmax1: 0.3084 - val_ARmax10: 0.3979 - val_ARmax100: 0.4006 - val_ARs: 0.0549 - val_ARm: 0.2241 - val_ARl: 0.4542\n",
+ "Epoch 46/120\n",
+ "1238/1238 [==============================] - 111s 89ms/step\n",
+ "creating index...\n",
+ "index created!\n",
+ "creating index...\n",
+ "index created!\n",
+ "Running per image evaluation...\n",
+ "Evaluate annotation type *bbox*\n",
+ "DONE (t=4.70s).\n",
+ "Accumulating evaluation results...\n",
+ "DONE (t=1.01s).\n",
+ " Average Precision (AP) @[ IoU=0.50:0.95 | area= all | maxDets=100 ] = 0.296\n",
+ " Average Precision (AP) @[ IoU=0.50 | area= all | maxDets=100 ] = 0.426\n",
+ " Average Precision (AP) @[ IoU=0.75 | area= all | maxDets=100 ] = 0.326\n",
+ " Average Precision (AP) @[ IoU=0.50:0.95 | area= small | maxDets=100 ] = 0.034\n",
+ " Average Precision (AP) @[ IoU=0.50:0.95 | area=medium | maxDets=100 ] = 0.142\n",
+ " Average Precision (AP) @[ IoU=0.50:0.95 | area= large | maxDets=100 ] = 0.350\n",
+ " Average Recall (AR) @[ IoU=0.50:0.95 | area= all | maxDets= 1 ] = 0.310\n",
+ " Average Recall (AR) @[ IoU=0.50:0.95 | area= all | maxDets= 10 ] = 0.402\n",
+ " Average Recall (AR) @[ IoU=0.50:0.95 | area= all | maxDets=100 ] = 0.405\n",
+ " Average Recall (AR) @[ IoU=0.50:0.95 | area= small | maxDets=100 ] = 0.059\n",
+ " Average Recall (AR) @[ IoU=0.50:0.95 | area=medium | maxDets=100 ] = 0.208\n",
+ " Average Recall (AR) @[ IoU=0.50:0.95 | area= large | maxDets=100 ] = 0.461\n",
+ "4138/4138 [==============================] - 605s 146ms/step - loss: 0.7900 - box_loss: 0.7289 - class_loss: 0.0612 - val_loss: 1.2528 - val_box_loss: 1.1772 - val_class_loss: 0.0756 - val_AP: 0.2959 - val_AP50: 0.4262 - val_AP75: 0.3258 - val_APs: 0.0342 - val_APm: 0.1417 - val_APl: 0.3502 - val_ARmax1: 0.3102 - val_ARmax10: 0.4024 - val_ARmax100: 0.4052 - val_ARs: 0.0591 - val_ARm: 0.2084 - val_ARl: 0.4609\n",
+ "Epoch 47/120\n",
+ "1238/1238 [==============================] - 111s 89ms/step\n",
+ "creating index...\n",
+ "index created!\n",
+ "creating index...\n",
+ "index created!\n",
+ "Running per image evaluation...\n",
+ "Evaluate annotation type *bbox*\n",
+ "DONE (t=4.71s).\n",
+ "Accumulating evaluation results...\n",
+ "DONE (t=1.00s).\n",
+ " Average Precision (AP) @[ IoU=0.50:0.95 | area= all | maxDets=100 ] = 0.294\n",
+ " Average Precision (AP) @[ IoU=0.50 | area= all | maxDets=100 ] = 0.427\n",
+ " Average Precision (AP) @[ IoU=0.75 | area= all | maxDets=100 ] = 0.319\n",
+ " Average Precision (AP) @[ IoU=0.50:0.95 | area= small | maxDets=100 ] = 0.028\n",
+ " Average Precision (AP) @[ IoU=0.50:0.95 | area=medium | maxDets=100 ] = 0.147\n",
+ " Average Precision (AP) @[ IoU=0.50:0.95 | area= large | maxDets=100 ] = 0.347\n",
+ " Average Recall (AR) @[ IoU=0.50:0.95 | area= all | maxDets= 1 ] = 0.311\n",
+ " Average Recall (AR) @[ IoU=0.50:0.95 | area= all | maxDets= 10 ] = 0.403\n",
+ " Average Recall (AR) @[ IoU=0.50:0.95 | area= all | maxDets=100 ] = 0.406\n",
+ " Average Recall (AR) @[ IoU=0.50:0.95 | area= small | maxDets=100 ] = 0.049\n",
+ " Average Recall (AR) @[ IoU=0.50:0.95 | area=medium | maxDets=100 ] = 0.215\n",
+ " Average Recall (AR) @[ IoU=0.50:0.95 | area= large | maxDets=100 ] = 0.463\n",
+ "4138/4138 [==============================] - 605s 146ms/step - loss: 0.7789 - box_loss: 0.7188 - class_loss: 0.0602 - val_loss: 1.2567 - val_box_loss: 1.1812 - val_class_loss: 0.0756 - val_AP: 0.2944 - val_AP50: 0.4271 - val_AP75: 0.3193 - val_APs: 0.0284 - val_APm: 0.1468 - val_APl: 0.3470 - val_ARmax1: 0.3115 - val_ARmax10: 0.4032 - val_ARmax100: 0.4064 - val_ARs: 0.0493 - val_ARm: 0.2145 - val_ARl: 0.4629\n",
+ "Epoch 48/120\n",
+ "1238/1238 [==============================] - 111s 89ms/step\n",
+ "creating index...\n",
+ "index created!\n",
+ "creating index...\n",
+ "index created!\n",
+ "Running per image evaluation...\n",
+ "Evaluate annotation type *bbox*\n",
+ "DONE (t=4.77s).\n",
+ "Accumulating evaluation results...\n",
+ "DONE (t=1.02s).\n",
+ " Average Precision (AP) @[ IoU=0.50:0.95 | area= all | maxDets=100 ] = 0.297\n",
+ " Average Precision (AP) @[ IoU=0.50 | area= all | maxDets=100 ] = 0.430\n",
+ " Average Precision (AP) @[ IoU=0.75 | area= all | maxDets=100 ] = 0.321\n",
+ " Average Precision (AP) @[ IoU=0.50:0.95 | area= small | maxDets=100 ] = 0.031\n",
+ " Average Precision (AP) @[ IoU=0.50:0.95 | area=medium | maxDets=100 ] = 0.150\n",
+ " Average Precision (AP) @[ IoU=0.50:0.95 | area= large | maxDets=100 ] = 0.349\n",
+ " Average Recall (AR) @[ IoU=0.50:0.95 | area= all | maxDets= 1 ] = 0.311\n",
+ " Average Recall (AR) @[ IoU=0.50:0.95 | area= all | maxDets= 10 ] = 0.398\n",
+ " Average Recall (AR) @[ IoU=0.50:0.95 | area= all | maxDets=100 ] = 0.401\n",
+ " Average Recall (AR) @[ IoU=0.50:0.95 | area= small | maxDets=100 ] = 0.060\n",
+ " Average Recall (AR) @[ IoU=0.50:0.95 | area=medium | maxDets=100 ] = 0.237\n",
+ " Average Recall (AR) @[ IoU=0.50:0.95 | area= large | maxDets=100 ] = 0.453\n",
+ "4138/4138 [==============================] - 605s 146ms/step - loss: 0.7607 - box_loss: 0.7021 - class_loss: 0.0586 - val_loss: 1.2492 - val_box_loss: 1.1740 - val_class_loss: 0.0752 - val_AP: 0.2970 - val_AP50: 0.4296 - val_AP75: 0.3210 - val_APs: 0.0312 - val_APm: 0.1496 - val_APl: 0.3494 - val_ARmax1: 0.3109 - val_ARmax10: 0.3985 - val_ARmax100: 0.4011 - val_ARs: 0.0601 - val_ARm: 0.2369 - val_ARl: 0.4535\n",
+ "Epoch 49/120\n",
+ "1238/1238 [==============================] - 111s 89ms/step\n",
+ "creating index...\n",
+ "index created!\n",
+ "creating index...\n",
+ "index created!\n",
+ "Running per image evaluation...\n",
+ "Evaluate annotation type *bbox*\n",
+ "DONE (t=5.28s).\n",
+ "Accumulating evaluation results...\n",
+ "DONE (t=1.01s).\n",
+ " Average Precision (AP) @[ IoU=0.50:0.95 | area= all | maxDets=100 ] = 0.299\n",
+ " Average Precision (AP) @[ IoU=0.50 | area= all | maxDets=100 ] = 0.433\n",
+ " Average Precision (AP) @[ IoU=0.75 | area= all | maxDets=100 ] = 0.326\n",
+ " Average Precision (AP) @[ IoU=0.50:0.95 | area= small | maxDets=100 ] = 0.043\n",
+ " Average Precision (AP) @[ IoU=0.50:0.95 | area=medium | maxDets=100 ] = 0.149\n",
+ " Average Precision (AP) @[ IoU=0.50:0.95 | area= large | maxDets=100 ] = 0.350\n",
+ " Average Recall (AR) @[ IoU=0.50:0.95 | area= all | maxDets= 1 ] = 0.316\n",
+ " Average Recall (AR) @[ IoU=0.50:0.95 | area= all | maxDets= 10 ] = 0.406\n",
+ " Average Recall (AR) @[ IoU=0.50:0.95 | area= all | maxDets=100 ] = 0.409\n",
+ " Average Recall (AR) @[ IoU=0.50:0.95 | area= small | maxDets=100 ] = 0.070\n",
+ " Average Recall (AR) @[ IoU=0.50:0.95 | area=medium | maxDets=100 ] = 0.231\n",
+ " Average Recall (AR) @[ IoU=0.50:0.95 | area= large | maxDets=100 ] = 0.463\n",
+ "4138/4138 [==============================] - 605s 146ms/step - loss: 0.7512 - box_loss: 0.6928 - class_loss: 0.0584 - val_loss: 1.2506 - val_box_loss: 1.1753 - val_class_loss: 0.0753 - val_AP: 0.2995 - val_AP50: 0.4335 - val_AP75: 0.3263 - val_APs: 0.0429 - val_APm: 0.1490 - val_APl: 0.3504 - val_ARmax1: 0.3162 - val_ARmax10: 0.4063 - val_ARmax100: 0.4092 - val_ARs: 0.0701 - val_ARm: 0.2315 - val_ARl: 0.4631\n",
+ "Epoch 50/120\n",
+ "1238/1238 [==============================] - 111s 89ms/step\n",
+ "creating index...\n",
+ "index created!\n",
+ "creating index...\n",
+ "index created!\n",
+ "Running per image evaluation...\n",
+ "Evaluate annotation type *bbox*\n",
+ "DONE (t=4.76s).\n",
+ "Accumulating evaluation results...\n",
+ "DONE (t=1.04s).\n",
+ " Average Precision (AP) @[ IoU=0.50:0.95 | area= all | maxDets=100 ] = 0.300\n",
+ " Average Precision (AP) @[ IoU=0.50 | area= all | maxDets=100 ] = 0.433\n",
+ " Average Precision (AP) @[ IoU=0.75 | area= all | maxDets=100 ] = 0.325\n",
+ " Average Precision (AP) @[ IoU=0.50:0.95 | area= small | maxDets=100 ] = 0.035\n",
+ " Average Precision (AP) @[ IoU=0.50:0.95 | area=medium | maxDets=100 ] = 0.145\n",
+ " Average Precision (AP) @[ IoU=0.50:0.95 | area= large | maxDets=100 ] = 0.349\n",
+ " Average Recall (AR) @[ IoU=0.50:0.95 | area= all | maxDets= 1 ] = 0.309\n",
+ " Average Recall (AR) @[ IoU=0.50:0.95 | area= all | maxDets= 10 ] = 0.403\n",
+ " Average Recall (AR) @[ IoU=0.50:0.95 | area= all | maxDets=100 ] = 0.407\n",
+ " Average Recall (AR) @[ IoU=0.50:0.95 | area= small | maxDets=100 ] = 0.066\n",
+ " Average Recall (AR) @[ IoU=0.50:0.95 | area=medium | maxDets=100 ] = 0.233\n",
+ " Average Recall (AR) @[ IoU=0.50:0.95 | area= large | maxDets=100 ] = 0.456\n",
+ "4138/4138 [==============================] - 604s 146ms/step - loss: 0.7524 - box_loss: 0.6940 - class_loss: 0.0584 - val_loss: 1.2530 - val_box_loss: 1.1776 - val_class_loss: 0.0754 - val_AP: 0.2998 - val_AP50: 0.4334 - val_AP75: 0.3254 - val_APs: 0.0348 - val_APm: 0.1449 - val_APl: 0.3493 - val_ARmax1: 0.3089 - val_ARmax10: 0.4035 - val_ARmax100: 0.4067 - val_ARs: 0.0658 - val_ARm: 0.2331 - val_ARl: 0.4563\n",
+ "Epoch 51/120\n",
+ "1238/1238 [==============================] - 110s 89ms/step\n",
+ "creating index...\n",
+ "index created!\n",
+ "creating index...\n",
+ "index created!\n",
+ "Running per image evaluation...\n",
+ "Evaluate annotation type *bbox*\n",
+ "DONE (t=5.34s).\n",
+ "Accumulating evaluation results...\n",
+ "DONE (t=1.01s).\n",
+ " Average Precision (AP) @[ IoU=0.50:0.95 | area= all | maxDets=100 ] = 0.308\n",
+ " Average Precision (AP) @[ IoU=0.50 | area= all | maxDets=100 ] = 0.445\n",
+ " Average Precision (AP) @[ IoU=0.75 | area= all | maxDets=100 ] = 0.335\n",
+ " Average Precision (AP) @[ IoU=0.50:0.95 | area= small | maxDets=100 ] = 0.038\n",
+ " Average Precision (AP) @[ IoU=0.50:0.95 | area=medium | maxDets=100 ] = 0.151\n",
+ " Average Precision (AP) @[ IoU=0.50:0.95 | area= large | maxDets=100 ] = 0.359\n",
+ " Average Recall (AR) @[ IoU=0.50:0.95 | area= all | maxDets= 1 ] = 0.320\n",
+ " Average Recall (AR) @[ IoU=0.50:0.95 | area= all | maxDets= 10 ] = 0.414\n",
+ " Average Recall (AR) @[ IoU=0.50:0.95 | area= all | maxDets=100 ] = 0.417\n",
+ " Average Recall (AR) @[ IoU=0.50:0.95 | area= small | maxDets=100 ] = 0.063\n",
+ " Average Recall (AR) @[ IoU=0.50:0.95 | area=medium | maxDets=100 ] = 0.239\n",
+ " Average Recall (AR) @[ IoU=0.50:0.95 | area= large | maxDets=100 ] = 0.468\n",
+ "4138/4138 [==============================] - 605s 146ms/step - loss: 0.7398 - box_loss: 0.6825 - class_loss: 0.0573 - val_loss: 1.2493 - val_box_loss: 1.1759 - val_class_loss: 0.0734 - val_AP: 0.3077 - val_AP50: 0.4453 - val_AP75: 0.3355 - val_APs: 0.0377 - val_APm: 0.1513 - val_APl: 0.3586 - val_ARmax1: 0.3196 - val_ARmax10: 0.4140 - val_ARmax100: 0.4170 - val_ARs: 0.0629 - val_ARm: 0.2392 - val_ARl: 0.4681\n",
+ "Epoch 52/120\n",
+ "1238/1238 [==============================] - 111s 89ms/step\n",
+ "creating index...\n",
+ "index created!\n",
+ "creating index...\n",
+ "index created!\n",
+ "Running per image evaluation...\n",
+ "Evaluate annotation type *bbox*\n",
+ "DONE (t=4.79s).\n",
+ "Accumulating evaluation results...\n",
+ "DONE (t=1.03s).\n",
+ " Average Precision (AP) @[ IoU=0.50:0.95 | area= all | maxDets=100 ] = 0.311\n",
+ " Average Precision (AP) @[ IoU=0.50 | area= all | maxDets=100 ] = 0.455\n",
+ " Average Precision (AP) @[ IoU=0.75 | area= all | maxDets=100 ] = 0.336\n",
+ " Average Precision (AP) @[ IoU=0.50:0.95 | area= small | maxDets=100 ] = 0.036\n",
+ " Average Precision (AP) @[ IoU=0.50:0.95 | area=medium | maxDets=100 ] = 0.154\n",
+ " Average Precision (AP) @[ IoU=0.50:0.95 | area= large | maxDets=100 ] = 0.362\n",
+ " Average Recall (AR) @[ IoU=0.50:0.95 | area= all | maxDets= 1 ] = 0.322\n",
+ " Average Recall (AR) @[ IoU=0.50:0.95 | area= all | maxDets= 10 ] = 0.420\n",
+ " Average Recall (AR) @[ IoU=0.50:0.95 | area= all | maxDets=100 ] = 0.423\n",
+ " Average Recall (AR) @[ IoU=0.50:0.95 | area= small | maxDets=100 ] = 0.063\n",
+ " Average Recall (AR) @[ IoU=0.50:0.95 | area=medium | maxDets=100 ] = 0.249\n",
+ " Average Recall (AR) @[ IoU=0.50:0.95 | area= large | maxDets=100 ] = 0.475\n",
+ "4138/4138 [==============================] - 605s 146ms/step - loss: 0.7419 - box_loss: 0.6849 - class_loss: 0.0571 - val_loss: 1.2458 - val_box_loss: 1.1725 - val_class_loss: 0.0734 - val_AP: 0.3109 - val_AP50: 0.4545 - val_AP75: 0.3357 - val_APs: 0.0356 - val_APm: 0.1545 - val_APl: 0.3621 - val_ARmax1: 0.3220 - val_ARmax10: 0.4198 - val_ARmax100: 0.4232 - val_ARs: 0.0633 - val_ARm: 0.2493 - val_ARl: 0.4754\n",
+ "Epoch 53/120\n",
+ "1238/1238 [==============================] - 111s 89ms/step\n",
+ "creating index...\n",
+ "index created!\n",
+ "creating index...\n",
+ "index created!\n",
+ "Running per image evaluation...\n",
+ "Evaluate annotation type *bbox*\n",
+ "DONE (t=4.73s).\n",
+ "Accumulating evaluation results...\n",
+ "DONE (t=1.02s).\n",
+ " Average Precision (AP) @[ IoU=0.50:0.95 | area= all | maxDets=100 ] = 0.301\n",
+ " Average Precision (AP) @[ IoU=0.50 | area= all | maxDets=100 ] = 0.438\n",
+ " Average Precision (AP) @[ IoU=0.75 | area= all | maxDets=100 ] = 0.325\n",
+ " Average Precision (AP) @[ IoU=0.50:0.95 | area= small | maxDets=100 ] = 0.030\n",
+ " Average Precision (AP) @[ IoU=0.50:0.95 | area=medium | maxDets=100 ] = 0.147\n",
+ " Average Precision (AP) @[ IoU=0.50:0.95 | area= large | maxDets=100 ] = 0.352\n",
+ " Average Recall (AR) @[ IoU=0.50:0.95 | area= all | maxDets= 1 ] = 0.315\n",
+ " Average Recall (AR) @[ IoU=0.50:0.95 | area= all | maxDets= 10 ] = 0.406\n",
+ " Average Recall (AR) @[ IoU=0.50:0.95 | area= all | maxDets=100 ] = 0.409\n",
+ " Average Recall (AR) @[ IoU=0.50:0.95 | area= small | maxDets=100 ] = 0.060\n",
+ " Average Recall (AR) @[ IoU=0.50:0.95 | area=medium | maxDets=100 ] = 0.240\n",
+ " Average Recall (AR) @[ IoU=0.50:0.95 | area= large | maxDets=100 ] = 0.460\n",
+ "4138/4138 [==============================] - 605s 146ms/step - loss: 0.7359 - box_loss: 0.6793 - class_loss: 0.0566 - val_loss: 1.2609 - val_box_loss: 1.1857 - val_class_loss: 0.0752 - val_AP: 0.3013 - val_AP50: 0.4378 - val_AP75: 0.3249 - val_APs: 0.0298 - val_APm: 0.1468 - val_APl: 0.3519 - val_ARmax1: 0.3147 - val_ARmax10: 0.4062 - val_ARmax100: 0.4090 - val_ARs: 0.0602 - val_ARm: 0.2396 - val_ARl: 0.4605\n",
+ "Epoch 54/120\n",
+ "1238/1238 [==============================] - 110s 89ms/step\n",
+ "creating index...\n",
+ "index created!\n",
+ "creating index...\n",
+ "index created!\n",
+ "Running per image evaluation...\n",
+ "Evaluate annotation type *bbox*\n",
+ "DONE (t=4.66s).\n",
+ "Accumulating evaluation results...\n",
+ "DONE (t=1.67s).\n",
+ " Average Precision (AP) @[ IoU=0.50:0.95 | area= all | maxDets=100 ] = 0.314\n",
+ " Average Precision (AP) @[ IoU=0.50 | area= all | maxDets=100 ] = 0.452\n",
+ " Average Precision (AP) @[ IoU=0.75 | area= all | maxDets=100 ] = 0.343\n",
+ " Average Precision (AP) @[ IoU=0.50:0.95 | area= small | maxDets=100 ] = 0.032\n",
+ " Average Precision (AP) @[ IoU=0.50:0.95 | area=medium | maxDets=100 ] = 0.156\n",
+ " Average Precision (AP) @[ IoU=0.50:0.95 | area= large | maxDets=100 ] = 0.369\n",
+ " Average Recall (AR) @[ IoU=0.50:0.95 | area= all | maxDets= 1 ] = 0.325\n",
+ " Average Recall (AR) @[ IoU=0.50:0.95 | area= all | maxDets= 10 ] = 0.419\n",
+ " Average Recall (AR) @[ IoU=0.50:0.95 | area= all | maxDets=100 ] = 0.421\n",
+ " Average Recall (AR) @[ IoU=0.50:0.95 | area= small | maxDets=100 ] = 0.064\n",
+ " Average Recall (AR) @[ IoU=0.50:0.95 | area=medium | maxDets=100 ] = 0.245\n",
+ " Average Recall (AR) @[ IoU=0.50:0.95 | area= large | maxDets=100 ] = 0.476\n",
+ "4138/4138 [==============================] - 604s 146ms/step - loss: 0.7176 - box_loss: 0.6620 - class_loss: 0.0556 - val_loss: 1.2498 - val_box_loss: 1.1762 - val_class_loss: 0.0737 - val_AP: 0.3144 - val_AP50: 0.4522 - val_AP75: 0.3426 - val_APs: 0.0323 - val_APm: 0.1565 - val_APl: 0.3690 - val_ARmax1: 0.3251 - val_ARmax10: 0.4188 - val_ARmax100: 0.4213 - val_ARs: 0.0638 - val_ARm: 0.2451 - val_ARl: 0.4764\n",
+ "Epoch 55/120\n",
+ "1238/1238 [==============================] - 111s 89ms/step\n",
+ "creating index...\n",
+ "index created!\n",
+ "creating index...\n",
+ "index created!\n",
+ "Running per image evaluation...\n",
+ "Evaluate annotation type *bbox*\n",
+ "DONE (t=4.78s).\n",
+ "Accumulating evaluation results...\n",
+ "DONE (t=1.04s).\n",
+ " Average Precision (AP) @[ IoU=0.50:0.95 | area= all | maxDets=100 ] = 0.309\n",
+ " Average Precision (AP) @[ IoU=0.50 | area= all | maxDets=100 ] = 0.449\n",
+ " Average Precision (AP) @[ IoU=0.75 | area= all | maxDets=100 ] = 0.338\n",
+ " Average Precision (AP) @[ IoU=0.50:0.95 | area= small | maxDets=100 ] = 0.044\n",
+ " Average Precision (AP) @[ IoU=0.50:0.95 | area=medium | maxDets=100 ] = 0.155\n",
+ " Average Precision (AP) @[ IoU=0.50:0.95 | area= large | maxDets=100 ] = 0.363\n",
+ " Average Recall (AR) @[ IoU=0.50:0.95 | area= all | maxDets= 1 ] = 0.322\n",
+ " Average Recall (AR) @[ IoU=0.50:0.95 | area= all | maxDets= 10 ] = 0.417\n",
+ " Average Recall (AR) @[ IoU=0.50:0.95 | area= all | maxDets=100 ] = 0.420\n",
+ " Average Recall (AR) @[ IoU=0.50:0.95 | area= small | maxDets=100 ] = 0.073\n",
+ " Average Recall (AR) @[ IoU=0.50:0.95 | area=medium | maxDets=100 ] = 0.248\n",
+ " Average Recall (AR) @[ IoU=0.50:0.95 | area= large | maxDets=100 ] = 0.476\n",
+ "4138/4138 [==============================] - 604s 146ms/step - loss: 0.7078 - box_loss: 0.6531 - class_loss: 0.0547 - val_loss: 1.2627 - val_box_loss: 1.1891 - val_class_loss: 0.0736 - val_AP: 0.3092 - val_AP50: 0.4493 - val_AP75: 0.3379 - val_APs: 0.0441 - val_APm: 0.1548 - val_APl: 0.3634 - val_ARmax1: 0.3224 - val_ARmax10: 0.4174 - val_ARmax100: 0.4203 - val_ARs: 0.0735 - val_ARm: 0.2476 - val_ARl: 0.4759\n",
+ "Epoch 56/120\n",
+ "1238/1238 [==============================] - 111s 89ms/step\n",
+ "creating index...\n",
+ "index created!\n",
+ "creating index...\n",
+ "index created!\n",
+ "Running per image evaluation...\n",
+ "Evaluate annotation type *bbox*\n",
+ "DONE (t=5.35s).\n",
+ "Accumulating evaluation results...\n",
+ "DONE (t=1.02s).\n",
+ " Average Precision (AP) @[ IoU=0.50:0.95 | area= all | maxDets=100 ] = 0.310\n",
+ " Average Precision (AP) @[ IoU=0.50 | area= all | maxDets=100 ] = 0.448\n",
+ " Average Precision (AP) @[ IoU=0.75 | area= all | maxDets=100 ] = 0.338\n",
+ " Average Precision (AP) @[ IoU=0.50:0.95 | area= small | maxDets=100 ] = 0.035\n",
+ " Average Precision (AP) @[ IoU=0.50:0.95 | area=medium | maxDets=100 ] = 0.152\n",
+ " Average Precision (AP) @[ IoU=0.50:0.95 | area= large | maxDets=100 ] = 0.364\n",
+ " Average Recall (AR) @[ IoU=0.50:0.95 | area= all | maxDets= 1 ] = 0.320\n",
+ " Average Recall (AR) @[ IoU=0.50:0.95 | area= all | maxDets= 10 ] = 0.416\n",
+ " Average Recall (AR) @[ IoU=0.50:0.95 | area= all | maxDets=100 ] = 0.419\n",
+ " Average Recall (AR) @[ IoU=0.50:0.95 | area= small | maxDets=100 ] = 0.068\n",
+ " Average Recall (AR) @[ IoU=0.50:0.95 | area=medium | maxDets=100 ] = 0.247\n",
+ " Average Recall (AR) @[ IoU=0.50:0.95 | area= large | maxDets=100 ] = 0.474\n",
+ "4138/4138 [==============================] - 606s 146ms/step - loss: 0.6986 - box_loss: 0.6445 - class_loss: 0.0541 - val_loss: 1.2498 - val_box_loss: 1.1760 - val_class_loss: 0.0738 - val_AP: 0.3095 - val_AP50: 0.4480 - val_AP75: 0.3380 - val_APs: 0.0349 - val_APm: 0.1524 - val_APl: 0.3641 - val_ARmax1: 0.3203 - val_ARmax10: 0.4162 - val_ARmax100: 0.4189 - val_ARs: 0.0678 - val_ARm: 0.2468 - val_ARl: 0.4745\n",
+ "Epoch 57/120\n",
+ "1238/1238 [==============================] - 111s 89ms/step\n",
+ "creating index...\n",
+ "index created!\n",
+ "creating index...\n",
+ "index created!\n",
+ "Running per image evaluation...\n",
+ "Evaluate annotation type *bbox*\n",
+ "DONE (t=4.72s).\n",
+ "Accumulating evaluation results...\n",
+ "DONE (t=1.03s).\n",
+ " Average Precision (AP) @[ IoU=0.50:0.95 | area= all | maxDets=100 ] = 0.312\n",
+ " Average Precision (AP) @[ IoU=0.50 | area= all | maxDets=100 ] = 0.451\n",
+ " Average Precision (AP) @[ IoU=0.75 | area= all | maxDets=100 ] = 0.340\n",
+ " Average Precision (AP) @[ IoU=0.50:0.95 | area= small | maxDets=100 ] = 0.033\n",
+ " Average Precision (AP) @[ IoU=0.50:0.95 | area=medium | maxDets=100 ] = 0.154\n",
+ " Average Precision (AP) @[ IoU=0.50:0.95 | area= large | maxDets=100 ] = 0.365\n",
+ " Average Recall (AR) @[ IoU=0.50:0.95 | area= all | maxDets= 1 ] = 0.320\n",
+ " Average Recall (AR) @[ IoU=0.50:0.95 | area= all | maxDets= 10 ] = 0.416\n",
+ " Average Recall (AR) @[ IoU=0.50:0.95 | area= all | maxDets=100 ] = 0.419\n",
+ " Average Recall (AR) @[ IoU=0.50:0.95 | area= small | maxDets=100 ] = 0.061\n",
+ " Average Recall (AR) @[ IoU=0.50:0.95 | area=medium | maxDets=100 ] = 0.247\n",
+ " Average Recall (AR) @[ IoU=0.50:0.95 | area= large | maxDets=100 ] = 0.471\n",
+ "4138/4138 [==============================] - 603s 146ms/step - loss: 0.7083 - box_loss: 0.6536 - class_loss: 0.0547 - val_loss: 1.2516 - val_box_loss: 1.1791 - val_class_loss: 0.0725 - val_AP: 0.3122 - val_AP50: 0.4505 - val_AP75: 0.3399 - val_APs: 0.0328 - val_APm: 0.1539 - val_APl: 0.3653 - val_ARmax1: 0.3201 - val_ARmax10: 0.4163 - val_ARmax100: 0.4185 - val_ARs: 0.0610 - val_ARm: 0.2466 - val_ARl: 0.4709\n",
+ "Epoch 58/120\n",
+ "1238/1238 [==============================] - 111s 89ms/step\n",
+ "creating index...\n",
+ "index created!\n",
+ "creating index...\n",
+ "index created!\n",
+ "Running per image evaluation...\n",
+ "Evaluate annotation type *bbox*\n",
+ "DONE (t=5.34s).\n",
+ "Accumulating evaluation results...\n",
+ "DONE (t=1.02s).\n",
+ " Average Precision (AP) @[ IoU=0.50:0.95 | area= all | maxDets=100 ] = 0.316\n",
+ " Average Precision (AP) @[ IoU=0.50 | area= all | maxDets=100 ] = 0.457\n",
+ " Average Precision (AP) @[ IoU=0.75 | area= all | maxDets=100 ] = 0.346\n",
+ " Average Precision (AP) @[ IoU=0.50:0.95 | area= small | maxDets=100 ] = 0.039\n",
+ " Average Precision (AP) @[ IoU=0.50:0.95 | area=medium | maxDets=100 ] = 0.157\n",
+ " Average Precision (AP) @[ IoU=0.50:0.95 | area= large | maxDets=100 ] = 0.370\n",
+ " Average Recall (AR) @[ IoU=0.50:0.95 | area= all | maxDets= 1 ] = 0.325\n",
+ " Average Recall (AR) @[ IoU=0.50:0.95 | area= all | maxDets= 10 ] = 0.423\n",
+ " Average Recall (AR) @[ IoU=0.50:0.95 | area= all | maxDets=100 ] = 0.426\n",
+ " Average Recall (AR) @[ IoU=0.50:0.95 | area= small | maxDets=100 ] = 0.063\n",
+ " Average Recall (AR) @[ IoU=0.50:0.95 | area=medium | maxDets=100 ] = 0.246\n",
+ " Average Recall (AR) @[ IoU=0.50:0.95 | area= large | maxDets=100 ] = 0.482\n",
+ "4138/4138 [==============================] - 605s 146ms/step - loss: 0.6981 - box_loss: 0.6443 - class_loss: 0.0538 - val_loss: 1.2455 - val_box_loss: 1.1739 - val_class_loss: 0.0716 - val_AP: 0.3160 - val_AP50: 0.4565 - val_AP75: 0.3463 - val_APs: 0.0389 - val_APm: 0.1572 - val_APl: 0.3704 - val_ARmax1: 0.3253 - val_ARmax10: 0.4228 - val_ARmax100: 0.4256 - val_ARs: 0.0632 - val_ARm: 0.2462 - val_ARl: 0.4817\n",
+ "Epoch 59/120\n",
+ "1238/1238 [==============================] - 110s 89ms/step\n",
+ "creating index...\n",
+ "index created!\n",
+ "creating index...\n",
+ "index created!\n",
+ "Running per image evaluation...\n",
+ "Evaluate annotation type *bbox*\n",
+ "DONE (t=4.84s).\n",
+ "Accumulating evaluation results...\n",
+ "DONE (t=1.03s).\n",
+ " Average Precision (AP) @[ IoU=0.50:0.95 | area= all | maxDets=100 ] = 0.315\n",
+ " Average Precision (AP) @[ IoU=0.50 | area= all | maxDets=100 ] = 0.455\n",
+ " Average Precision (AP) @[ IoU=0.75 | area= all | maxDets=100 ] = 0.347\n",
+ " Average Precision (AP) @[ IoU=0.50:0.95 | area= small | maxDets=100 ] = 0.035\n",
+ " Average Precision (AP) @[ IoU=0.50:0.95 | area=medium | maxDets=100 ] = 0.156\n",
+ " Average Precision (AP) @[ IoU=0.50:0.95 | area= large | maxDets=100 ] = 0.370\n",
+ " Average Recall (AR) @[ IoU=0.50:0.95 | area= all | maxDets= 1 ] = 0.324\n",
+ " Average Recall (AR) @[ IoU=0.50:0.95 | area= all | maxDets= 10 ] = 0.426\n",
+ " Average Recall (AR) @[ IoU=0.50:0.95 | area= all | maxDets=100 ] = 0.429\n",
+ " Average Recall (AR) @[ IoU=0.50:0.95 | area= small | maxDets=100 ] = 0.063\n",
+ " Average Recall (AR) @[ IoU=0.50:0.95 | area=medium | maxDets=100 ] = 0.250\n",
+ " Average Recall (AR) @[ IoU=0.50:0.95 | area= large | maxDets=100 ] = 0.484\n",
+ "4138/4138 [==============================] - 604s 146ms/step - loss: 0.6801 - box_loss: 0.6278 - class_loss: 0.0524 - val_loss: 1.2500 - val_box_loss: 1.1775 - val_class_loss: 0.0725 - val_AP: 0.3152 - val_AP50: 0.4553 - val_AP75: 0.3473 - val_APs: 0.0350 - val_APm: 0.1556 - val_APl: 0.3702 - val_ARmax1: 0.3240 - val_ARmax10: 0.4261 - val_ARmax100: 0.4286 - val_ARs: 0.0635 - val_ARm: 0.2496 - val_ARl: 0.4840\n",
+ "Epoch 60/120\n",
+ "1238/1238 [==============================] - 111s 89ms/step\n",
+ "creating index...\n",
+ "index created!\n",
+ "creating index...\n",
+ "index created!\n",
+ "Running per image evaluation...\n",
+ "Evaluate annotation type *bbox*\n",
+ "DONE (t=5.38s).\n",
+ "Accumulating evaluation results...\n",
+ "DONE (t=1.03s).\n",
+ " Average Precision (AP) @[ IoU=0.50:0.95 | area= all | maxDets=100 ] = 0.319\n",
+ " Average Precision (AP) @[ IoU=0.50 | area= all | maxDets=100 ] = 0.459\n",
+ " Average Precision (AP) @[ IoU=0.75 | area= all | maxDets=100 ] = 0.351\n",
+ " Average Precision (AP) @[ IoU=0.50:0.95 | area= small | maxDets=100 ] = 0.030\n",
+ " Average Precision (AP) @[ IoU=0.50:0.95 | area=medium | maxDets=100 ] = 0.163\n",
+ " Average Precision (AP) @[ IoU=0.50:0.95 | area= large | maxDets=100 ] = 0.373\n",
+ " Average Recall (AR) @[ IoU=0.50:0.95 | area= all | maxDets= 1 ] = 0.328\n",
+ " Average Recall (AR) @[ IoU=0.50:0.95 | area= all | maxDets= 10 ] = 0.427\n",
+ " Average Recall (AR) @[ IoU=0.50:0.95 | area= all | maxDets=100 ] = 0.430\n",
+ " Average Recall (AR) @[ IoU=0.50:0.95 | area= small | maxDets=100 ] = 0.059\n",
+ " Average Recall (AR) @[ IoU=0.50:0.95 | area=medium | maxDets=100 ] = 0.258\n",
+ " Average Recall (AR) @[ IoU=0.50:0.95 | area= large | maxDets=100 ] = 0.483\n",
+ "4138/4138 [==============================] - 606s 146ms/step - loss: 0.6702 - box_loss: 0.6184 - class_loss: 0.0519 - val_loss: 1.2401 - val_box_loss: 1.1686 - val_class_loss: 0.0715 - val_AP: 0.3192 - val_AP50: 0.4590 - val_AP75: 0.3506 - val_APs: 0.0301 - val_APm: 0.1628 - val_APl: 0.3732 - val_ARmax1: 0.3282 - val_ARmax10: 0.4272 - val_ARmax100: 0.4300 - val_ARs: 0.0593 - val_ARm: 0.2576 - val_ARl: 0.4825\n",
+ "Epoch 61/120\n",
+ "1238/1238 [==============================] - 111s 89ms/step\n",
+ "creating index...\n",
+ "index created!\n",
+ "creating index...\n",
+ "index created!\n",
+ "Running per image evaluation...\n",
+ "Evaluate annotation type *bbox*\n",
+ "DONE (t=4.79s).\n",
+ "Accumulating evaluation results...\n",
+ "DONE (t=1.02s).\n",
+ " Average Precision (AP) @[ IoU=0.50:0.95 | area= all | maxDets=100 ] = 0.317\n",
+ " Average Precision (AP) @[ IoU=0.50 | area= all | maxDets=100 ] = 0.460\n",
+ " Average Precision (AP) @[ IoU=0.75 | area= all | maxDets=100 ] = 0.345\n",
+ " Average Precision (AP) @[ IoU=0.50:0.95 | area= small | maxDets=100 ] = 0.039\n",
+ " Average Precision (AP) @[ IoU=0.50:0.95 | area=medium | maxDets=100 ] = 0.156\n",
+ " Average Precision (AP) @[ IoU=0.50:0.95 | area= large | maxDets=100 ] = 0.370\n",
+ " Average Recall (AR) @[ IoU=0.50:0.95 | area= all | maxDets= 1 ] = 0.324\n",
+ " Average Recall (AR) @[ IoU=0.50:0.95 | area= all | maxDets= 10 ] = 0.426\n",
+ " Average Recall (AR) @[ IoU=0.50:0.95 | area= all | maxDets=100 ] = 0.429\n",
+ " Average Recall (AR) @[ IoU=0.50:0.95 | area= small | maxDets=100 ] = 0.068\n",
+ " Average Recall (AR) @[ IoU=0.50:0.95 | area=medium | maxDets=100 ] = 0.253\n",
+ " Average Recall (AR) @[ IoU=0.50:0.95 | area= large | maxDets=100 ] = 0.483\n",
+ "4138/4138 [==============================] - 606s 146ms/step - loss: 0.6786 - box_loss: 0.6265 - class_loss: 0.0522 - val_loss: 1.2553 - val_box_loss: 1.1827 - val_class_loss: 0.0726 - val_AP: 0.3167 - val_AP50: 0.4597 - val_AP75: 0.3448 - val_APs: 0.0385 - val_APm: 0.1564 - val_APl: 0.3703 - val_ARmax1: 0.3241 - val_ARmax10: 0.4261 - val_ARmax100: 0.4291 - val_ARs: 0.0676 - val_ARm: 0.2527 - val_ARl: 0.4825\n",
+ "Epoch 62/120\n",
+ "1238/1238 [==============================] - 111s 89ms/step\n",
+ "creating index...\n",
+ "index created!\n",
+ "creating index...\n",
+ "index created!\n",
+ "Running per image evaluation...\n",
+ "Evaluate annotation type *bbox*\n",
+ "DONE (t=4.80s).\n",
+ "Accumulating evaluation results...\n",
+ "DONE (t=1.03s).\n",
+ " Average Precision (AP) @[ IoU=0.50:0.95 | area= all | maxDets=100 ] = 0.323\n",
+ " Average Precision (AP) @[ IoU=0.50 | area= all | maxDets=100 ] = 0.466\n",
+ " Average Precision (AP) @[ IoU=0.75 | area= all | maxDets=100 ] = 0.357\n",
+ " Average Precision (AP) @[ IoU=0.50:0.95 | area= small | maxDets=100 ] = 0.041\n",
+ " Average Precision (AP) @[ IoU=0.50:0.95 | area=medium | maxDets=100 ] = 0.157\n",
+ " Average Precision (AP) @[ IoU=0.50:0.95 | area= large | maxDets=100 ] = 0.381\n",
+ " Average Recall (AR) @[ IoU=0.50:0.95 | area= all | maxDets= 1 ] = 0.327\n",
+ " Average Recall (AR) @[ IoU=0.50:0.95 | area= all | maxDets= 10 ] = 0.429\n",
+ " Average Recall (AR) @[ IoU=0.50:0.95 | area= all | maxDets=100 ] = 0.432\n",
+ " Average Recall (AR) @[ IoU=0.50:0.95 | area= small | maxDets=100 ] = 0.074\n",
+ " Average Recall (AR) @[ IoU=0.50:0.95 | area=medium | maxDets=100 ] = 0.250\n",
+ " Average Recall (AR) @[ IoU=0.50:0.95 | area= large | maxDets=100 ] = 0.491\n",
+ "4138/4138 [==============================] - 605s 146ms/step - loss: 0.6773 - box_loss: 0.6249 - class_loss: 0.0524 - val_loss: 1.2505 - val_box_loss: 1.1786 - val_class_loss: 0.0719 - val_AP: 0.3231 - val_AP50: 0.4665 - val_AP75: 0.3571 - val_APs: 0.0413 - val_APm: 0.1574 - val_APl: 0.3812 - val_ARmax1: 0.3270 - val_ARmax10: 0.4287 - val_ARmax100: 0.4320 - val_ARs: 0.0744 - val_ARm: 0.2502 - val_ARl: 0.4909\n",
+ "Epoch 63/120\n",
+ "1238/1238 [==============================] - 111s 89ms/step\n",
+ "creating index...\n",
+ "index created!\n",
+ "creating index...\n",
+ "index created!\n",
+ "Running per image evaluation...\n",
+ "Evaluate annotation type *bbox*\n",
+ "DONE (t=4.72s).\n",
+ "Accumulating evaluation results...\n",
+ "DONE (t=1.03s).\n",
+ " Average Precision (AP) @[ IoU=0.50:0.95 | area= all | maxDets=100 ] = 0.321\n",
+ " Average Precision (AP) @[ IoU=0.50 | area= all | maxDets=100 ] = 0.468\n",
+ " Average Precision (AP) @[ IoU=0.75 | area= all | maxDets=100 ] = 0.352\n",
+ " Average Precision (AP) @[ IoU=0.50:0.95 | area= small | maxDets=100 ] = 0.038\n",
+ " Average Precision (AP) @[ IoU=0.50:0.95 | area=medium | maxDets=100 ] = 0.165\n",
+ " Average Precision (AP) @[ IoU=0.50:0.95 | area= large | maxDets=100 ] = 0.375\n",
+ " Average Recall (AR) @[ IoU=0.50:0.95 | area= all | maxDets= 1 ] = 0.327\n",
+ " Average Recall (AR) @[ IoU=0.50:0.95 | area= all | maxDets= 10 ] = 0.428\n",
+ " Average Recall (AR) @[ IoU=0.50:0.95 | area= all | maxDets=100 ] = 0.431\n",
+ " Average Recall (AR) @[ IoU=0.50:0.95 | area= small | maxDets=100 ] = 0.070\n",
+ " Average Recall (AR) @[ IoU=0.50:0.95 | area=medium | maxDets=100 ] = 0.255\n",
+ " Average Recall (AR) @[ IoU=0.50:0.95 | area= large | maxDets=100 ] = 0.485\n",
+ "4138/4138 [==============================] - 604s 146ms/step - loss: 0.6592 - box_loss: 0.6082 - class_loss: 0.0510 - val_loss: 1.2489 - val_box_loss: 1.1781 - val_class_loss: 0.0707 - val_AP: 0.3214 - val_AP50: 0.4682 - val_AP75: 0.3520 - val_APs: 0.0380 - val_APm: 0.1652 - val_APl: 0.3750 - val_ARmax1: 0.3267 - val_ARmax10: 0.4277 - val_ARmax100: 0.4306 - val_ARs: 0.0696 - val_ARm: 0.2551 - val_ARl: 0.4850\n",
+ "Epoch 64/120\n",
+ "1238/1238 [==============================] - 111s 89ms/step\n",
+ "creating index...\n",
+ "index created!\n",
+ "creating index...\n",
+ "index created!\n",
+ "Running per image evaluation...\n",
+ "Evaluate annotation type *bbox*\n",
+ "DONE (t=4.67s).\n",
+ "Accumulating evaluation results...\n",
+ "DONE (t=1.00s).\n",
+ " Average Precision (AP) @[ IoU=0.50:0.95 | area= all | maxDets=100 ] = 0.325\n",
+ " Average Precision (AP) @[ IoU=0.50 | area= all | maxDets=100 ] = 0.467\n",
+ " Average Precision (AP) @[ IoU=0.75 | area= all | maxDets=100 ] = 0.356\n",
+ " Average Precision (AP) @[ IoU=0.50:0.95 | area= small | maxDets=100 ] = 0.037\n",
+ " Average Precision (AP) @[ IoU=0.50:0.95 | area=medium | maxDets=100 ] = 0.159\n",
+ " Average Precision (AP) @[ IoU=0.50:0.95 | area= large | maxDets=100 ] = 0.381\n",
+ " Average Recall (AR) @[ IoU=0.50:0.95 | area= all | maxDets= 1 ] = 0.330\n",
+ " Average Recall (AR) @[ IoU=0.50:0.95 | area= all | maxDets= 10 ] = 0.432\n",
+ " Average Recall (AR) @[ IoU=0.50:0.95 | area= all | maxDets=100 ] = 0.435\n",
+ " Average Recall (AR) @[ IoU=0.50:0.95 | area= small | maxDets=100 ] = 0.065\n",
+ " Average Recall (AR) @[ IoU=0.50:0.95 | area=medium | maxDets=100 ] = 0.257\n",
+ " Average Recall (AR) @[ IoU=0.50:0.95 | area= large | maxDets=100 ] = 0.494\n",
+ "4138/4138 [==============================] - 605s 146ms/step - loss: 0.6498 - box_loss: 0.5996 - class_loss: 0.0501 - val_loss: 1.2489 - val_box_loss: 1.1776 - val_class_loss: 0.0713 - val_AP: 0.3250 - val_AP50: 0.4672 - val_AP75: 0.3558 - val_APs: 0.0372 - val_APm: 0.1591 - val_APl: 0.3811 - val_ARmax1: 0.3299 - val_ARmax10: 0.4320 - val_ARmax100: 0.4347 - val_ARs: 0.0651 - val_ARm: 0.2573 - val_ARl: 0.4943\n",
+ "Epoch 65/120\n",
+ "1238/1238 [==============================] - 111s 89ms/step\n",
+ "creating index...\n",
+ "index created!\n",
+ "creating index...\n",
+ "index created!\n",
+ "Running per image evaluation...\n",
+ "Evaluate annotation type *bbox*\n",
+ "DONE (t=4.80s).\n",
+ "Accumulating evaluation results...\n",
+ "DONE (t=1.02s).\n",
+ " Average Precision (AP) @[ IoU=0.50:0.95 | area= all | maxDets=100 ] = 0.326\n",
+ " Average Precision (AP) @[ IoU=0.50 | area= all | maxDets=100 ] = 0.471\n",
+ " Average Precision (AP) @[ IoU=0.75 | area= all | maxDets=100 ] = 0.357\n",
+ " Average Precision (AP) @[ IoU=0.50:0.95 | area= small | maxDets=100 ] = 0.041\n",
+ " Average Precision (AP) @[ IoU=0.50:0.95 | area=medium | maxDets=100 ] = 0.158\n",
+ " Average Precision (AP) @[ IoU=0.50:0.95 | area= large | maxDets=100 ] = 0.384\n",
+ " Average Recall (AR) @[ IoU=0.50:0.95 | area= all | maxDets= 1 ] = 0.334\n",
+ " Average Recall (AR) @[ IoU=0.50:0.95 | area= all | maxDets= 10 ] = 0.435\n",
+ " Average Recall (AR) @[ IoU=0.50:0.95 | area= all | maxDets=100 ] = 0.438\n",
+ " Average Recall (AR) @[ IoU=0.50:0.95 | area= small | maxDets=100 ] = 0.072\n",
+ " Average Recall (AR) @[ IoU=0.50:0.95 | area=medium | maxDets=100 ] = 0.253\n",
+ " Average Recall (AR) @[ IoU=0.50:0.95 | area= large | maxDets=100 ] = 0.498\n",
+ "4138/4138 [==============================] - 605s 146ms/step - loss: 0.6423 - box_loss: 0.5929 - class_loss: 0.0494 - val_loss: 1.2458 - val_box_loss: 1.1751 - val_class_loss: 0.0707 - val_AP: 0.3257 - val_AP50: 0.4712 - val_AP75: 0.3573 - val_APs: 0.0412 - val_APm: 0.1581 - val_APl: 0.3844 - val_ARmax1: 0.3342 - val_ARmax10: 0.4353 - val_ARmax100: 0.4381 - val_ARs: 0.0723 - val_ARm: 0.2529 - val_ARl: 0.4976\n",
+ "Epoch 66/120\n",
+ "1238/1238 [==============================] - 111s 89ms/step\n",
+ "creating index...\n",
+ "index created!\n",
+ "creating index...\n",
+ "index created!\n",
+ "Running per image evaluation...\n",
+ "Evaluate annotation type *bbox*\n",
+ "DONE (t=4.78s).\n",
+ "Accumulating evaluation results...\n",
+ "DONE (t=1.02s).\n",
+ " Average Precision (AP) @[ IoU=0.50:0.95 | area= all | maxDets=100 ] = 0.328\n",
+ " Average Precision (AP) @[ IoU=0.50 | area= all | maxDets=100 ] = 0.476\n",
+ " Average Precision (AP) @[ IoU=0.75 | area= all | maxDets=100 ] = 0.358\n",
+ " Average Precision (AP) @[ IoU=0.50:0.95 | area= small | maxDets=100 ] = 0.044\n",
+ " Average Precision (AP) @[ IoU=0.50:0.95 | area=medium | maxDets=100 ] = 0.163\n",
+ " Average Precision (AP) @[ IoU=0.50:0.95 | area= large | maxDets=100 ] = 0.383\n",
+ " Average Recall (AR) @[ IoU=0.50:0.95 | area= all | maxDets= 1 ] = 0.331\n",
+ " Average Recall (AR) @[ IoU=0.50:0.95 | area= all | maxDets= 10 ] = 0.436\n",
+ " Average Recall (AR) @[ IoU=0.50:0.95 | area= all | maxDets=100 ] = 0.439\n",
+ " Average Recall (AR) @[ IoU=0.50:0.95 | area= small | maxDets=100 ] = 0.075\n",
+ " Average Recall (AR) @[ IoU=0.50:0.95 | area=medium | maxDets=100 ] = 0.256\n",
+ " Average Recall (AR) @[ IoU=0.50:0.95 | area= large | maxDets=100 ] = 0.494\n",
+ "4138/4138 [==============================] - 605s 146ms/step - loss: 0.6395 - box_loss: 0.5902 - class_loss: 0.0493 - val_loss: 1.2390 - val_box_loss: 1.1682 - val_class_loss: 0.0707 - val_AP: 0.3281 - val_AP50: 0.4756 - val_AP75: 0.3583 - val_APs: 0.0443 - val_APm: 0.1631 - val_APl: 0.3826 - val_ARmax1: 0.3308 - val_ARmax10: 0.4362 - val_ARmax100: 0.4391 - val_ARs: 0.0748 - val_ARm: 0.2564 - val_ARl: 0.4938\n",
+ "Epoch 67/120\n",
+ "1238/1238 [==============================] - 111s 89ms/step\n",
+ "creating index...\n",
+ "index created!\n",
+ "creating index...\n",
+ "index created!\n",
+ "Running per image evaluation...\n",
+ "Evaluate annotation type *bbox*\n",
+ "DONE (t=4.83s).\n",
+ "Accumulating evaluation results...\n",
+ "DONE (t=1.03s).\n",
+ " Average Precision (AP) @[ IoU=0.50:0.95 | area= all | maxDets=100 ] = 0.327\n",
+ " Average Precision (AP) @[ IoU=0.50 | area= all | maxDets=100 ] = 0.472\n",
+ " Average Precision (AP) @[ IoU=0.75 | area= all | maxDets=100 ] = 0.359\n",
+ " Average Precision (AP) @[ IoU=0.50:0.95 | area= small | maxDets=100 ] = 0.045\n",
+ " Average Precision (AP) @[ IoU=0.50:0.95 | area=medium | maxDets=100 ] = 0.164\n",
+ " Average Precision (AP) @[ IoU=0.50:0.95 | area= large | maxDets=100 ] = 0.384\n",
+ " Average Recall (AR) @[ IoU=0.50:0.95 | area= all | maxDets= 1 ] = 0.332\n",
+ " Average Recall (AR) @[ IoU=0.50:0.95 | area= all | maxDets= 10 ] = 0.436\n",
+ " Average Recall (AR) @[ IoU=0.50:0.95 | area= all | maxDets=100 ] = 0.439\n",
+ " Average Recall (AR) @[ IoU=0.50:0.95 | area= small | maxDets=100 ] = 0.074\n",
+ " Average Recall (AR) @[ IoU=0.50:0.95 | area=medium | maxDets=100 ] = 0.251\n",
+ " Average Recall (AR) @[ IoU=0.50:0.95 | area= large | maxDets=100 ] = 0.498\n",
+ "4138/4138 [==============================] - 605s 146ms/step - loss: 0.6309 - box_loss: 0.5824 - class_loss: 0.0486 - val_loss: 1.2445 - val_box_loss: 1.1740 - val_class_loss: 0.0705 - val_AP: 0.3271 - val_AP50: 0.4718 - val_AP75: 0.3593 - val_APs: 0.0448 - val_APm: 0.1639 - val_APl: 0.3839 - val_ARmax1: 0.3322 - val_ARmax10: 0.4358 - val_ARmax100: 0.4388 - val_ARs: 0.0736 - val_ARm: 0.2507 - val_ARl: 0.4982\n",
+ "Epoch 68/120\n",
+ "1238/1238 [==============================] - 111s 89ms/step\n",
+ "creating index...\n",
+ "index created!\n",
+ "creating index...\n",
+ "index created!\n",
+ "Running per image evaluation...\n",
+ "Evaluate annotation type *bbox*\n",
+ "DONE (t=5.42s).\n",
+ "Accumulating evaluation results...\n",
+ "DONE (t=1.03s).\n",
+ " Average Precision (AP) @[ IoU=0.50:0.95 | area= all | maxDets=100 ] = 0.330\n",
+ " Average Precision (AP) @[ IoU=0.50 | area= all | maxDets=100 ] = 0.476\n",
+ " Average Precision (AP) @[ IoU=0.75 | area= all | maxDets=100 ] = 0.361\n",
+ " Average Precision (AP) @[ IoU=0.50:0.95 | area= small | maxDets=100 ] = 0.041\n",
+ " Average Precision (AP) @[ IoU=0.50:0.95 | area=medium | maxDets=100 ] = 0.161\n",
+ " Average Precision (AP) @[ IoU=0.50:0.95 | area= large | maxDets=100 ] = 0.390\n",
+ " Average Recall (AR) @[ IoU=0.50:0.95 | area= all | maxDets= 1 ] = 0.334\n",
+ " Average Recall (AR) @[ IoU=0.50:0.95 | area= all | maxDets= 10 ] = 0.435\n",
+ " Average Recall (AR) @[ IoU=0.50:0.95 | area= all | maxDets=100 ] = 0.438\n",
+ " Average Recall (AR) @[ IoU=0.50:0.95 | area= small | maxDets=100 ] = 0.073\n",
+ " Average Recall (AR) @[ IoU=0.50:0.95 | area=medium | maxDets=100 ] = 0.250\n",
+ " Average Recall (AR) @[ IoU=0.50:0.95 | area= large | maxDets=100 ] = 0.498\n",
+ "4138/4138 [==============================] - 605s 146ms/step - loss: 0.6405 - box_loss: 0.5911 - class_loss: 0.0494 - val_loss: 1.2396 - val_box_loss: 1.1700 - val_class_loss: 0.0696 - val_AP: 0.3300 - val_AP50: 0.4762 - val_AP75: 0.3611 - val_APs: 0.0407 - val_APm: 0.1612 - val_APl: 0.3897 - val_ARmax1: 0.3342 - val_ARmax10: 0.4348 - val_ARmax100: 0.4377 - val_ARs: 0.0735 - val_ARm: 0.2499 - val_ARl: 0.4982\n",
+ "Epoch 69/120\n",
+ "1238/1238 [==============================] - 111s 89ms/step\n",
+ "creating index...\n",
+ "index created!\n",
+ "creating index...\n",
+ "index created!\n",
+ "Running per image evaluation...\n",
+ "Evaluate annotation type *bbox*\n",
+ "DONE (t=4.87s).\n",
+ "Accumulating evaluation results...\n",
+ "DONE (t=1.03s).\n",
+ " Average Precision (AP) @[ IoU=0.50:0.95 | area= all | maxDets=100 ] = 0.335\n",
+ " Average Precision (AP) @[ IoU=0.50 | area= all | maxDets=100 ] = 0.481\n",
+ " Average Precision (AP) @[ IoU=0.75 | area= all | maxDets=100 ] = 0.361\n",
+ " Average Precision (AP) @[ IoU=0.50:0.95 | area= small | maxDets=100 ] = 0.041\n",
+ " Average Precision (AP) @[ IoU=0.50:0.95 | area=medium | maxDets=100 ] = 0.170\n",
+ " Average Precision (AP) @[ IoU=0.50:0.95 | area= large | maxDets=100 ] = 0.392\n",
+ " Average Recall (AR) @[ IoU=0.50:0.95 | area= all | maxDets= 1 ] = 0.338\n",
+ " Average Recall (AR) @[ IoU=0.50:0.95 | area= all | maxDets= 10 ] = 0.440\n",
+ " Average Recall (AR) @[ IoU=0.50:0.95 | area= all | maxDets=100 ] = 0.443\n",
+ " Average Recall (AR) @[ IoU=0.50:0.95 | area= small | maxDets=100 ] = 0.071\n",
+ " Average Recall (AR) @[ IoU=0.50:0.95 | area=medium | maxDets=100 ] = 0.265\n",
+ " Average Recall (AR) @[ IoU=0.50:0.95 | area= large | maxDets=100 ] = 0.500\n",
+ "4138/4138 [==============================] - 605s 146ms/step - loss: 0.6178 - box_loss: 0.5699 - class_loss: 0.0479 - val_loss: 1.2288 - val_box_loss: 1.1592 - val_class_loss: 0.0696 - val_AP: 0.3348 - val_AP50: 0.4811 - val_AP75: 0.3614 - val_APs: 0.0406 - val_APm: 0.1704 - val_APl: 0.3921 - val_ARmax1: 0.3376 - val_ARmax10: 0.4399 - val_ARmax100: 0.4427 - val_ARs: 0.0708 - val_ARm: 0.2646 - val_ARl: 0.5003\n",
+ "Epoch 70/120\n",
+ "1238/1238 [==============================] - 111s 89ms/step\n",
+ "creating index...\n",
+ "index created!\n",
+ "creating index...\n",
+ "index created!\n",
+ "Running per image evaluation...\n",
+ "Evaluate annotation type *bbox*\n",
+ "DONE (t=5.43s).\n",
+ "Accumulating evaluation results...\n",
+ "DONE (t=1.03s).\n",
+ " Average Precision (AP) @[ IoU=0.50:0.95 | area= all | maxDets=100 ] = 0.327\n",
+ " Average Precision (AP) @[ IoU=0.50 | area= all | maxDets=100 ] = 0.471\n",
+ " Average Precision (AP) @[ IoU=0.75 | area= all | maxDets=100 ] = 0.357\n",
+ " Average Precision (AP) @[ IoU=0.50:0.95 | area= small | maxDets=100 ] = 0.038\n",
+ " Average Precision (AP) @[ IoU=0.50:0.95 | area=medium | maxDets=100 ] = 0.160\n",
+ " Average Precision (AP) @[ IoU=0.50:0.95 | area= large | maxDets=100 ] = 0.383\n",
+ " Average Recall (AR) @[ IoU=0.50:0.95 | area= all | maxDets= 1 ] = 0.335\n",
+ " Average Recall (AR) @[ IoU=0.50:0.95 | area= all | maxDets= 10 ] = 0.435\n",
+ " Average Recall (AR) @[ IoU=0.50:0.95 | area= all | maxDets=100 ] = 0.437\n",
+ " Average Recall (AR) @[ IoU=0.50:0.95 | area= small | maxDets=100 ] = 0.065\n",
+ " Average Recall (AR) @[ IoU=0.50:0.95 | area=medium | maxDets=100 ] = 0.253\n",
+ " Average Recall (AR) @[ IoU=0.50:0.95 | area= large | maxDets=100 ] = 0.493\n",
+ "4138/4138 [==============================] - 605s 146ms/step - loss: 0.6195 - box_loss: 0.5718 - class_loss: 0.0477 - val_loss: 1.2435 - val_box_loss: 1.1730 - val_class_loss: 0.0705 - val_AP: 0.3274 - val_AP50: 0.4711 - val_AP75: 0.3566 - val_APs: 0.0376 - val_APm: 0.1595 - val_APl: 0.3825 - val_ARmax1: 0.3354 - val_ARmax10: 0.4346 - val_ARmax100: 0.4374 - val_ARs: 0.0648 - val_ARm: 0.2532 - val_ARl: 0.4932\n",
+ "Epoch 71/120\n",
+ "1238/1238 [==============================] - 111s 89ms/step\n",
+ "creating index...\n",
+ "index created!\n",
+ "creating index...\n",
+ "index created!\n",
+ "Running per image evaluation...\n",
+ "Evaluate annotation type *bbox*\n",
+ "DONE (t=4.76s).\n",
+ "Accumulating evaluation results...\n",
+ "DONE (t=1.03s).\n",
+ " Average Precision (AP) @[ IoU=0.50:0.95 | area= all | maxDets=100 ] = 0.328\n",
+ " Average Precision (AP) @[ IoU=0.50 | area= all | maxDets=100 ] = 0.473\n",
+ " Average Precision (AP) @[ IoU=0.75 | area= all | maxDets=100 ] = 0.356\n",
+ " Average Precision (AP) @[ IoU=0.50:0.95 | area= small | maxDets=100 ] = 0.035\n",
+ " Average Precision (AP) @[ IoU=0.50:0.95 | area=medium | maxDets=100 ] = 0.159\n",
+ " Average Precision (AP) @[ IoU=0.50:0.95 | area= large | maxDets=100 ] = 0.385\n",
+ " Average Recall (AR) @[ IoU=0.50:0.95 | area= all | maxDets= 1 ] = 0.334\n",
+ " Average Recall (AR) @[ IoU=0.50:0.95 | area= all | maxDets= 10 ] = 0.435\n",
+ " Average Recall (AR) @[ IoU=0.50:0.95 | area= all | maxDets=100 ] = 0.438\n",
+ " Average Recall (AR) @[ IoU=0.50:0.95 | area= small | maxDets=100 ] = 0.064\n",
+ " Average Recall (AR) @[ IoU=0.50:0.95 | area=medium | maxDets=100 ] = 0.252\n",
+ " Average Recall (AR) @[ IoU=0.50:0.95 | area= large | maxDets=100 ] = 0.496\n",
+ "4138/4138 [==============================] - 605s 146ms/step - loss: 0.6085 - box_loss: 0.5614 - class_loss: 0.0471 - val_loss: 1.2360 - val_box_loss: 1.1660 - val_class_loss: 0.0700 - val_AP: 0.3283 - val_AP50: 0.4726 - val_AP75: 0.3564 - val_APs: 0.0346 - val_APm: 0.1587 - val_APl: 0.3852 - val_ARmax1: 0.3345 - val_ARmax10: 0.4351 - val_ARmax100: 0.4377 - val_ARs: 0.0639 - val_ARm: 0.2522 - val_ARl: 0.4962\n",
+ "Epoch 72/120\n",
+ "1238/1238 [==============================] - 111s 89ms/step\n",
+ "creating index...\n",
+ "index created!\n",
+ "creating index...\n",
+ "index created!\n",
+ "Running per image evaluation...\n",
+ "Evaluate annotation type *bbox*\n",
+ "DONE (t=4.71s).\n",
+ "Accumulating evaluation results...\n",
+ "DONE (t=1.03s).\n",
+ " Average Precision (AP) @[ IoU=0.50:0.95 | area= all | maxDets=100 ] = 0.331\n",
+ " Average Precision (AP) @[ IoU=0.50 | area= all | maxDets=100 ] = 0.477\n",
+ " Average Precision (AP) @[ IoU=0.75 | area= all | maxDets=100 ] = 0.364\n",
+ " Average Precision (AP) @[ IoU=0.50:0.95 | area= small | maxDets=100 ] = 0.037\n",
+ " Average Precision (AP) @[ IoU=0.50:0.95 | area=medium | maxDets=100 ] = 0.162\n",
+ " Average Precision (AP) @[ IoU=0.50:0.95 | area= large | maxDets=100 ] = 0.388\n",
+ " Average Recall (AR) @[ IoU=0.50:0.95 | area= all | maxDets= 1 ] = 0.336\n",
+ " Average Recall (AR) @[ IoU=0.50:0.95 | area= all | maxDets= 10 ] = 0.438\n",
+ " Average Recall (AR) @[ IoU=0.50:0.95 | area= all | maxDets=100 ] = 0.441\n",
+ " Average Recall (AR) @[ IoU=0.50:0.95 | area= small | maxDets=100 ] = 0.071\n",
+ " Average Recall (AR) @[ IoU=0.50:0.95 | area=medium | maxDets=100 ] = 0.252\n",
+ " Average Recall (AR) @[ IoU=0.50:0.95 | area= large | maxDets=100 ] = 0.498\n",
+ "4138/4138 [==============================] - 604s 146ms/step - loss: 0.6023 - box_loss: 0.5558 - class_loss: 0.0465 - val_loss: 1.2502 - val_box_loss: 1.1798 - val_class_loss: 0.0704 - val_AP: 0.3315 - val_AP50: 0.4766 - val_AP75: 0.3641 - val_APs: 0.0372 - val_APm: 0.1616 - val_APl: 0.3877 - val_ARmax1: 0.3358 - val_ARmax10: 0.4381 - val_ARmax100: 0.4405 - val_ARs: 0.0706 - val_ARm: 0.2519 - val_ARl: 0.4978\n",
+ "Epoch 73/120\n",
+ "1238/1238 [==============================] - 111s 89ms/step\n",
+ "creating index...\n",
+ "index created!\n",
+ "creating index...\n",
+ "index created!\n",
+ "Running per image evaluation...\n",
+ "Evaluate annotation type *bbox*\n",
+ "DONE (t=4.72s).\n",
+ "Accumulating evaluation results...\n",
+ "DONE (t=1.03s).\n",
+ " Average Precision (AP) @[ IoU=0.50:0.95 | area= all | maxDets=100 ] = 0.331\n",
+ " Average Precision (AP) @[ IoU=0.50 | area= all | maxDets=100 ] = 0.477\n",
+ " Average Precision (AP) @[ IoU=0.75 | area= all | maxDets=100 ] = 0.361\n",
+ " Average Precision (AP) @[ IoU=0.50:0.95 | area= small | maxDets=100 ] = 0.050\n",
+ " Average Precision (AP) @[ IoU=0.50:0.95 | area=medium | maxDets=100 ] = 0.165\n",
+ " Average Precision (AP) @[ IoU=0.50:0.95 | area= large | maxDets=100 ] = 0.387\n",
+ " Average Recall (AR) @[ IoU=0.50:0.95 | area= all | maxDets= 1 ] = 0.334\n",
+ " Average Recall (AR) @[ IoU=0.50:0.95 | area= all | maxDets= 10 ] = 0.437\n",
+ " Average Recall (AR) @[ IoU=0.50:0.95 | area= all | maxDets=100 ] = 0.440\n",
+ " Average Recall (AR) @[ IoU=0.50:0.95 | area= small | maxDets=100 ] = 0.087\n",
+ " Average Recall (AR) @[ IoU=0.50:0.95 | area=medium | maxDets=100 ] = 0.257\n",
+ " Average Recall (AR) @[ IoU=0.50:0.95 | area= large | maxDets=100 ] = 0.496\n",
+ "4138/4138 [==============================] - 604s 146ms/step - loss: 0.5951 - box_loss: 0.5490 - class_loss: 0.0461 - val_loss: 1.2444 - val_box_loss: 1.1750 - val_class_loss: 0.0694 - val_AP: 0.3309 - val_AP50: 0.4767 - val_AP75: 0.3608 - val_APs: 0.0497 - val_APm: 0.1647 - val_APl: 0.3865 - val_ARmax1: 0.3341 - val_ARmax10: 0.4373 - val_ARmax100: 0.4405 - val_ARs: 0.0874 - val_ARm: 0.2572 - val_ARl: 0.4958\n",
+ "Epoch 74/120\n",
+ "1238/1238 [==============================] - 111s 89ms/step\n",
+ "creating index...\n",
+ "index created!\n",
+ "creating index...\n",
+ "index created!\n",
+ "Running per image evaluation...\n",
+ "Evaluate annotation type *bbox*\n",
+ "DONE (t=4.81s).\n",
+ "Accumulating evaluation results...\n",
+ "DONE (t=1.03s).\n",
+ " Average Precision (AP) @[ IoU=0.50:0.95 | area= all | maxDets=100 ] = 0.339\n",
+ " Average Precision (AP) @[ IoU=0.50 | area= all | maxDets=100 ] = 0.483\n",
+ " Average Precision (AP) @[ IoU=0.75 | area= all | maxDets=100 ] = 0.371\n",
+ " Average Precision (AP) @[ IoU=0.50:0.95 | area= small | maxDets=100 ] = 0.036\n",
+ " Average Precision (AP) @[ IoU=0.50:0.95 | area=medium | maxDets=100 ] = 0.169\n",
+ " Average Precision (AP) @[ IoU=0.50:0.95 | area= large | maxDets=100 ] = 0.395\n",
+ " Average Recall (AR) @[ IoU=0.50:0.95 | area= all | maxDets= 1 ] = 0.340\n",
+ " Average Recall (AR) @[ IoU=0.50:0.95 | area= all | maxDets= 10 ] = 0.445\n",
+ " Average Recall (AR) @[ IoU=0.50:0.95 | area= all | maxDets=100 ] = 0.448\n",
+ " Average Recall (AR) @[ IoU=0.50:0.95 | area= small | maxDets=100 ] = 0.066\n",
+ " Average Recall (AR) @[ IoU=0.50:0.95 | area=medium | maxDets=100 ] = 0.265\n",
+ " Average Recall (AR) @[ IoU=0.50:0.95 | area= large | maxDets=100 ] = 0.507\n",
+ "4138/4138 [==============================] - 604s 146ms/step - loss: 0.6105 - box_loss: 0.5636 - class_loss: 0.0469 - val_loss: 1.2329 - val_box_loss: 1.1640 - val_class_loss: 0.0689 - val_AP: 0.3386 - val_AP50: 0.4833 - val_AP75: 0.3710 - val_APs: 0.0360 - val_APm: 0.1695 - val_APl: 0.3954 - val_ARmax1: 0.3396 - val_ARmax10: 0.4455 - val_ARmax100: 0.4483 - val_ARs: 0.0662 - val_ARm: 0.2650 - val_ARl: 0.5065\n",
+ "Epoch 75/120\n",
+ "1238/1238 [==============================] - 111s 89ms/step\n",
+ "creating index...\n",
+ "index created!\n",
+ "creating index...\n",
+ "index created!\n",
+ "Running per image evaluation...\n",
+ "Evaluate annotation type *bbox*\n",
+ "DONE (t=5.33s).\n",
+ "Accumulating evaluation results...\n",
+ "DONE (t=1.02s).\n",
+ " Average Precision (AP) @[ IoU=0.50:0.95 | area= all | maxDets=100 ] = 0.335\n",
+ " Average Precision (AP) @[ IoU=0.50 | area= all | maxDets=100 ] = 0.486\n",
+ " Average Precision (AP) @[ IoU=0.75 | area= all | maxDets=100 ] = 0.368\n",
+ " Average Precision (AP) @[ IoU=0.50:0.95 | area= small | maxDets=100 ] = 0.042\n",
+ " Average Precision (AP) @[ IoU=0.50:0.95 | area=medium | maxDets=100 ] = 0.167\n",
+ " Average Precision (AP) @[ IoU=0.50:0.95 | area= large | maxDets=100 ] = 0.393\n",
+ " Average Recall (AR) @[ IoU=0.50:0.95 | area= all | maxDets= 1 ] = 0.336\n",
+ " Average Recall (AR) @[ IoU=0.50:0.95 | area= all | maxDets= 10 ] = 0.440\n",
+ " Average Recall (AR) @[ IoU=0.50:0.95 | area= all | maxDets=100 ] = 0.443\n",
+ " Average Recall (AR) @[ IoU=0.50:0.95 | area= small | maxDets=100 ] = 0.065\n",
+ " Average Recall (AR) @[ IoU=0.50:0.95 | area=medium | maxDets=100 ] = 0.263\n",
+ " Average Recall (AR) @[ IoU=0.50:0.95 | area= large | maxDets=100 ] = 0.502\n",
+ "4138/4138 [==============================] - 605s 146ms/step - loss: 0.5984 - box_loss: 0.5520 - class_loss: 0.0464 - val_loss: 1.2381 - val_box_loss: 1.1687 - val_class_loss: 0.0694 - val_AP: 0.3351 - val_AP50: 0.4856 - val_AP75: 0.3678 - val_APs: 0.0419 - val_APm: 0.1666 - val_APl: 0.3928 - val_ARmax1: 0.3357 - val_ARmax10: 0.4403 - val_ARmax100: 0.4431 - val_ARs: 0.0650 - val_ARm: 0.2626 - val_ARl: 0.5025\n",
+ "Epoch 76/120\n",
+ "1238/1238 [==============================] - 111s 89ms/step\n",
+ "creating index...\n",
+ "index created!\n",
+ "creating index...\n",
+ "index created!\n",
+ "Running per image evaluation...\n",
+ "Evaluate annotation type *bbox*\n",
+ "DONE (t=4.81s).\n",
+ "Accumulating evaluation results...\n",
+ "DONE (t=1.04s).\n",
+ " Average Precision (AP) @[ IoU=0.50:0.95 | area= all | maxDets=100 ] = 0.337\n",
+ " Average Precision (AP) @[ IoU=0.50 | area= all | maxDets=100 ] = 0.485\n",
+ " Average Precision (AP) @[ IoU=0.75 | area= all | maxDets=100 ] = 0.367\n",
+ " Average Precision (AP) @[ IoU=0.50:0.95 | area= small | maxDets=100 ] = 0.040\n",
+ " Average Precision (AP) @[ IoU=0.50:0.95 | area=medium | maxDets=100 ] = 0.165\n",
+ " Average Precision (AP) @[ IoU=0.50:0.95 | area= large | maxDets=100 ] = 0.396\n",
+ " Average Recall (AR) @[ IoU=0.50:0.95 | area= all | maxDets= 1 ] = 0.338\n",
+ " Average Recall (AR) @[ IoU=0.50:0.95 | area= all | maxDets= 10 ] = 0.444\n",
+ " Average Recall (AR) @[ IoU=0.50:0.95 | area= all | maxDets=100 ] = 0.447\n",
+ " Average Recall (AR) @[ IoU=0.50:0.95 | area= small | maxDets=100 ] = 0.073\n",
+ " Average Recall (AR) @[ IoU=0.50:0.95 | area=medium | maxDets=100 ] = 0.259\n",
+ " Average Recall (AR) @[ IoU=0.50:0.95 | area= large | maxDets=100 ] = 0.506\n",
+ "4138/4138 [==============================] - 605s 146ms/step - loss: 0.5940 - box_loss: 0.5480 - class_loss: 0.0460 - val_loss: 1.2382 - val_box_loss: 1.1690 - val_class_loss: 0.0692 - val_AP: 0.3371 - val_AP50: 0.4848 - val_AP75: 0.3673 - val_APs: 0.0397 - val_APm: 0.1652 - val_APl: 0.3963 - val_ARmax1: 0.3376 - val_ARmax10: 0.4440 - val_ARmax100: 0.4471 - val_ARs: 0.0733 - val_ARm: 0.2590 - val_ARl: 0.5062\n",
+ "Epoch 77/120\n",
+ "1238/1238 [==============================] - 111s 89ms/step\n",
+ "creating index...\n",
+ "index created!\n",
+ "creating index...\n",
+ "index created!\n",
+ "Running per image evaluation...\n",
+ "Evaluate annotation type *bbox*\n",
+ "DONE (t=4.80s).\n",
+ "Accumulating evaluation results...\n",
+ "DONE (t=1.02s).\n",
+ " Average Precision (AP) @[ IoU=0.50:0.95 | area= all | maxDets=100 ] = 0.336\n",
+ " Average Precision (AP) @[ IoU=0.50 | area= all | maxDets=100 ] = 0.487\n",
+ " Average Precision (AP) @[ IoU=0.75 | area= all | maxDets=100 ] = 0.366\n",
+ " Average Precision (AP) @[ IoU=0.50:0.95 | area= small | maxDets=100 ] = 0.042\n",
+ " Average Precision (AP) @[ IoU=0.50:0.95 | area=medium | maxDets=100 ] = 0.169\n",
+ " Average Precision (AP) @[ IoU=0.50:0.95 | area= large | maxDets=100 ] = 0.394\n",
+ " Average Recall (AR) @[ IoU=0.50:0.95 | area= all | maxDets= 1 ] = 0.337\n",
+ " Average Recall (AR) @[ IoU=0.50:0.95 | area= all | maxDets= 10 ] = 0.441\n",
+ " Average Recall (AR) @[ IoU=0.50:0.95 | area= all | maxDets=100 ] = 0.444\n",
+ " Average Recall (AR) @[ IoU=0.50:0.95 | area= small | maxDets=100 ] = 0.071\n",
+ " Average Recall (AR) @[ IoU=0.50:0.95 | area=medium | maxDets=100 ] = 0.262\n",
+ " Average Recall (AR) @[ IoU=0.50:0.95 | area= large | maxDets=100 ] = 0.504\n",
+ "4138/4138 [==============================] - 606s 146ms/step - loss: 0.5802 - box_loss: 0.5352 - class_loss: 0.0450 - val_loss: 1.2430 - val_box_loss: 1.1741 - val_class_loss: 0.0689 - val_AP: 0.3358 - val_AP50: 0.4874 - val_AP75: 0.3665 - val_APs: 0.0416 - val_APm: 0.1689 - val_APl: 0.3943 - val_ARmax1: 0.3368 - val_ARmax10: 0.4411 - val_ARmax100: 0.4439 - val_ARs: 0.0706 - val_ARm: 0.2623 - val_ARl: 0.5042\n",
+ "Epoch 78/120\n",
+ "1238/1238 [==============================] - 111s 89ms/step\n",
+ "creating index...\n",
+ "index created!\n",
+ "creating index...\n",
+ "index created!\n",
+ "Running per image evaluation...\n",
+ "Evaluate annotation type *bbox*\n",
+ "DONE (t=4.73s).\n",
+ "Accumulating evaluation results...\n",
+ "DONE (t=1.02s).\n",
+ " Average Precision (AP) @[ IoU=0.50:0.95 | area= all | maxDets=100 ] = 0.341\n",
+ " Average Precision (AP) @[ IoU=0.50 | area= all | maxDets=100 ] = 0.493\n",
+ " Average Precision (AP) @[ IoU=0.75 | area= all | maxDets=100 ] = 0.371\n",
+ " Average Precision (AP) @[ IoU=0.50:0.95 | area= small | maxDets=100 ] = 0.044\n",
+ " Average Precision (AP) @[ IoU=0.50:0.95 | area=medium | maxDets=100 ] = 0.170\n",
+ " Average Precision (AP) @[ IoU=0.50:0.95 | area= large | maxDets=100 ] = 0.400\n",
+ " Average Recall (AR) @[ IoU=0.50:0.95 | area= all | maxDets= 1 ] = 0.344\n",
+ " Average Recall (AR) @[ IoU=0.50:0.95 | area= all | maxDets= 10 ] = 0.449\n",
+ " Average Recall (AR) @[ IoU=0.50:0.95 | area= all | maxDets=100 ] = 0.451\n",
+ " Average Recall (AR) @[ IoU=0.50:0.95 | area= small | maxDets=100 ] = 0.075\n",
+ " Average Recall (AR) @[ IoU=0.50:0.95 | area=medium | maxDets=100 ] = 0.268\n",
+ " Average Recall (AR) @[ IoU=0.50:0.95 | area= large | maxDets=100 ] = 0.509\n",
+ "4138/4138 [==============================] - 605s 146ms/step - loss: 0.5713 - box_loss: 0.5272 - class_loss: 0.0442 - val_loss: 1.2382 - val_box_loss: 1.1692 - val_class_loss: 0.0690 - val_AP: 0.3411 - val_AP50: 0.4931 - val_AP75: 0.3714 - val_APs: 0.0438 - val_APm: 0.1704 - val_APl: 0.3998 - val_ARmax1: 0.3442 - val_ARmax10: 0.4486 - val_ARmax100: 0.4513 - val_ARs: 0.0753 - val_ARm: 0.2684 - val_ARl: 0.5094\n",
+ "Epoch 79/120\n",
+ "1238/1238 [==============================] - 111s 89ms/step\n",
+ "creating index...\n",
+ "index created!\n",
+ "creating index...\n",
+ "index created!\n",
+ "Running per image evaluation...\n",
+ "Evaluate annotation type *bbox*\n",
+ "DONE (t=4.75s).\n",
+ "Accumulating evaluation results...\n",
+ "DONE (t=1.01s).\n",
+ " Average Precision (AP) @[ IoU=0.50:0.95 | area= all | maxDets=100 ] = 0.337\n",
+ " Average Precision (AP) @[ IoU=0.50 | area= all | maxDets=100 ] = 0.485\n",
+ " Average Precision (AP) @[ IoU=0.75 | area= all | maxDets=100 ] = 0.368\n",
+ " Average Precision (AP) @[ IoU=0.50:0.95 | area= small | maxDets=100 ] = 0.042\n",
+ " Average Precision (AP) @[ IoU=0.50:0.95 | area=medium | maxDets=100 ] = 0.178\n",
+ " Average Precision (AP) @[ IoU=0.50:0.95 | area= large | maxDets=100 ] = 0.393\n",
+ " Average Recall (AR) @[ IoU=0.50:0.95 | area= all | maxDets= 1 ] = 0.338\n",
+ " Average Recall (AR) @[ IoU=0.50:0.95 | area= all | maxDets= 10 ] = 0.443\n",
+ " Average Recall (AR) @[ IoU=0.50:0.95 | area= all | maxDets=100 ] = 0.445\n",
+ " Average Recall (AR) @[ IoU=0.50:0.95 | area= small | maxDets=100 ] = 0.073\n",
+ " Average Recall (AR) @[ IoU=0.50:0.95 | area=medium | maxDets=100 ] = 0.269\n",
+ " Average Recall (AR) @[ IoU=0.50:0.95 | area= large | maxDets=100 ] = 0.502\n",
+ "4138/4138 [==============================] - 605s 146ms/step - loss: 0.5786 - box_loss: 0.5338 - class_loss: 0.0448 - val_loss: 1.2447 - val_box_loss: 1.1755 - val_class_loss: 0.0693 - val_AP: 0.3373 - val_AP50: 0.4846 - val_AP75: 0.3685 - val_APs: 0.0417 - val_APm: 0.1775 - val_APl: 0.3933 - val_ARmax1: 0.3377 - val_ARmax10: 0.4426 - val_ARmax100: 0.4451 - val_ARs: 0.0728 - val_ARm: 0.2686 - val_ARl: 0.5016\n",
+ "Epoch 80/120\n",
+ "1238/1238 [==============================] - 111s 89ms/step\n",
+ "creating index...\n",
+ "index created!\n",
+ "creating index...\n",
+ "index created!\n",
+ "Running per image evaluation...\n",
+ "Evaluate annotation type *bbox*\n",
+ "DONE (t=4.82s).\n",
+ "Accumulating evaluation results...\n",
+ "DONE (t=1.03s).\n",
+ " Average Precision (AP) @[ IoU=0.50:0.95 | area= all | maxDets=100 ] = 0.338\n",
+ " Average Precision (AP) @[ IoU=0.50 | area= all | maxDets=100 ] = 0.486\n",
+ " Average Precision (AP) @[ IoU=0.75 | area= all | maxDets=100 ] = 0.371\n",
+ " Average Precision (AP) @[ IoU=0.50:0.95 | area= small | maxDets=100 ] = 0.048\n",
+ " Average Precision (AP) @[ IoU=0.50:0.95 | area=medium | maxDets=100 ] = 0.175\n",
+ " Average Precision (AP) @[ IoU=0.50:0.95 | area= large | maxDets=100 ] = 0.395\n",
+ " Average Recall (AR) @[ IoU=0.50:0.95 | area= all | maxDets= 1 ] = 0.339\n",
+ " Average Recall (AR) @[ IoU=0.50:0.95 | area= all | maxDets= 10 ] = 0.447\n",
+ " Average Recall (AR) @[ IoU=0.50:0.95 | area= all | maxDets=100 ] = 0.450\n",
+ " Average Recall (AR) @[ IoU=0.50:0.95 | area= small | maxDets=100 ] = 0.079\n",
+ " Average Recall (AR) @[ IoU=0.50:0.95 | area=medium | maxDets=100 ] = 0.287\n",
+ " Average Recall (AR) @[ IoU=0.50:0.95 | area= large | maxDets=100 ] = 0.504\n",
+ "4138/4138 [==============================] - 606s 146ms/step - loss: 0.5653 - box_loss: 0.5215 - class_loss: 0.0438 - val_loss: 1.2362 - val_box_loss: 1.1666 - val_class_loss: 0.0696 - val_AP: 0.3384 - val_AP50: 0.4860 - val_AP75: 0.3712 - val_APs: 0.0480 - val_APm: 0.1748 - val_APl: 0.3951 - val_ARmax1: 0.3392 - val_ARmax10: 0.4468 - val_ARmax100: 0.4497 - val_ARs: 0.0787 - val_ARm: 0.2866 - val_ARl: 0.5038\n",
+ "Epoch 81/120\n",
+ "1238/1238 [==============================] - 111s 89ms/step\n",
+ "creating index...\n",
+ "index created!\n",
+ "creating index...\n",
+ "index created!\n",
+ "Running per image evaluation...\n",
+ "Evaluate annotation type *bbox*\n",
+ "DONE (t=4.80s).\n",
+ "Accumulating evaluation results...\n",
+ "DONE (t=1.05s).\n",
+ " Average Precision (AP) @[ IoU=0.50:0.95 | area= all | maxDets=100 ] = 0.339\n",
+ " Average Precision (AP) @[ IoU=0.50 | area= all | maxDets=100 ] = 0.489\n",
+ " Average Precision (AP) @[ IoU=0.75 | area= all | maxDets=100 ] = 0.369\n",
+ " Average Precision (AP) @[ IoU=0.50:0.95 | area= small | maxDets=100 ] = 0.047\n",
+ " Average Precision (AP) @[ IoU=0.50:0.95 | area=medium | maxDets=100 ] = 0.164\n",
+ " Average Precision (AP) @[ IoU=0.50:0.95 | area= large | maxDets=100 ] = 0.397\n",
+ " Average Recall (AR) @[ IoU=0.50:0.95 | area= all | maxDets= 1 ] = 0.341\n",
+ " Average Recall (AR) @[ IoU=0.50:0.95 | area= all | maxDets= 10 ] = 0.446\n",
+ " Average Recall (AR) @[ IoU=0.50:0.95 | area= all | maxDets=100 ] = 0.448\n",
+ " Average Recall (AR) @[ IoU=0.50:0.95 | area= small | maxDets=100 ] = 0.080\n",
+ " Average Recall (AR) @[ IoU=0.50:0.95 | area=medium | maxDets=100 ] = 0.258\n",
+ " Average Recall (AR) @[ IoU=0.50:0.95 | area= large | maxDets=100 ] = 0.509\n",
+ "4138/4138 [==============================] - 606s 146ms/step - loss: 0.5614 - box_loss: 0.5179 - class_loss: 0.0435 - val_loss: 1.2338 - val_box_loss: 1.1650 - val_class_loss: 0.0687 - val_AP: 0.3386 - val_AP50: 0.4893 - val_AP75: 0.3693 - val_APs: 0.0466 - val_APm: 0.1645 - val_APl: 0.3970 - val_ARmax1: 0.3408 - val_ARmax10: 0.4457 - val_ARmax100: 0.4484 - val_ARs: 0.0804 - val_ARm: 0.2577 - val_ARl: 0.5087\n",
+ "Epoch 82/120\n",
+ "1238/1238 [==============================] - 111s 89ms/step\n",
+ "creating index...\n",
+ "index created!\n",
+ "creating index...\n",
+ "index created!\n",
+ "Running per image evaluation...\n",
+ "Evaluate annotation type *bbox*\n",
+ "DONE (t=4.70s).\n",
+ "Accumulating evaluation results...\n",
+ "DONE (t=1.01s).\n",
+ " Average Precision (AP) @[ IoU=0.50:0.95 | area= all | maxDets=100 ] = 0.341\n",
+ " Average Precision (AP) @[ IoU=0.50 | area= all | maxDets=100 ] = 0.494\n",
+ " Average Precision (AP) @[ IoU=0.75 | area= all | maxDets=100 ] = 0.372\n",
+ " Average Precision (AP) @[ IoU=0.50:0.95 | area= small | maxDets=100 ] = 0.054\n",
+ " Average Precision (AP) @[ IoU=0.50:0.95 | area=medium | maxDets=100 ] = 0.174\n",
+ " Average Precision (AP) @[ IoU=0.50:0.95 | area= large | maxDets=100 ] = 0.398\n",
+ " Average Recall (AR) @[ IoU=0.50:0.95 | area= all | maxDets= 1 ] = 0.340\n",
+ " Average Recall (AR) @[ IoU=0.50:0.95 | area= all | maxDets= 10 ] = 0.446\n",
+ " Average Recall (AR) @[ IoU=0.50:0.95 | area= all | maxDets=100 ] = 0.450\n",
+ " Average Recall (AR) @[ IoU=0.50:0.95 | area= small | maxDets=100 ] = 0.093\n",
+ " Average Recall (AR) @[ IoU=0.50:0.95 | area=medium | maxDets=100 ] = 0.268\n",
+ " Average Recall (AR) @[ IoU=0.50:0.95 | area= large | maxDets=100 ] = 0.507\n",
+ "4138/4138 [==============================] - 605s 146ms/step - loss: 0.5578 - box_loss: 0.5147 - class_loss: 0.0431 - val_loss: 1.2430 - val_box_loss: 1.1734 - val_class_loss: 0.0696 - val_AP: 0.3413 - val_AP50: 0.4936 - val_AP75: 0.3722 - val_APs: 0.0544 - val_APm: 0.1739 - val_APl: 0.3983 - val_ARmax1: 0.3399 - val_ARmax10: 0.4464 - val_ARmax100: 0.4496 - val_ARs: 0.0930 - val_ARm: 0.2678 - val_ARl: 0.5071\n",
+ "Epoch 83/120\n",
+ "1238/1238 [==============================] - 111s 89ms/step\n",
+ "creating index...\n",
+ "index created!\n",
+ "creating index...\n",
+ "index created!\n",
+ "Running per image evaluation...\n",
+ "Evaluate annotation type *bbox*\n",
+ "DONE (t=4.73s).\n",
+ "Accumulating evaluation results...\n",
+ "DONE (t=1.01s).\n",
+ " Average Precision (AP) @[ IoU=0.50:0.95 | area= all | maxDets=100 ] = 0.340\n",
+ " Average Precision (AP) @[ IoU=0.50 | area= all | maxDets=100 ] = 0.489\n",
+ " Average Precision (AP) @[ IoU=0.75 | area= all | maxDets=100 ] = 0.372\n",
+ " Average Precision (AP) @[ IoU=0.50:0.95 | area= small | maxDets=100 ] = 0.054\n",
+ " Average Precision (AP) @[ IoU=0.50:0.95 | area=medium | maxDets=100 ] = 0.171\n",
+ " Average Precision (AP) @[ IoU=0.50:0.95 | area= large | maxDets=100 ] = 0.398\n",
+ " Average Recall (AR) @[ IoU=0.50:0.95 | area= all | maxDets= 1 ] = 0.340\n",
+ " Average Recall (AR) @[ IoU=0.50:0.95 | area= all | maxDets= 10 ] = 0.446\n",
+ " Average Recall (AR) @[ IoU=0.50:0.95 | area= all | maxDets=100 ] = 0.449\n",
+ " Average Recall (AR) @[ IoU=0.50:0.95 | area= small | maxDets=100 ] = 0.095\n",
+ " Average Recall (AR) @[ IoU=0.50:0.95 | area=medium | maxDets=100 ] = 0.261\n",
+ " Average Recall (AR) @[ IoU=0.50:0.95 | area= large | maxDets=100 ] = 0.508\n",
+ "4138/4138 [==============================] - 605s 146ms/step - loss: 0.5646 - box_loss: 0.5210 - class_loss: 0.0436 - val_loss: 1.2417 - val_box_loss: 1.1733 - val_class_loss: 0.0684 - val_AP: 0.3400 - val_AP50: 0.4892 - val_AP75: 0.3720 - val_APs: 0.0538 - val_APm: 0.1714 - val_APl: 0.3975 - val_ARmax1: 0.3404 - val_ARmax10: 0.4462 - val_ARmax100: 0.4491 - val_ARs: 0.0950 - val_ARm: 0.2612 - val_ARl: 0.5078\n",
+ "Epoch 84/120\n",
+ "1238/1238 [==============================] - 111s 89ms/step\n",
+ "creating index...\n",
+ "index created!\n",
+ "creating index...\n",
+ "index created!\n",
+ "Running per image evaluation...\n",
+ "Evaluate annotation type *bbox*\n",
+ "DONE (t=4.69s).\n",
+ "Accumulating evaluation results...\n",
+ "DONE (t=1.00s).\n",
+ " Average Precision (AP) @[ IoU=0.50:0.95 | area= all | maxDets=100 ] = 0.341\n",
+ " Average Precision (AP) @[ IoU=0.50 | area= all | maxDets=100 ] = 0.489\n",
+ " Average Precision (AP) @[ IoU=0.75 | area= all | maxDets=100 ] = 0.371\n",
+ " Average Precision (AP) @[ IoU=0.50:0.95 | area= small | maxDets=100 ] = 0.050\n",
+ " Average Precision (AP) @[ IoU=0.50:0.95 | area=medium | maxDets=100 ] = 0.169\n",
+ " Average Precision (AP) @[ IoU=0.50:0.95 | area= large | maxDets=100 ] = 0.398\n",
+ " Average Recall (AR) @[ IoU=0.50:0.95 | area= all | maxDets= 1 ] = 0.342\n",
+ " Average Recall (AR) @[ IoU=0.50:0.95 | area= all | maxDets= 10 ] = 0.446\n",
+ " Average Recall (AR) @[ IoU=0.50:0.95 | area= all | maxDets=100 ] = 0.449\n",
+ " Average Recall (AR) @[ IoU=0.50:0.95 | area= small | maxDets=100 ] = 0.086\n",
+ " Average Recall (AR) @[ IoU=0.50:0.95 | area=medium | maxDets=100 ] = 0.265\n",
+ " Average Recall (AR) @[ IoU=0.50:0.95 | area= large | maxDets=100 ] = 0.506\n",
+ "4138/4138 [==============================] - 604s 146ms/step - loss: 0.5480 - box_loss: 0.5056 - class_loss: 0.0425 - val_loss: 1.2412 - val_box_loss: 1.1724 - val_class_loss: 0.0689 - val_AP: 0.3410 - val_AP50: 0.4889 - val_AP75: 0.3713 - val_APs: 0.0497 - val_APm: 0.1690 - val_APl: 0.3982 - val_ARmax1: 0.3417 - val_ARmax10: 0.4461 - val_ARmax100: 0.4490 - val_ARs: 0.0860 - val_ARm: 0.2648 - val_ARl: 0.5060\n",
+ "Epoch 85/120\n",
+ "1238/1238 [==============================] - 111s 89ms/step\n",
+ "creating index...\n",
+ "index created!\n",
+ "creating index...\n",
+ "index created!\n",
+ "Running per image evaluation...\n",
+ "Evaluate annotation type *bbox*\n",
+ "DONE (t=4.77s).\n",
+ "Accumulating evaluation results...\n",
+ "DONE (t=1.01s).\n",
+ " Average Precision (AP) @[ IoU=0.50:0.95 | area= all | maxDets=100 ] = 0.344\n",
+ " Average Precision (AP) @[ IoU=0.50 | area= all | maxDets=100 ] = 0.494\n",
+ " Average Precision (AP) @[ IoU=0.75 | area= all | maxDets=100 ] = 0.377\n",
+ " Average Precision (AP) @[ IoU=0.50:0.95 | area= small | maxDets=100 ] = 0.050\n",
+ " Average Precision (AP) @[ IoU=0.50:0.95 | area=medium | maxDets=100 ] = 0.171\n",
+ " Average Precision (AP) @[ IoU=0.50:0.95 | area= large | maxDets=100 ] = 0.402\n",
+ " Average Recall (AR) @[ IoU=0.50:0.95 | area= all | maxDets= 1 ] = 0.342\n",
+ " Average Recall (AR) @[ IoU=0.50:0.95 | area= all | maxDets= 10 ] = 0.448\n",
+ " Average Recall (AR) @[ IoU=0.50:0.95 | area= all | maxDets=100 ] = 0.451\n",
+ " Average Recall (AR) @[ IoU=0.50:0.95 | area= small | maxDets=100 ] = 0.085\n",
+ " Average Recall (AR) @[ IoU=0.50:0.95 | area=medium | maxDets=100 ] = 0.264\n",
+ " Average Recall (AR) @[ IoU=0.50:0.95 | area= large | maxDets=100 ] = 0.510\n",
+ "4138/4138 [==============================] - 605s 146ms/step - loss: 0.5501 - box_loss: 0.5075 - class_loss: 0.0427 - val_loss: 1.2498 - val_box_loss: 1.1808 - val_class_loss: 0.0689 - val_AP: 0.3443 - val_AP50: 0.4940 - val_AP75: 0.3771 - val_APs: 0.0503 - val_APm: 0.1712 - val_APl: 0.4024 - val_ARmax1: 0.3423 - val_ARmax10: 0.4479 - val_ARmax100: 0.4508 - val_ARs: 0.0852 - val_ARm: 0.2643 - val_ARl: 0.5100\n",
+ "Epoch 86/120\n",
+ "1238/1238 [==============================] - 111s 89ms/step\n",
+ "creating index...\n",
+ "index created!\n",
+ "creating index...\n",
+ "index created!\n",
+ "Running per image evaluation...\n",
+ "Evaluate annotation type *bbox*\n",
+ "DONE (t=4.82s).\n",
+ "Accumulating evaluation results...\n",
+ "DONE (t=1.02s).\n",
+ " Average Precision (AP) @[ IoU=0.50:0.95 | area= all | maxDets=100 ] = 0.345\n",
+ " Average Precision (AP) @[ IoU=0.50 | area= all | maxDets=100 ] = 0.497\n",
+ " Average Precision (AP) @[ IoU=0.75 | area= all | maxDets=100 ] = 0.375\n",
+ " Average Precision (AP) @[ IoU=0.50:0.95 | area= small | maxDets=100 ] = 0.050\n",
+ " Average Precision (AP) @[ IoU=0.50:0.95 | area=medium | maxDets=100 ] = 0.166\n",
+ " Average Precision (AP) @[ IoU=0.50:0.95 | area= large | maxDets=100 ] = 0.404\n",
+ " Average Recall (AR) @[ IoU=0.50:0.95 | area= all | maxDets= 1 ] = 0.343\n",
+ " Average Recall (AR) @[ IoU=0.50:0.95 | area= all | maxDets= 10 ] = 0.450\n",
+ " Average Recall (AR) @[ IoU=0.50:0.95 | area= all | maxDets=100 ] = 0.453\n",
+ " Average Recall (AR) @[ IoU=0.50:0.95 | area= small | maxDets=100 ] = 0.082\n",
+ " Average Recall (AR) @[ IoU=0.50:0.95 | area=medium | maxDets=100 ] = 0.262\n",
+ " Average Recall (AR) @[ IoU=0.50:0.95 | area= large | maxDets=100 ] = 0.512\n",
+ "4138/4138 [==============================] - 606s 146ms/step - loss: 0.5503 - box_loss: 0.5076 - class_loss: 0.0426 - val_loss: 1.2389 - val_box_loss: 1.1706 - val_class_loss: 0.0683 - val_AP: 0.3449 - val_AP50: 0.4971 - val_AP75: 0.3754 - val_APs: 0.0499 - val_APm: 0.1657 - val_APl: 0.4042 - val_ARmax1: 0.3430 - val_ARmax10: 0.4501 - val_ARmax100: 0.4533 - val_ARs: 0.0816 - val_ARm: 0.2619 - val_ARl: 0.5118\n",
+ "Epoch 87/120\n",
+ "1238/1238 [==============================] - 111s 89ms/step\n",
+ "creating index...\n",
+ "index created!\n",
+ "creating index...\n",
+ "index created!\n",
+ "Running per image evaluation...\n",
+ "Evaluate annotation type *bbox*\n",
+ "DONE (t=5.45s).\n",
+ "Accumulating evaluation results...\n",
+ "DONE (t=1.03s).\n",
+ " Average Precision (AP) @[ IoU=0.50:0.95 | area= all | maxDets=100 ] = 0.345\n",
+ " Average Precision (AP) @[ IoU=0.50 | area= all | maxDets=100 ] = 0.495\n",
+ " Average Precision (AP) @[ IoU=0.75 | area= all | maxDets=100 ] = 0.378\n",
+ " Average Precision (AP) @[ IoU=0.50:0.95 | area= small | maxDets=100 ] = 0.057\n",
+ " Average Precision (AP) @[ IoU=0.50:0.95 | area=medium | maxDets=100 ] = 0.164\n",
+ " Average Precision (AP) @[ IoU=0.50:0.95 | area= large | maxDets=100 ] = 0.406\n",
+ " Average Recall (AR) @[ IoU=0.50:0.95 | area= all | maxDets= 1 ] = 0.346\n",
+ " Average Recall (AR) @[ IoU=0.50:0.95 | area= all | maxDets= 10 ] = 0.451\n",
+ " Average Recall (AR) @[ IoU=0.50:0.95 | area= all | maxDets=100 ] = 0.454\n",
+ " Average Recall (AR) @[ IoU=0.50:0.95 | area= small | maxDets=100 ] = 0.090\n",
+ " Average Recall (AR) @[ IoU=0.50:0.95 | area=medium | maxDets=100 ] = 0.258\n",
+ " Average Recall (AR) @[ IoU=0.50:0.95 | area= large | maxDets=100 ] = 0.515\n",
+ "4138/4138 [==============================] - 605s 146ms/step - loss: 0.5344 - box_loss: 0.4928 - class_loss: 0.0416 - val_loss: 1.2376 - val_box_loss: 1.1695 - val_class_loss: 0.0681 - val_AP: 0.3455 - val_AP50: 0.4952 - val_AP75: 0.3783 - val_APs: 0.0566 - val_APm: 0.1644 - val_APl: 0.4061 - val_ARmax1: 0.3458 - val_ARmax10: 0.4510 - val_ARmax100: 0.4537 - val_ARs: 0.0900 - val_ARm: 0.2579 - val_ARl: 0.5151\n",
+ "Epoch 88/120\n",
+ "1238/1238 [==============================] - 111s 89ms/step\n",
+ "creating index...\n",
+ "index created!\n",
+ "creating index...\n",
+ "index created!\n",
+ "Running per image evaluation...\n",
+ "Evaluate annotation type *bbox*\n",
+ "DONE (t=4.88s).\n",
+ "Accumulating evaluation results...\n",
+ "DONE (t=1.03s).\n",
+ " Average Precision (AP) @[ IoU=0.50:0.95 | area= all | maxDets=100 ] = 0.350\n",
+ " Average Precision (AP) @[ IoU=0.50 | area= all | maxDets=100 ] = 0.501\n",
+ " Average Precision (AP) @[ IoU=0.75 | area= all | maxDets=100 ] = 0.384\n",
+ " Average Precision (AP) @[ IoU=0.50:0.95 | area= small | maxDets=100 ] = 0.056\n",
+ " Average Precision (AP) @[ IoU=0.50:0.95 | area=medium | maxDets=100 ] = 0.173\n",
+ " Average Precision (AP) @[ IoU=0.50:0.95 | area= large | maxDets=100 ] = 0.410\n",
+ " Average Recall (AR) @[ IoU=0.50:0.95 | area= all | maxDets= 1 ] = 0.344\n",
+ " Average Recall (AR) @[ IoU=0.50:0.95 | area= all | maxDets= 10 ] = 0.454\n",
+ " Average Recall (AR) @[ IoU=0.50:0.95 | area= all | maxDets=100 ] = 0.457\n",
+ " Average Recall (AR) @[ IoU=0.50:0.95 | area= small | maxDets=100 ] = 0.095\n",
+ " Average Recall (AR) @[ IoU=0.50:0.95 | area=medium | maxDets=100 ] = 0.261\n",
+ " Average Recall (AR) @[ IoU=0.50:0.95 | area= large | maxDets=100 ] = 0.516\n",
+ "4138/4138 [==============================] - 605s 146ms/step - loss: 0.5402 - box_loss: 0.4982 - class_loss: 0.0420 - val_loss: 1.2366 - val_box_loss: 1.1678 - val_class_loss: 0.0689 - val_AP: 0.3502 - val_AP50: 0.5007 - val_AP75: 0.3838 - val_APs: 0.0559 - val_APm: 0.1730 - val_APl: 0.4095 - val_ARmax1: 0.3443 - val_ARmax10: 0.4539 - val_ARmax100: 0.4571 - val_ARs: 0.0948 - val_ARm: 0.2613 - val_ARl: 0.5160\n",
+ "Epoch 89/120\n",
+ "1238/1238 [==============================] - 111s 89ms/step\n",
+ "creating index...\n",
+ "index created!\n",
+ "creating index...\n",
+ "index created!\n",
+ "Running per image evaluation...\n",
+ "Evaluate annotation type *bbox*\n",
+ "DONE (t=5.39s).\n",
+ "Accumulating evaluation results...\n",
+ "DONE (t=1.02s).\n",
+ " Average Precision (AP) @[ IoU=0.50:0.95 | area= all | maxDets=100 ] = 0.352\n",
+ " Average Precision (AP) @[ IoU=0.50 | area= all | maxDets=100 ] = 0.502\n",
+ " Average Precision (AP) @[ IoU=0.75 | area= all | maxDets=100 ] = 0.384\n",
+ " Average Precision (AP) @[ IoU=0.50:0.95 | area= small | maxDets=100 ] = 0.053\n",
+ " Average Precision (AP) @[ IoU=0.50:0.95 | area=medium | maxDets=100 ] = 0.173\n",
+ " Average Precision (AP) @[ IoU=0.50:0.95 | area= large | maxDets=100 ] = 0.414\n",
+ " Average Recall (AR) @[ IoU=0.50:0.95 | area= all | maxDets= 1 ] = 0.346\n",
+ " Average Recall (AR) @[ IoU=0.50:0.95 | area= all | maxDets= 10 ] = 0.454\n",
+ " Average Recall (AR) @[ IoU=0.50:0.95 | area= all | maxDets=100 ] = 0.457\n",
+ " Average Recall (AR) @[ IoU=0.50:0.95 | area= small | maxDets=100 ] = 0.090\n",
+ " Average Recall (AR) @[ IoU=0.50:0.95 | area=medium | maxDets=100 ] = 0.264\n",
+ " Average Recall (AR) @[ IoU=0.50:0.95 | area= large | maxDets=100 ] = 0.519\n",
+ "4138/4138 [==============================] - 605s 146ms/step - loss: 0.5330 - box_loss: 0.4915 - class_loss: 0.0415 - val_loss: 1.2327 - val_box_loss: 1.1649 - val_class_loss: 0.0678 - val_AP: 0.3521 - val_AP50: 0.5021 - val_AP75: 0.3835 - val_APs: 0.0533 - val_APm: 0.1735 - val_APl: 0.4138 - val_ARmax1: 0.3465 - val_ARmax10: 0.4542 - val_ARmax100: 0.4573 - val_ARs: 0.0900 - val_ARm: 0.2637 - val_ARl: 0.5195\n",
+ "Epoch 90/120\n",
+ "1238/1238 [==============================] - 111s 89ms/step\n",
+ "creating index...\n",
+ "index created!\n",
+ "creating index...\n",
+ "index created!\n",
+ "Running per image evaluation...\n",
+ "Evaluate annotation type *bbox*\n",
+ "DONE (t=4.78s).\n",
+ "Accumulating evaluation results...\n",
+ "DONE (t=1.03s).\n",
+ " Average Precision (AP) @[ IoU=0.50:0.95 | area= all | maxDets=100 ] = 0.344\n",
+ " Average Precision (AP) @[ IoU=0.50 | area= all | maxDets=100 ] = 0.492\n",
+ " Average Precision (AP) @[ IoU=0.75 | area= all | maxDets=100 ] = 0.375\n",
+ " Average Precision (AP) @[ IoU=0.50:0.95 | area= small | maxDets=100 ] = 0.055\n",
+ " Average Precision (AP) @[ IoU=0.50:0.95 | area=medium | maxDets=100 ] = 0.168\n",
+ " Average Precision (AP) @[ IoU=0.50:0.95 | area= large | maxDets=100 ] = 0.403\n",
+ " Average Recall (AR) @[ IoU=0.50:0.95 | area= all | maxDets= 1 ] = 0.342\n",
+ " Average Recall (AR) @[ IoU=0.50:0.95 | area= all | maxDets= 10 ] = 0.447\n",
+ " Average Recall (AR) @[ IoU=0.50:0.95 | area= all | maxDets=100 ] = 0.450\n",
+ " Average Recall (AR) @[ IoU=0.50:0.95 | area= small | maxDets=100 ] = 0.086\n",
+ " Average Recall (AR) @[ IoU=0.50:0.95 | area=medium | maxDets=100 ] = 0.262\n",
+ " Average Recall (AR) @[ IoU=0.50:0.95 | area= large | maxDets=100 ] = 0.509\n",
+ "4138/4138 [==============================] - 605s 146ms/step - loss: 0.5319 - box_loss: 0.4906 - class_loss: 0.0413 - val_loss: 1.2377 - val_box_loss: 1.1694 - val_class_loss: 0.0683 - val_AP: 0.3443 - val_AP50: 0.4917 - val_AP75: 0.3753 - val_APs: 0.0552 - val_APm: 0.1675 - val_APl: 0.4035 - val_ARmax1: 0.3421 - val_ARmax10: 0.4473 - val_ARmax100: 0.4501 - val_ARs: 0.0860 - val_ARm: 0.2620 - val_ARl: 0.5091\n",
+ "Epoch 91/120\n",
+ "1238/1238 [==============================] - 111s 89ms/step\n",
+ "creating index...\n",
+ "index created!\n",
+ "creating index...\n",
+ "index created!\n",
+ "Running per image evaluation...\n",
+ "Evaluate annotation type *bbox*\n",
+ "DONE (t=5.46s).\n",
+ "Accumulating evaluation results...\n",
+ "DONE (t=1.05s).\n",
+ " Average Precision (AP) @[ IoU=0.50:0.95 | area= all | maxDets=100 ] = 0.348\n",
+ " Average Precision (AP) @[ IoU=0.50 | area= all | maxDets=100 ] = 0.498\n",
+ " Average Precision (AP) @[ IoU=0.75 | area= all | maxDets=100 ] = 0.378\n",
+ " Average Precision (AP) @[ IoU=0.50:0.95 | area= small | maxDets=100 ] = 0.066\n",
+ " Average Precision (AP) @[ IoU=0.50:0.95 | area=medium | maxDets=100 ] = 0.177\n",
+ " Average Precision (AP) @[ IoU=0.50:0.95 | area= large | maxDets=100 ] = 0.406\n",
+ " Average Recall (AR) @[ IoU=0.50:0.95 | area= all | maxDets= 1 ] = 0.345\n",
+ " Average Recall (AR) @[ IoU=0.50:0.95 | area= all | maxDets= 10 ] = 0.452\n",
+ " Average Recall (AR) @[ IoU=0.50:0.95 | area= all | maxDets=100 ] = 0.455\n",
+ " Average Recall (AR) @[ IoU=0.50:0.95 | area= small | maxDets=100 ] = 0.099\n",
+ " Average Recall (AR) @[ IoU=0.50:0.95 | area=medium | maxDets=100 ] = 0.269\n",
+ " Average Recall (AR) @[ IoU=0.50:0.95 | area= large | maxDets=100 ] = 0.513\n",
+ "4138/4138 [==============================] - 606s 146ms/step - loss: 0.5280 - box_loss: 0.4871 - class_loss: 0.0408 - val_loss: 1.2316 - val_box_loss: 1.1638 - val_class_loss: 0.0678 - val_AP: 0.3476 - val_AP50: 0.4978 - val_AP75: 0.3778 - val_APs: 0.0661 - val_APm: 0.1771 - val_APl: 0.4058 - val_ARmax1: 0.3447 - val_ARmax10: 0.4524 - val_ARmax100: 0.4555 - val_ARs: 0.0989 - val_ARm: 0.2688 - val_ARl: 0.5135\n",
+ "Epoch 92/120\n",
+ "1238/1238 [==============================] - 111s 89ms/step\n",
+ "creating index...\n",
+ "index created!\n",
+ "creating index...\n",
+ "index created!\n",
+ "Running per image evaluation...\n",
+ "Evaluate annotation type *bbox*\n",
+ "DONE (t=4.81s).\n",
+ "Accumulating evaluation results...\n",
+ "DONE (t=1.02s).\n",
+ " Average Precision (AP) @[ IoU=0.50:0.95 | area= all | maxDets=100 ] = 0.350\n",
+ " Average Precision (AP) @[ IoU=0.50 | area= all | maxDets=100 ] = 0.501\n",
+ " Average Precision (AP) @[ IoU=0.75 | area= all | maxDets=100 ] = 0.381\n",
+ " Average Precision (AP) @[ IoU=0.50:0.95 | area= small | maxDets=100 ] = 0.045\n",
+ " Average Precision (AP) @[ IoU=0.50:0.95 | area=medium | maxDets=100 ] = 0.169\n",
+ " Average Precision (AP) @[ IoU=0.50:0.95 | area= large | maxDets=100 ] = 0.412\n",
+ " Average Recall (AR) @[ IoU=0.50:0.95 | area= all | maxDets= 1 ] = 0.349\n",
+ " Average Recall (AR) @[ IoU=0.50:0.95 | area= all | maxDets= 10 ] = 0.453\n",
+ " Average Recall (AR) @[ IoU=0.50:0.95 | area= all | maxDets=100 ] = 0.457\n",
+ " Average Recall (AR) @[ IoU=0.50:0.95 | area= small | maxDets=100 ] = 0.076\n",
+ " Average Recall (AR) @[ IoU=0.50:0.95 | area=medium | maxDets=100 ] = 0.259\n",
+ " Average Recall (AR) @[ IoU=0.50:0.95 | area= large | maxDets=100 ] = 0.518\n",
+ "4138/4138 [==============================] - 604s 146ms/step - loss: 0.5164 - box_loss: 0.4762 - class_loss: 0.0401 - val_loss: 1.2365 - val_box_loss: 1.1685 - val_class_loss: 0.0680 - val_AP: 0.3503 - val_AP50: 0.5005 - val_AP75: 0.3806 - val_APs: 0.0452 - val_APm: 0.1687 - val_APl: 0.4116 - val_ARmax1: 0.3491 - val_ARmax10: 0.4535 - val_ARmax100: 0.4568 - val_ARs: 0.0756 - val_ARm: 0.2590 - val_ARl: 0.5183\n",
+ "Epoch 93/120\n",
+ "1238/1238 [==============================] - 111s 89ms/step\n",
+ "creating index...\n",
+ "index created!\n",
+ "creating index...\n",
+ "index created!\n",
+ "Running per image evaluation...\n",
+ "Evaluate annotation type *bbox*\n",
+ "DONE (t=5.38s).\n",
+ "Accumulating evaluation results...\n",
+ "DONE (t=1.01s).\n",
+ " Average Precision (AP) @[ IoU=0.50:0.95 | area= all | maxDets=100 ] = 0.350\n",
+ " Average Precision (AP) @[ IoU=0.50 | area= all | maxDets=100 ] = 0.504\n",
+ " Average Precision (AP) @[ IoU=0.75 | area= all | maxDets=100 ] = 0.382\n",
+ " Average Precision (AP) @[ IoU=0.50:0.95 | area= small | maxDets=100 ] = 0.050\n",
+ " Average Precision (AP) @[ IoU=0.50:0.95 | area=medium | maxDets=100 ] = 0.180\n",
+ " Average Precision (AP) @[ IoU=0.50:0.95 | area= large | maxDets=100 ] = 0.410\n",
+ " Average Recall (AR) @[ IoU=0.50:0.95 | area= all | maxDets= 1 ] = 0.348\n",
+ " Average Recall (AR) @[ IoU=0.50:0.95 | area= all | maxDets= 10 ] = 0.455\n",
+ " Average Recall (AR) @[ IoU=0.50:0.95 | area= all | maxDets=100 ] = 0.458\n",
+ " Average Recall (AR) @[ IoU=0.50:0.95 | area= small | maxDets=100 ] = 0.079\n",
+ " Average Recall (AR) @[ IoU=0.50:0.95 | area=medium | maxDets=100 ] = 0.267\n",
+ " Average Recall (AR) @[ IoU=0.50:0.95 | area= large | maxDets=100 ] = 0.518\n",
+ "4138/4138 [==============================] - 606s 146ms/step - loss: 0.5081 - box_loss: 0.4686 - class_loss: 0.0396 - val_loss: 1.2380 - val_box_loss: 1.1700 - val_class_loss: 0.0681 - val_AP: 0.3505 - val_AP50: 0.5036 - val_AP75: 0.3819 - val_APs: 0.0497 - val_APm: 0.1798 - val_APl: 0.4096 - val_ARmax1: 0.3479 - val_ARmax10: 0.4548 - val_ARmax100: 0.4581 - val_ARs: 0.0791 - val_ARm: 0.2672 - val_ARl: 0.5183\n",
+ "Epoch 94/120\n",
+ "1238/1238 [==============================] - 111s 89ms/step\n",
+ "creating index...\n",
+ "index created!\n",
+ "creating index...\n",
+ "index created!\n",
+ "Running per image evaluation...\n",
+ "Evaluate annotation type *bbox*\n",
+ "DONE (t=4.79s).\n",
+ "Accumulating evaluation results...\n",
+ "DONE (t=1.01s).\n",
+ " Average Precision (AP) @[ IoU=0.50:0.95 | area= all | maxDets=100 ] = 0.352\n",
+ " Average Precision (AP) @[ IoU=0.50 | area= all | maxDets=100 ] = 0.504\n",
+ " Average Precision (AP) @[ IoU=0.75 | area= all | maxDets=100 ] = 0.385\n",
+ " Average Precision (AP) @[ IoU=0.50:0.95 | area= small | maxDets=100 ] = 0.048\n",
+ " Average Precision (AP) @[ IoU=0.50:0.95 | area=medium | maxDets=100 ] = 0.172\n",
+ " Average Precision (AP) @[ IoU=0.50:0.95 | area= large | maxDets=100 ] = 0.414\n",
+ " Average Recall (AR) @[ IoU=0.50:0.95 | area= all | maxDets= 1 ] = 0.351\n",
+ " Average Recall (AR) @[ IoU=0.50:0.95 | area= all | maxDets= 10 ] = 0.457\n",
+ " Average Recall (AR) @[ IoU=0.50:0.95 | area= all | maxDets=100 ] = 0.460\n",
+ " Average Recall (AR) @[ IoU=0.50:0.95 | area= small | maxDets=100 ] = 0.085\n",
+ " Average Recall (AR) @[ IoU=0.50:0.95 | area=medium | maxDets=100 ] = 0.261\n",
+ " Average Recall (AR) @[ IoU=0.50:0.95 | area= large | maxDets=100 ] = 0.522\n",
+ "4138/4138 [==============================] - 605s 146ms/step - loss: 0.5134 - box_loss: 0.4735 - class_loss: 0.0399 - val_loss: 1.2370 - val_box_loss: 1.1695 - val_class_loss: 0.0675 - val_AP: 0.3524 - val_AP50: 0.5045 - val_AP75: 0.3850 - val_APs: 0.0475 - val_APm: 0.1719 - val_APl: 0.4141 - val_ARmax1: 0.3513 - val_ARmax10: 0.4569 - val_ARmax100: 0.4598 - val_ARs: 0.0847 - val_ARm: 0.2609 - val_ARl: 0.5220\n",
+ "Epoch 95/120\n",
+ "1238/1238 [==============================] - 111s 89ms/step\n",
+ "creating index...\n",
+ "index created!\n",
+ "creating index...\n",
+ "index created!\n",
+ "Running per image evaluation...\n",
+ "Evaluate annotation type *bbox*\n",
+ "DONE (t=5.46s).\n",
+ "Accumulating evaluation results...\n",
+ "DONE (t=1.03s).\n",
+ " Average Precision (AP) @[ IoU=0.50:0.95 | area= all | maxDets=100 ] = 0.355\n",
+ " Average Precision (AP) @[ IoU=0.50 | area= all | maxDets=100 ] = 0.507\n",
+ " Average Precision (AP) @[ IoU=0.75 | area= all | maxDets=100 ] = 0.387\n",
+ " Average Precision (AP) @[ IoU=0.50:0.95 | area= small | maxDets=100 ] = 0.063\n",
+ " Average Precision (AP) @[ IoU=0.50:0.95 | area=medium | maxDets=100 ] = 0.172\n",
+ " Average Precision (AP) @[ IoU=0.50:0.95 | area= large | maxDets=100 ] = 0.416\n",
+ " Average Recall (AR) @[ IoU=0.50:0.95 | area= all | maxDets= 1 ] = 0.350\n",
+ " Average Recall (AR) @[ IoU=0.50:0.95 | area= all | maxDets= 10 ] = 0.459\n",
+ " Average Recall (AR) @[ IoU=0.50:0.95 | area= all | maxDets=100 ] = 0.463\n",
+ " Average Recall (AR) @[ IoU=0.50:0.95 | area= small | maxDets=100 ] = 0.099\n",
+ " Average Recall (AR) @[ IoU=0.50:0.95 | area=medium | maxDets=100 ] = 0.267\n",
+ " Average Recall (AR) @[ IoU=0.50:0.95 | area= large | maxDets=100 ] = 0.523\n",
+ "4138/4138 [==============================] - 606s 146ms/step - loss: 0.5109 - box_loss: 0.4711 - class_loss: 0.0398 - val_loss: 1.2341 - val_box_loss: 1.1667 - val_class_loss: 0.0674 - val_AP: 0.3548 - val_AP50: 0.5072 - val_AP75: 0.3872 - val_APs: 0.0630 - val_APm: 0.1716 - val_APl: 0.4156 - val_ARmax1: 0.3501 - val_ARmax10: 0.4590 - val_ARmax100: 0.4625 - val_ARs: 0.0989 - val_ARm: 0.2670 - val_ARl: 0.5229\n",
+ "Epoch 96/120\n",
+ "1238/1238 [==============================] - 111s 89ms/step\n",
+ "creating index...\n",
+ "index created!\n",
+ "creating index...\n",
+ "index created!\n",
+ "Running per image evaluation...\n",
+ "Evaluate annotation type *bbox*\n",
+ "DONE (t=4.78s).\n",
+ "Accumulating evaluation results...\n",
+ "DONE (t=1.02s).\n",
+ " Average Precision (AP) @[ IoU=0.50:0.95 | area= all | maxDets=100 ] = 0.350\n",
+ " Average Precision (AP) @[ IoU=0.50 | area= all | maxDets=100 ] = 0.500\n",
+ " Average Precision (AP) @[ IoU=0.75 | area= all | maxDets=100 ] = 0.381\n",
+ " Average Precision (AP) @[ IoU=0.50:0.95 | area= small | maxDets=100 ] = 0.054\n",
+ " Average Precision (AP) @[ IoU=0.50:0.95 | area=medium | maxDets=100 ] = 0.176\n",
+ " Average Precision (AP) @[ IoU=0.50:0.95 | area= large | maxDets=100 ] = 0.411\n",
+ " Average Recall (AR) @[ IoU=0.50:0.95 | area= all | maxDets= 1 ] = 0.349\n",
+ " Average Recall (AR) @[ IoU=0.50:0.95 | area= all | maxDets= 10 ] = 0.457\n",
+ " Average Recall (AR) @[ IoU=0.50:0.95 | area= all | maxDets=100 ] = 0.460\n",
+ " Average Recall (AR) @[ IoU=0.50:0.95 | area= small | maxDets=100 ] = 0.088\n",
+ " Average Recall (AR) @[ IoU=0.50:0.95 | area=medium | maxDets=100 ] = 0.265\n",
+ " Average Recall (AR) @[ IoU=0.50:0.95 | area= large | maxDets=100 ] = 0.521\n",
+ "4138/4138 [==============================] - 605s 146ms/step - loss: 0.4990 - box_loss: 0.4599 - class_loss: 0.0391 - val_loss: 1.2384 - val_box_loss: 1.1706 - val_class_loss: 0.0678 - val_AP: 0.3498 - val_AP50: 0.5001 - val_AP75: 0.3811 - val_APs: 0.0537 - val_APm: 0.1756 - val_APl: 0.4107 - val_ARmax1: 0.3495 - val_ARmax10: 0.4566 - val_ARmax100: 0.4595 - val_ARs: 0.0884 - val_ARm: 0.2649 - val_ARl: 0.5205\n",
+ "Epoch 97/120\n",
+ "1238/1238 [==============================] - 111s 89ms/step\n",
+ "creating index...\n",
+ "index created!\n",
+ "creating index...\n",
+ "index created!\n",
+ "Running per image evaluation...\n",
+ "Evaluate annotation type *bbox*\n",
+ "DONE (t=5.39s).\n",
+ "Accumulating evaluation results...\n",
+ "DONE (t=1.01s).\n",
+ " Average Precision (AP) @[ IoU=0.50:0.95 | area= all | maxDets=100 ] = 0.354\n",
+ " Average Precision (AP) @[ IoU=0.50 | area= all | maxDets=100 ] = 0.508\n",
+ " Average Precision (AP) @[ IoU=0.75 | area= all | maxDets=100 ] = 0.387\n",
+ " Average Precision (AP) @[ IoU=0.50:0.95 | area= small | maxDets=100 ] = 0.066\n",
+ " Average Precision (AP) @[ IoU=0.50:0.95 | area=medium | maxDets=100 ] = 0.175\n",
+ " Average Precision (AP) @[ IoU=0.50:0.95 | area= large | maxDets=100 ] = 0.415\n",
+ " Average Recall (AR) @[ IoU=0.50:0.95 | area= all | maxDets= 1 ] = 0.349\n",
+ " Average Recall (AR) @[ IoU=0.50:0.95 | area= all | maxDets= 10 ] = 0.458\n",
+ " Average Recall (AR) @[ IoU=0.50:0.95 | area= all | maxDets=100 ] = 0.461\n",
+ " Average Recall (AR) @[ IoU=0.50:0.95 | area= small | maxDets=100 ] = 0.098\n",
+ " Average Recall (AR) @[ IoU=0.50:0.95 | area=medium | maxDets=100 ] = 0.265\n",
+ " Average Recall (AR) @[ IoU=0.50:0.95 | area= large | maxDets=100 ] = 0.522\n",
+ "4138/4138 [==============================] - 607s 146ms/step - loss: 0.4921 - box_loss: 0.4534 - class_loss: 0.0387 - val_loss: 1.2402 - val_box_loss: 1.1727 - val_class_loss: 0.0675 - val_AP: 0.3539 - val_AP50: 0.5078 - val_AP75: 0.3872 - val_APs: 0.0656 - val_APm: 0.1753 - val_APl: 0.4152 - val_ARmax1: 0.3493 - val_ARmax10: 0.4578 - val_ARmax100: 0.4610 - val_ARs: 0.0976 - val_ARm: 0.2654 - val_ARl: 0.5223\n",
+ "Epoch 98/120\n",
+ "1238/1238 [==============================] - 111s 89ms/step\n",
+ "creating index...\n",
+ "index created!\n",
+ "creating index...\n",
+ "index created!\n",
+ "Running per image evaluation...\n",
+ "Evaluate annotation type *bbox*\n",
+ "DONE (t=4.71s).\n",
+ "Accumulating evaluation results...\n",
+ "DONE (t=1.00s).\n",
+ " Average Precision (AP) @[ IoU=0.50:0.95 | area= all | maxDets=100 ] = 0.344\n",
+ " Average Precision (AP) @[ IoU=0.50 | area= all | maxDets=100 ] = 0.493\n",
+ " Average Precision (AP) @[ IoU=0.75 | area= all | maxDets=100 ] = 0.377\n",
+ " Average Precision (AP) @[ IoU=0.50:0.95 | area= small | maxDets=100 ] = 0.058\n",
+ " Average Precision (AP) @[ IoU=0.50:0.95 | area=medium | maxDets=100 ] = 0.169\n",
+ " Average Precision (AP) @[ IoU=0.50:0.95 | area= large | maxDets=100 ] = 0.405\n",
+ " Average Recall (AR) @[ IoU=0.50:0.95 | area= all | maxDets= 1 ] = 0.343\n",
+ " Average Recall (AR) @[ IoU=0.50:0.95 | area= all | maxDets= 10 ] = 0.448\n",
+ " Average Recall (AR) @[ IoU=0.50:0.95 | area= all | maxDets=100 ] = 0.451\n",
+ " Average Recall (AR) @[ IoU=0.50:0.95 | area= small | maxDets=100 ] = 0.094\n",
+ " Average Recall (AR) @[ IoU=0.50:0.95 | area=medium | maxDets=100 ] = 0.262\n",
+ " Average Recall (AR) @[ IoU=0.50:0.95 | area= large | maxDets=100 ] = 0.512\n",
+ "4138/4138 [==============================] - 605s 146ms/step - loss: 0.4868 - box_loss: 0.4485 - class_loss: 0.0383 - val_loss: 1.2434 - val_box_loss: 1.1750 - val_class_loss: 0.0684 - val_AP: 0.3445 - val_AP50: 0.4929 - val_AP75: 0.3775 - val_APs: 0.0578 - val_APm: 0.1694 - val_APl: 0.4052 - val_ARmax1: 0.3435 - val_ARmax10: 0.4482 - val_ARmax100: 0.4511 - val_ARs: 0.0943 - val_ARm: 0.2622 - val_ARl: 0.5122\n",
+ "Epoch 99/120\n",
+ "1238/1238 [==============================] - 111s 89ms/step\n",
+ "creating index...\n",
+ "index created!\n",
+ "creating index...\n",
+ "index created!\n",
+ "Running per image evaluation...\n",
+ "Evaluate annotation type *bbox*\n",
+ "DONE (t=4.87s).\n",
+ "Accumulating evaluation results...\n",
+ "DONE (t=1.04s).\n",
+ " Average Precision (AP) @[ IoU=0.50:0.95 | area= all | maxDets=100 ] = 0.358\n",
+ " Average Precision (AP) @[ IoU=0.50 | area= all | maxDets=100 ] = 0.513\n",
+ " Average Precision (AP) @[ IoU=0.75 | area= all | maxDets=100 ] = 0.389\n",
+ " Average Precision (AP) @[ IoU=0.50:0.95 | area= small | maxDets=100 ] = 0.057\n",
+ " Average Precision (AP) @[ IoU=0.50:0.95 | area=medium | maxDets=100 ] = 0.176\n",
+ " Average Precision (AP) @[ IoU=0.50:0.95 | area= large | maxDets=100 ] = 0.421\n",
+ " Average Recall (AR) @[ IoU=0.50:0.95 | area= all | maxDets= 1 ] = 0.354\n",
+ " Average Recall (AR) @[ IoU=0.50:0.95 | area= all | maxDets= 10 ] = 0.466\n",
+ " Average Recall (AR) @[ IoU=0.50:0.95 | area= all | maxDets=100 ] = 0.469\n",
+ " Average Recall (AR) @[ IoU=0.50:0.95 | area= small | maxDets=100 ] = 0.096\n",
+ " Average Recall (AR) @[ IoU=0.50:0.95 | area=medium | maxDets=100 ] = 0.267\n",
+ " Average Recall (AR) @[ IoU=0.50:0.95 | area= large | maxDets=100 ] = 0.531\n",
+ "4138/4138 [==============================] - 606s 146ms/step - loss: 0.4826 - box_loss: 0.4446 - class_loss: 0.0380 - val_loss: 1.2316 - val_box_loss: 1.1647 - val_class_loss: 0.0669 - val_AP: 0.3583 - val_AP50: 0.5129 - val_AP75: 0.3888 - val_APs: 0.0567 - val_APm: 0.1757 - val_APl: 0.4208 - val_ARmax1: 0.3545 - val_ARmax10: 0.4657 - val_ARmax100: 0.4688 - val_ARs: 0.0961 - val_ARm: 0.2671 - val_ARl: 0.5308\n",
+ "Epoch 100/120\n",
+ "1238/1238 [==============================] - 111s 89ms/step\n",
+ "creating index...\n",
+ "index created!\n",
+ "creating index...\n",
+ "index created!\n",
+ "Running per image evaluation...\n",
+ "Evaluate annotation type *bbox*\n",
+ "DONE (t=4.79s).\n",
+ "Accumulating evaluation results...\n",
+ "DONE (t=1.01s).\n",
+ " Average Precision (AP) @[ IoU=0.50:0.95 | area= all | maxDets=100 ] = 0.354\n",
+ " Average Precision (AP) @[ IoU=0.50 | area= all | maxDets=100 ] = 0.507\n",
+ " Average Precision (AP) @[ IoU=0.75 | area= all | maxDets=100 ] = 0.384\n",
+ " Average Precision (AP) @[ IoU=0.50:0.95 | area= small | maxDets=100 ] = 0.052\n",
+ " Average Precision (AP) @[ IoU=0.50:0.95 | area=medium | maxDets=100 ] = 0.176\n",
+ " Average Precision (AP) @[ IoU=0.50:0.95 | area= large | maxDets=100 ] = 0.417\n",
+ " Average Recall (AR) @[ IoU=0.50:0.95 | area= all | maxDets= 1 ] = 0.350\n",
+ " Average Recall (AR) @[ IoU=0.50:0.95 | area= all | maxDets= 10 ] = 0.460\n",
+ " Average Recall (AR) @[ IoU=0.50:0.95 | area= all | maxDets=100 ] = 0.464\n",
+ " Average Recall (AR) @[ IoU=0.50:0.95 | area= small | maxDets=100 ] = 0.092\n",
+ " Average Recall (AR) @[ IoU=0.50:0.95 | area=medium | maxDets=100 ] = 0.270\n",
+ " Average Recall (AR) @[ IoU=0.50:0.95 | area= large | maxDets=100 ] = 0.526\n",
+ "4138/4138 [==============================] - 605s 146ms/step - loss: 0.4918 - box_loss: 0.4532 - class_loss: 0.0386 - val_loss: 1.2328 - val_box_loss: 1.1654 - val_class_loss: 0.0674 - val_AP: 0.3544 - val_AP50: 0.5068 - val_AP75: 0.3843 - val_APs: 0.0516 - val_APm: 0.1761 - val_APl: 0.4166 - val_ARmax1: 0.3498 - val_ARmax10: 0.4603 - val_ARmax100: 0.4638 - val_ARs: 0.0921 - val_ARm: 0.2698 - val_ARl: 0.5261\n",
+ "Epoch 101/120\n",
+ "1238/1238 [==============================] - 111s 89ms/step\n",
+ "creating index...\n",
+ "index created!\n",
+ "creating index...\n",
+ "index created!\n",
+ "Running per image evaluation...\n",
+ "Evaluate annotation type *bbox*\n",
+ "DONE (t=4.71s).\n",
+ "Accumulating evaluation results...\n",
+ "DONE (t=1.00s).\n",
+ " Average Precision (AP) @[ IoU=0.50:0.95 | area= all | maxDets=100 ] = 0.353\n",
+ " Average Precision (AP) @[ IoU=0.50 | area= all | maxDets=100 ] = 0.503\n",
+ " Average Precision (AP) @[ IoU=0.75 | area= all | maxDets=100 ] = 0.384\n",
+ " Average Precision (AP) @[ IoU=0.50:0.95 | area= small | maxDets=100 ] = 0.057\n",
+ " Average Precision (AP) @[ IoU=0.50:0.95 | area=medium | maxDets=100 ] = 0.171\n",
+ " Average Precision (AP) @[ IoU=0.50:0.95 | area= large | maxDets=100 ] = 0.415\n",
+ " Average Recall (AR) @[ IoU=0.50:0.95 | area= all | maxDets= 1 ] = 0.349\n",
+ " Average Recall (AR) @[ IoU=0.50:0.95 | area= all | maxDets= 10 ] = 0.457\n",
+ " Average Recall (AR) @[ IoU=0.50:0.95 | area= all | maxDets=100 ] = 0.460\n",
+ " Average Recall (AR) @[ IoU=0.50:0.95 | area= small | maxDets=100 ] = 0.090\n",
+ " Average Recall (AR) @[ IoU=0.50:0.95 | area=medium | maxDets=100 ] = 0.266\n",
+ " Average Recall (AR) @[ IoU=0.50:0.95 | area= large | maxDets=100 ] = 0.522\n",
+ "4138/4138 [==============================] - 607s 146ms/step - loss: 0.4804 - box_loss: 0.4427 - class_loss: 0.0378 - val_loss: 1.2321 - val_box_loss: 1.1649 - val_class_loss: 0.0672 - val_AP: 0.3532 - val_AP50: 0.5033 - val_AP75: 0.3844 - val_APs: 0.0570 - val_APm: 0.1708 - val_APl: 0.4151 - val_ARmax1: 0.3490 - val_ARmax10: 0.4572 - val_ARmax100: 0.4602 - val_ARs: 0.0903 - val_ARm: 0.2662 - val_ARl: 0.5223\n",
+ "Epoch 102/120\n",
+ "1238/1238 [==============================] - 111s 89ms/step\n",
+ "creating index...\n",
+ "index created!\n",
+ "creating index...\n",
+ "index created!\n",
+ "Running per image evaluation...\n",
+ "Evaluate annotation type *bbox*\n",
+ "DONE (t=4.80s).\n",
+ "Accumulating evaluation results...\n",
+ "DONE (t=1.01s).\n",
+ " Average Precision (AP) @[ IoU=0.50:0.95 | area= all | maxDets=100 ] = 0.358\n",
+ " Average Precision (AP) @[ IoU=0.50 | area= all | maxDets=100 ] = 0.511\n",
+ " Average Precision (AP) @[ IoU=0.75 | area= all | maxDets=100 ] = 0.393\n",
+ " Average Precision (AP) @[ IoU=0.50:0.95 | area= small | maxDets=100 ] = 0.061\n",
+ " Average Precision (AP) @[ IoU=0.50:0.95 | area=medium | maxDets=100 ] = 0.172\n",
+ " Average Precision (AP) @[ IoU=0.50:0.95 | area= large | maxDets=100 ] = 0.421\n",
+ " Average Recall (AR) @[ IoU=0.50:0.95 | area= all | maxDets= 1 ] = 0.353\n",
+ " Average Recall (AR) @[ IoU=0.50:0.95 | area= all | maxDets= 10 ] = 0.462\n",
+ " Average Recall (AR) @[ IoU=0.50:0.95 | area= all | maxDets=100 ] = 0.465\n",
+ " Average Recall (AR) @[ IoU=0.50:0.95 | area= small | maxDets=100 ] = 0.098\n",
+ " Average Recall (AR) @[ IoU=0.50:0.95 | area=medium | maxDets=100 ] = 0.268\n",
+ " Average Recall (AR) @[ IoU=0.50:0.95 | area= large | maxDets=100 ] = 0.527\n",
+ "4138/4138 [==============================] - 605s 146ms/step - loss: 0.4729 - box_loss: 0.4358 - class_loss: 0.0372 - val_loss: 1.2324 - val_box_loss: 1.1654 - val_class_loss: 0.0669 - val_AP: 0.3580 - val_AP50: 0.5106 - val_AP75: 0.3927 - val_APs: 0.0614 - val_APm: 0.1716 - val_APl: 0.4214 - val_ARmax1: 0.3530 - val_ARmax10: 0.4619 - val_ARmax100: 0.4649 - val_ARs: 0.0981 - val_ARm: 0.2679 - val_ARl: 0.5272\n",
+ "Epoch 103/120\n",
+ "1238/1238 [==============================] - 111s 89ms/step\n",
+ "creating index...\n",
+ "index created!\n",
+ "creating index...\n",
+ "index created!\n",
+ "Running per image evaluation...\n",
+ "Evaluate annotation type *bbox*\n",
+ "DONE (t=4.83s).\n",
+ "Accumulating evaluation results...\n",
+ "DONE (t=1.03s).\n",
+ " Average Precision (AP) @[ IoU=0.50:0.95 | area= all | maxDets=100 ] = 0.356\n",
+ " Average Precision (AP) @[ IoU=0.50 | area= all | maxDets=100 ] = 0.510\n",
+ " Average Precision (AP) @[ IoU=0.75 | area= all | maxDets=100 ] = 0.389\n",
+ " Average Precision (AP) @[ IoU=0.50:0.95 | area= small | maxDets=100 ] = 0.058\n",
+ " Average Precision (AP) @[ IoU=0.50:0.95 | area=medium | maxDets=100 ] = 0.171\n",
+ " Average Precision (AP) @[ IoU=0.50:0.95 | area= large | maxDets=100 ] = 0.416\n",
+ " Average Recall (AR) @[ IoU=0.50:0.95 | area= all | maxDets= 1 ] = 0.348\n",
+ " Average Recall (AR) @[ IoU=0.50:0.95 | area= all | maxDets= 10 ] = 0.459\n",
+ " Average Recall (AR) @[ IoU=0.50:0.95 | area= all | maxDets=100 ] = 0.462\n",
+ " Average Recall (AR) @[ IoU=0.50:0.95 | area= small | maxDets=100 ] = 0.102\n",
+ " Average Recall (AR) @[ IoU=0.50:0.95 | area=medium | maxDets=100 ] = 0.269\n",
+ " Average Recall (AR) @[ IoU=0.50:0.95 | area= large | maxDets=100 ] = 0.521\n",
+ "4138/4138 [==============================] - 606s 146ms/step - loss: 0.4819 - box_loss: 0.4441 - class_loss: 0.0378 - val_loss: 1.2363 - val_box_loss: 1.1692 - val_class_loss: 0.0670 - val_AP: 0.3555 - val_AP50: 0.5102 - val_AP75: 0.3889 - val_APs: 0.0580 - val_APm: 0.1713 - val_APl: 0.4162 - val_ARmax1: 0.3481 - val_ARmax10: 0.4588 - val_ARmax100: 0.4621 - val_ARs: 0.1024 - val_ARm: 0.2693 - val_ARl: 0.5205\n",
+ "Epoch 104/120\n",
+ "1238/1238 [==============================] - 111s 89ms/step\n",
+ "creating index...\n",
+ "index created!\n",
+ "creating index...\n",
+ "index created!\n",
+ "Running per image evaluation...\n",
+ "Evaluate annotation type *bbox*\n",
+ "DONE (t=4.78s).\n",
+ "Accumulating evaluation results...\n",
+ "DONE (t=1.02s).\n",
+ " Average Precision (AP) @[ IoU=0.50:0.95 | area= all | maxDets=100 ] = 0.357\n",
+ " Average Precision (AP) @[ IoU=0.50 | area= all | maxDets=100 ] = 0.509\n",
+ " Average Precision (AP) @[ IoU=0.75 | area= all | maxDets=100 ] = 0.391\n",
+ " Average Precision (AP) @[ IoU=0.50:0.95 | area= small | maxDets=100 ] = 0.055\n",
+ " Average Precision (AP) @[ IoU=0.50:0.95 | area=medium | maxDets=100 ] = 0.174\n",
+ " Average Precision (AP) @[ IoU=0.50:0.95 | area= large | maxDets=100 ] = 0.419\n",
+ " Average Recall (AR) @[ IoU=0.50:0.95 | area= all | maxDets= 1 ] = 0.353\n",
+ " Average Recall (AR) @[ IoU=0.50:0.95 | area= all | maxDets= 10 ] = 0.462\n",
+ " Average Recall (AR) @[ IoU=0.50:0.95 | area= all | maxDets=100 ] = 0.465\n",
+ " Average Recall (AR) @[ IoU=0.50:0.95 | area= small | maxDets=100 ] = 0.094\n",
+ " Average Recall (AR) @[ IoU=0.50:0.95 | area=medium | maxDets=100 ] = 0.266\n",
+ " Average Recall (AR) @[ IoU=0.50:0.95 | area= large | maxDets=100 ] = 0.525\n",
+ "4138/4138 [==============================] - 605s 146ms/step - loss: 0.4663 - box_loss: 0.4296 - class_loss: 0.0367 - val_loss: 1.2301 - val_box_loss: 1.1631 - val_class_loss: 0.0670 - val_AP: 0.3572 - val_AP50: 0.5088 - val_AP75: 0.3912 - val_APs: 0.0551 - val_APm: 0.1741 - val_APl: 0.4193 - val_ARmax1: 0.3529 - val_ARmax10: 0.4617 - val_ARmax100: 0.4650 - val_ARs: 0.0936 - val_ARm: 0.2664 - val_ARl: 0.5254\n",
+ "Epoch 105/120\n",
+ "1238/1238 [==============================] - 111s 89ms/step\n",
+ "creating index...\n",
+ "index created!\n",
+ "creating index...\n",
+ "index created!\n",
+ "Running per image evaluation...\n",
+ "Evaluate annotation type *bbox*\n",
+ "DONE (t=5.37s).\n",
+ "Accumulating evaluation results...\n",
+ "DONE (t=1.02s).\n",
+ " Average Precision (AP) @[ IoU=0.50:0.95 | area= all | maxDets=100 ] = 0.358\n",
+ " Average Precision (AP) @[ IoU=0.50 | area= all | maxDets=100 ] = 0.510\n",
+ " Average Precision (AP) @[ IoU=0.75 | area= all | maxDets=100 ] = 0.391\n",
+ " Average Precision (AP) @[ IoU=0.50:0.95 | area= small | maxDets=100 ] = 0.064\n",
+ " Average Precision (AP) @[ IoU=0.50:0.95 | area=medium | maxDets=100 ] = 0.173\n",
+ " Average Precision (AP) @[ IoU=0.50:0.95 | area= large | maxDets=100 ] = 0.419\n",
+ " Average Recall (AR) @[ IoU=0.50:0.95 | area= all | maxDets= 1 ] = 0.353\n",
+ " Average Recall (AR) @[ IoU=0.50:0.95 | area= all | maxDets= 10 ] = 0.463\n",
+ " Average Recall (AR) @[ IoU=0.50:0.95 | area= all | maxDets=100 ] = 0.466\n",
+ " Average Recall (AR) @[ IoU=0.50:0.95 | area= small | maxDets=100 ] = 0.103\n",
+ " Average Recall (AR) @[ IoU=0.50:0.95 | area=medium | maxDets=100 ] = 0.271\n",
+ " Average Recall (AR) @[ IoU=0.50:0.95 | area= large | maxDets=100 ] = 0.525\n",
+ "4138/4138 [==============================] - 608s 147ms/step - loss: 0.4637 - box_loss: 0.4270 - class_loss: 0.0367 - val_loss: 1.2275 - val_box_loss: 1.1606 - val_class_loss: 0.0669 - val_AP: 0.3578 - val_AP50: 0.5102 - val_AP75: 0.3905 - val_APs: 0.0642 - val_APm: 0.1726 - val_APl: 0.4188 - val_ARmax1: 0.3532 - val_ARmax10: 0.4625 - val_ARmax100: 0.4657 - val_ARs: 0.1025 - val_ARm: 0.2708 - val_ARl: 0.5249\n",
+ "Epoch 106/120\n",
+ "1238/1238 [==============================] - 111s 89ms/step\n",
+ "creating index...\n",
+ "index created!\n",
+ "creating index...\n",
+ "index created!\n",
+ "Running per image evaluation...\n",
+ "Evaluate annotation type *bbox*\n",
+ "DONE (t=4.78s).\n",
+ "Accumulating evaluation results...\n",
+ "DONE (t=1.01s).\n",
+ " Average Precision (AP) @[ IoU=0.50:0.95 | area= all | maxDets=100 ] = 0.356\n",
+ " Average Precision (AP) @[ IoU=0.50 | area= all | maxDets=100 ] = 0.507\n",
+ " Average Precision (AP) @[ IoU=0.75 | area= all | maxDets=100 ] = 0.389\n",
+ " Average Precision (AP) @[ IoU=0.50:0.95 | area= small | maxDets=100 ] = 0.062\n",
+ " Average Precision (AP) @[ IoU=0.50:0.95 | area=medium | maxDets=100 ] = 0.175\n",
+ " Average Precision (AP) @[ IoU=0.50:0.95 | area= large | maxDets=100 ] = 0.418\n",
+ " Average Recall (AR) @[ IoU=0.50:0.95 | area= all | maxDets= 1 ] = 0.351\n",
+ " Average Recall (AR) @[ IoU=0.50:0.95 | area= all | maxDets= 10 ] = 0.459\n",
+ " Average Recall (AR) @[ IoU=0.50:0.95 | area= all | maxDets=100 ] = 0.462\n",
+ " Average Recall (AR) @[ IoU=0.50:0.95 | area= small | maxDets=100 ] = 0.101\n",
+ " Average Recall (AR) @[ IoU=0.50:0.95 | area=medium | maxDets=100 ] = 0.269\n",
+ " Average Recall (AR) @[ IoU=0.50:0.95 | area= large | maxDets=100 ] = 0.524\n",
+ "4138/4138 [==============================] - 606s 146ms/step - loss: 0.4600 - box_loss: 0.4236 - class_loss: 0.0364 - val_loss: 1.2348 - val_box_loss: 1.1677 - val_class_loss: 0.0672 - val_AP: 0.3556 - val_AP50: 0.5073 - val_AP75: 0.3890 - val_APs: 0.0616 - val_APm: 0.1752 - val_APl: 0.4182 - val_ARmax1: 0.3508 - val_ARmax10: 0.4590 - val_ARmax100: 0.4622 - val_ARs: 0.1007 - val_ARm: 0.2685 - val_ARl: 0.5242\n",
+ "Epoch 107/120\n",
+ "1238/1238 [==============================] - 111s 89ms/step\n",
+ "creating index...\n",
+ "index created!\n",
+ "creating index...\n",
+ "index created!\n",
+ "Running per image evaluation...\n",
+ "Evaluate annotation type *bbox*\n",
+ "DONE (t=4.80s).\n",
+ "Accumulating evaluation results...\n",
+ "DONE (t=1.01s).\n",
+ " Average Precision (AP) @[ IoU=0.50:0.95 | area= all | maxDets=100 ] = 0.354\n",
+ " Average Precision (AP) @[ IoU=0.50 | area= all | maxDets=100 ] = 0.504\n",
+ " Average Precision (AP) @[ IoU=0.75 | area= all | maxDets=100 ] = 0.387\n",
+ " Average Precision (AP) @[ IoU=0.50:0.95 | area= small | maxDets=100 ] = 0.060\n",
+ " Average Precision (AP) @[ IoU=0.50:0.95 | area=medium | maxDets=100 ] = 0.172\n",
+ " Average Precision (AP) @[ IoU=0.50:0.95 | area= large | maxDets=100 ] = 0.415\n",
+ " Average Recall (AR) @[ IoU=0.50:0.95 | area= all | maxDets= 1 ] = 0.352\n",
+ " Average Recall (AR) @[ IoU=0.50:0.95 | area= all | maxDets= 10 ] = 0.458\n",
+ " Average Recall (AR) @[ IoU=0.50:0.95 | area= all | maxDets=100 ] = 0.461\n",
+ " Average Recall (AR) @[ IoU=0.50:0.95 | area= small | maxDets=100 ] = 0.104\n",
+ " Average Recall (AR) @[ IoU=0.50:0.95 | area=medium | maxDets=100 ] = 0.267\n",
+ " Average Recall (AR) @[ IoU=0.50:0.95 | area= large | maxDets=100 ] = 0.520\n",
+ "4138/4138 [==============================] - 607s 146ms/step - loss: 0.4627 - box_loss: 0.4260 - class_loss: 0.0367 - val_loss: 1.2333 - val_box_loss: 1.1663 - val_class_loss: 0.0670 - val_AP: 0.3544 - val_AP50: 0.5044 - val_AP75: 0.3875 - val_APs: 0.0595 - val_APm: 0.1725 - val_APl: 0.4150 - val_ARmax1: 0.3516 - val_ARmax10: 0.4580 - val_ARmax100: 0.4609 - val_ARs: 0.1035 - val_ARm: 0.2668 - val_ARl: 0.5199\n",
+ "Epoch 108/120\n",
+ "1238/1238 [==============================] - 111s 89ms/step\n",
+ "creating index...\n",
+ "index created!\n",
+ "creating index...\n",
+ "index created!\n",
+ "Running per image evaluation...\n",
+ "Evaluate annotation type *bbox*\n",
+ "DONE (t=4.78s).\n",
+ "Accumulating evaluation results...\n",
+ "DONE (t=1.00s).\n",
+ " Average Precision (AP) @[ IoU=0.50:0.95 | area= all | maxDets=100 ] = 0.360\n",
+ " Average Precision (AP) @[ IoU=0.50 | area= all | maxDets=100 ] = 0.511\n",
+ " Average Precision (AP) @[ IoU=0.75 | area= all | maxDets=100 ] = 0.396\n",
+ " Average Precision (AP) @[ IoU=0.50:0.95 | area= small | maxDets=100 ] = 0.064\n",
+ " Average Precision (AP) @[ IoU=0.50:0.95 | area=medium | maxDets=100 ] = 0.178\n",
+ " Average Precision (AP) @[ IoU=0.50:0.95 | area= large | maxDets=100 ] = 0.423\n",
+ " Average Recall (AR) @[ IoU=0.50:0.95 | area= all | maxDets= 1 ] = 0.354\n",
+ " Average Recall (AR) @[ IoU=0.50:0.95 | area= all | maxDets= 10 ] = 0.462\n",
+ " Average Recall (AR) @[ IoU=0.50:0.95 | area= all | maxDets=100 ] = 0.465\n",
+ " Average Recall (AR) @[ IoU=0.50:0.95 | area= small | maxDets=100 ] = 0.102\n",
+ " Average Recall (AR) @[ IoU=0.50:0.95 | area=medium | maxDets=100 ] = 0.274\n",
+ " Average Recall (AR) @[ IoU=0.50:0.95 | area= large | maxDets=100 ] = 0.527\n",
+ "4138/4138 [==============================] - 606s 146ms/step - loss: 0.4550 - box_loss: 0.4189 - class_loss: 0.0361 - val_loss: 1.2288 - val_box_loss: 1.1625 - val_class_loss: 0.0663 - val_AP: 0.3601 - val_AP50: 0.5113 - val_AP75: 0.3955 - val_APs: 0.0640 - val_APm: 0.1775 - val_APl: 0.4229 - val_ARmax1: 0.3541 - val_ARmax10: 0.4622 - val_ARmax100: 0.4654 - val_ARs: 0.1020 - val_ARm: 0.2735 - val_ARl: 0.5272\n",
+ "Epoch 109/120\n",
+ "1238/1238 [==============================] - 111s 89ms/step\n",
+ "creating index...\n",
+ "index created!\n",
+ "creating index...\n",
+ "index created!\n",
+ "Running per image evaluation...\n",
+ "Evaluate annotation type *bbox*\n",
+ "DONE (t=4.80s).\n",
+ "Accumulating evaluation results...\n",
+ "DONE (t=1.02s).\n",
+ " Average Precision (AP) @[ IoU=0.50:0.95 | area= all | maxDets=100 ] = 0.358\n",
+ " Average Precision (AP) @[ IoU=0.50 | area= all | maxDets=100 ] = 0.511\n",
+ " Average Precision (AP) @[ IoU=0.75 | area= all | maxDets=100 ] = 0.389\n",
+ " Average Precision (AP) @[ IoU=0.50:0.95 | area= small | maxDets=100 ] = 0.057\n",
+ " Average Precision (AP) @[ IoU=0.50:0.95 | area=medium | maxDets=100 ] = 0.172\n",
+ " Average Precision (AP) @[ IoU=0.50:0.95 | area= large | maxDets=100 ] = 0.420\n",
+ " Average Recall (AR) @[ IoU=0.50:0.95 | area= all | maxDets= 1 ] = 0.350\n",
+ " Average Recall (AR) @[ IoU=0.50:0.95 | area= all | maxDets= 10 ] = 0.459\n",
+ " Average Recall (AR) @[ IoU=0.50:0.95 | area= all | maxDets=100 ] = 0.462\n",
+ " Average Recall (AR) @[ IoU=0.50:0.95 | area= small | maxDets=100 ] = 0.100\n",
+ " Average Recall (AR) @[ IoU=0.50:0.95 | area=medium | maxDets=100 ] = 0.268\n",
+ " Average Recall (AR) @[ IoU=0.50:0.95 | area= large | maxDets=100 ] = 0.524\n",
+ "4138/4138 [==============================] - 606s 146ms/step - loss: 0.4535 - box_loss: 0.4175 - class_loss: 0.0359 - val_loss: 1.2286 - val_box_loss: 1.1620 - val_class_loss: 0.0666 - val_AP: 0.3579 - val_AP50: 0.5107 - val_AP75: 0.3893 - val_APs: 0.0572 - val_APm: 0.1718 - val_APl: 0.4205 - val_ARmax1: 0.3497 - val_ARmax10: 0.4589 - val_ARmax100: 0.4621 - val_ARs: 0.0998 - val_ARm: 0.2678 - val_ARl: 0.5235\n",
+ "Epoch 110/120\n",
+ "1238/1238 [==============================] - 111s 89ms/step\n",
+ "creating index...\n",
+ "index created!\n",
+ "creating index...\n",
+ "index created!\n",
+ "Running per image evaluation...\n",
+ "Evaluate annotation type *bbox*\n",
+ "DONE (t=4.77s).\n",
+ "Accumulating evaluation results...\n",
+ "DONE (t=1.02s).\n",
+ " Average Precision (AP) @[ IoU=0.50:0.95 | area= all | maxDets=100 ] = 0.361\n",
+ " Average Precision (AP) @[ IoU=0.50 | area= all | maxDets=100 ] = 0.514\n",
+ " Average Precision (AP) @[ IoU=0.75 | area= all | maxDets=100 ] = 0.393\n",
+ " Average Precision (AP) @[ IoU=0.50:0.95 | area= small | maxDets=100 ] = 0.066\n",
+ " Average Precision (AP) @[ IoU=0.50:0.95 | area=medium | maxDets=100 ] = 0.173\n",
+ " Average Precision (AP) @[ IoU=0.50:0.95 | area= large | maxDets=100 ] = 0.425\n",
+ " Average Recall (AR) @[ IoU=0.50:0.95 | area= all | maxDets= 1 ] = 0.355\n",
+ " Average Recall (AR) @[ IoU=0.50:0.95 | area= all | maxDets= 10 ] = 0.467\n",
+ " Average Recall (AR) @[ IoU=0.50:0.95 | area= all | maxDets=100 ] = 0.470\n",
+ " Average Recall (AR) @[ IoU=0.50:0.95 | area= small | maxDets=100 ] = 0.102\n",
+ " Average Recall (AR) @[ IoU=0.50:0.95 | area=medium | maxDets=100 ] = 0.267\n",
+ " Average Recall (AR) @[ IoU=0.50:0.95 | area= large | maxDets=100 ] = 0.534\n",
+ "4138/4138 [==============================] - 605s 146ms/step - loss: 0.4477 - box_loss: 0.4122 - class_loss: 0.0356 - val_loss: 1.2308 - val_box_loss: 1.1642 - val_class_loss: 0.0666 - val_AP: 0.3609 - val_AP50: 0.5137 - val_AP75: 0.3932 - val_APs: 0.0662 - val_APm: 0.1730 - val_APl: 0.4254 - val_ARmax1: 0.3551 - val_ARmax10: 0.4668 - val_ARmax100: 0.4700 - val_ARs: 0.1015 - val_ARm: 0.2666 - val_ARl: 0.5338\n",
+ "Epoch 111/120\n",
+ "1238/1238 [==============================] - 111s 89ms/step\n",
+ "creating index...\n",
+ "index created!\n",
+ "creating index...\n",
+ "index created!\n",
+ "Running per image evaluation...\n",
+ "Evaluate annotation type *bbox*\n",
+ "DONE (t=4.70s).\n",
+ "Accumulating evaluation results...\n",
+ "DONE (t=1.00s).\n",
+ " Average Precision (AP) @[ IoU=0.50:0.95 | area= all | maxDets=100 ] = 0.356\n",
+ " Average Precision (AP) @[ IoU=0.50 | area= all | maxDets=100 ] = 0.507\n",
+ " Average Precision (AP) @[ IoU=0.75 | area= all | maxDets=100 ] = 0.387\n",
+ " Average Precision (AP) @[ IoU=0.50:0.95 | area= small | maxDets=100 ] = 0.060\n",
+ " Average Precision (AP) @[ IoU=0.50:0.95 | area=medium | maxDets=100 ] = 0.171\n",
+ " Average Precision (AP) @[ IoU=0.50:0.95 | area= large | maxDets=100 ] = 0.419\n",
+ " Average Recall (AR) @[ IoU=0.50:0.95 | area= all | maxDets= 1 ] = 0.350\n",
+ " Average Recall (AR) @[ IoU=0.50:0.95 | area= all | maxDets= 10 ] = 0.456\n",
+ " Average Recall (AR) @[ IoU=0.50:0.95 | area= all | maxDets=100 ] = 0.460\n",
+ " Average Recall (AR) @[ IoU=0.50:0.95 | area= small | maxDets=100 ] = 0.099\n",
+ " Average Recall (AR) @[ IoU=0.50:0.95 | area=medium | maxDets=100 ] = 0.263\n",
+ " Average Recall (AR) @[ IoU=0.50:0.95 | area= large | maxDets=100 ] = 0.522\n",
+ "4138/4138 [==============================] - 605s 146ms/step - loss: 0.4427 - box_loss: 0.4074 - class_loss: 0.0352 - val_loss: 1.2370 - val_box_loss: 1.1696 - val_class_loss: 0.0674 - val_AP: 0.3563 - val_AP50: 0.5066 - val_AP75: 0.3871 - val_APs: 0.0597 - val_APm: 0.1711 - val_APl: 0.4189 - val_ARmax1: 0.3502 - val_ARmax10: 0.4564 - val_ARmax100: 0.4598 - val_ARs: 0.0988 - val_ARm: 0.2626 - val_ARl: 0.5216\n",
+ "Epoch 112/120\n",
+ "1238/1238 [==============================] - 111s 89ms/step\n",
+ "creating index...\n",
+ "index created!\n",
+ "creating index...\n",
+ "index created!\n",
+ "Running per image evaluation...\n",
+ "Evaluate annotation type *bbox*\n",
+ "DONE (t=4.81s).\n",
+ "Accumulating evaluation results...\n",
+ "DONE (t=1.02s).\n",
+ " Average Precision (AP) @[ IoU=0.50:0.95 | area= all | maxDets=100 ] = 0.362\n",
+ " Average Precision (AP) @[ IoU=0.50 | area= all | maxDets=100 ] = 0.516\n",
+ " Average Precision (AP) @[ IoU=0.75 | area= all | maxDets=100 ] = 0.396\n",
+ " Average Precision (AP) @[ IoU=0.50:0.95 | area= small | maxDets=100 ] = 0.065\n",
+ " Average Precision (AP) @[ IoU=0.50:0.95 | area=medium | maxDets=100 ] = 0.175\n",
+ " Average Precision (AP) @[ IoU=0.50:0.95 | area= large | maxDets=100 ] = 0.424\n",
+ " Average Recall (AR) @[ IoU=0.50:0.95 | area= all | maxDets= 1 ] = 0.356\n",
+ " Average Recall (AR) @[ IoU=0.50:0.95 | area= all | maxDets= 10 ] = 0.465\n",
+ " Average Recall (AR) @[ IoU=0.50:0.95 | area= all | maxDets=100 ] = 0.468\n",
+ " Average Recall (AR) @[ IoU=0.50:0.95 | area= small | maxDets=100 ] = 0.100\n",
+ " Average Recall (AR) @[ IoU=0.50:0.95 | area=medium | maxDets=100 ] = 0.268\n",
+ " Average Recall (AR) @[ IoU=0.50:0.95 | area= large | maxDets=100 ] = 0.531\n",
+ "4138/4138 [==============================] - 606s 146ms/step - loss: 0.4384 - box_loss: 0.4033 - class_loss: 0.0350 - val_loss: 1.2323 - val_box_loss: 1.1656 - val_class_loss: 0.0667 - val_AP: 0.3620 - val_AP50: 0.5164 - val_AP75: 0.3960 - val_APs: 0.0653 - val_APm: 0.1751 - val_APl: 0.4244 - val_ARmax1: 0.3562 - val_ARmax10: 0.4653 - val_ARmax100: 0.4684 - val_ARs: 0.0997 - val_ARm: 0.2679 - val_ARl: 0.5309\n",
+ "Epoch 113/120\n",
+ "1238/1238 [==============================] - 111s 89ms/step\n",
+ "creating index...\n",
+ "index created!\n",
+ "creating index...\n",
+ "index created!\n",
+ "Running per image evaluation...\n",
+ "Evaluate annotation type *bbox*\n",
+ "DONE (t=5.37s).\n",
+ "Accumulating evaluation results...\n",
+ "DONE (t=1.01s).\n",
+ " Average Precision (AP) @[ IoU=0.50:0.95 | area= all | maxDets=100 ] = 0.355\n",
+ " Average Precision (AP) @[ IoU=0.50 | area= all | maxDets=100 ] = 0.506\n",
+ " Average Precision (AP) @[ IoU=0.75 | area= all | maxDets=100 ] = 0.388\n",
+ " Average Precision (AP) @[ IoU=0.50:0.95 | area= small | maxDets=100 ] = 0.061\n",
+ " Average Precision (AP) @[ IoU=0.50:0.95 | area=medium | maxDets=100 ] = 0.172\n",
+ " Average Precision (AP) @[ IoU=0.50:0.95 | area= large | maxDets=100 ] = 0.418\n",
+ " Average Recall (AR) @[ IoU=0.50:0.95 | area= all | maxDets= 1 ] = 0.354\n",
+ " Average Recall (AR) @[ IoU=0.50:0.95 | area= all | maxDets= 10 ] = 0.460\n",
+ " Average Recall (AR) @[ IoU=0.50:0.95 | area= all | maxDets=100 ] = 0.463\n",
+ " Average Recall (AR) @[ IoU=0.50:0.95 | area= small | maxDets=100 ] = 0.095\n",
+ " Average Recall (AR) @[ IoU=0.50:0.95 | area=medium | maxDets=100 ] = 0.261\n",
+ " Average Recall (AR) @[ IoU=0.50:0.95 | area= large | maxDets=100 ] = 0.526\n",
+ "4138/4138 [==============================] - 607s 146ms/step - loss: 0.4370 - box_loss: 0.4022 - class_loss: 0.0349 - val_loss: 1.2397 - val_box_loss: 1.1725 - val_class_loss: 0.0672 - val_AP: 0.3552 - val_AP50: 0.5063 - val_AP75: 0.3877 - val_APs: 0.0606 - val_APm: 0.1716 - val_APl: 0.4175 - val_ARmax1: 0.3535 - val_ARmax10: 0.4600 - val_ARmax100: 0.4630 - val_ARs: 0.0955 - val_ARm: 0.2609 - val_ARl: 0.5264\n",
+ "Epoch 114/120\n",
+ "1238/1238 [==============================] - 111s 89ms/step\n",
+ "creating index...\n",
+ "index created!\n",
+ "creating index...\n",
+ "index created!\n",
+ "Running per image evaluation...\n",
+ "Evaluate annotation type *bbox*\n",
+ "DONE (t=4.81s).\n",
+ "Accumulating evaluation results...\n",
+ "DONE (t=1.02s).\n",
+ " Average Precision (AP) @[ IoU=0.50:0.95 | area= all | maxDets=100 ] = 0.360\n",
+ " Average Precision (AP) @[ IoU=0.50 | area= all | maxDets=100 ] = 0.514\n",
+ " Average Precision (AP) @[ IoU=0.75 | area= all | maxDets=100 ] = 0.395\n",
+ " Average Precision (AP) @[ IoU=0.50:0.95 | area= small | maxDets=100 ] = 0.063\n",
+ " Average Precision (AP) @[ IoU=0.50:0.95 | area=medium | maxDets=100 ] = 0.179\n",
+ " Average Precision (AP) @[ IoU=0.50:0.95 | area= large | maxDets=100 ] = 0.423\n",
+ " Average Recall (AR) @[ IoU=0.50:0.95 | area= all | maxDets= 1 ] = 0.353\n",
+ " Average Recall (AR) @[ IoU=0.50:0.95 | area= all | maxDets= 10 ] = 0.463\n",
+ " Average Recall (AR) @[ IoU=0.50:0.95 | area= all | maxDets=100 ] = 0.466\n",
+ " Average Recall (AR) @[ IoU=0.50:0.95 | area= small | maxDets=100 ] = 0.101\n",
+ " Average Recall (AR) @[ IoU=0.50:0.95 | area=medium | maxDets=100 ] = 0.269\n",
+ " Average Recall (AR) @[ IoU=0.50:0.95 | area= large | maxDets=100 ] = 0.530\n",
+ "4138/4138 [==============================] - 605s 146ms/step - loss: 0.4473 - box_loss: 0.4118 - class_loss: 0.0355 - val_loss: 1.2302 - val_box_loss: 1.1637 - val_class_loss: 0.0665 - val_AP: 0.3603 - val_AP50: 0.5143 - val_AP75: 0.3950 - val_APs: 0.0626 - val_APm: 0.1788 - val_APl: 0.4235 - val_ARmax1: 0.3533 - val_ARmax10: 0.4631 - val_ARmax100: 0.4662 - val_ARs: 0.1013 - val_ARm: 0.2686 - val_ARl: 0.5296\n",
+ "Epoch 115/120\n",
+ "1238/1238 [==============================] - 110s 89ms/step\n",
+ "creating index...\n",
+ "index created!\n",
+ "creating index...\n",
+ "index created!\n",
+ "Running per image evaluation...\n",
+ "Evaluate annotation type *bbox*\n",
+ "DONE (t=5.42s).\n",
+ "Accumulating evaluation results...\n",
+ "DONE (t=1.01s).\n",
+ " Average Precision (AP) @[ IoU=0.50:0.95 | area= all | maxDets=100 ] = 0.355\n",
+ " Average Precision (AP) @[ IoU=0.50 | area= all | maxDets=100 ] = 0.507\n",
+ " Average Precision (AP) @[ IoU=0.75 | area= all | maxDets=100 ] = 0.387\n",
+ " Average Precision (AP) @[ IoU=0.50:0.95 | area= small | maxDets=100 ] = 0.061\n",
+ " Average Precision (AP) @[ IoU=0.50:0.95 | area=medium | maxDets=100 ] = 0.176\n",
+ " Average Precision (AP) @[ IoU=0.50:0.95 | area= large | maxDets=100 ] = 0.418\n",
+ " Average Recall (AR) @[ IoU=0.50:0.95 | area= all | maxDets= 1 ] = 0.351\n",
+ " Average Recall (AR) @[ IoU=0.50:0.95 | area= all | maxDets= 10 ] = 0.459\n",
+ " Average Recall (AR) @[ IoU=0.50:0.95 | area= all | maxDets=100 ] = 0.462\n",
+ " Average Recall (AR) @[ IoU=0.50:0.95 | area= small | maxDets=100 ] = 0.100\n",
+ " Average Recall (AR) @[ IoU=0.50:0.95 | area=medium | maxDets=100 ] = 0.266\n",
+ " Average Recall (AR) @[ IoU=0.50:0.95 | area= large | maxDets=100 ] = 0.525\n",
+ "4138/4138 [==============================] - 606s 146ms/step - loss: 0.4296 - box_loss: 0.3952 - class_loss: 0.0344 - val_loss: 1.2352 - val_box_loss: 1.1683 - val_class_loss: 0.0669 - val_AP: 0.3548 - val_AP50: 0.5075 - val_AP75: 0.3867 - val_APs: 0.0612 - val_APm: 0.1756 - val_APl: 0.4177 - val_ARmax1: 0.3509 - val_ARmax10: 0.4589 - val_ARmax100: 0.4618 - val_ARs: 0.0998 - val_ARm: 0.2657 - val_ARl: 0.5250\n",
+ "Epoch 116/120\n",
+ "1238/1238 [==============================] - 111s 89ms/step\n",
+ "creating index...\n",
+ "index created!\n",
+ "creating index...\n",
+ "index created!\n",
+ "Running per image evaluation...\n",
+ "Evaluate annotation type *bbox*\n",
+ "DONE (t=4.84s).\n",
+ "Accumulating evaluation results...\n",
+ "DONE (t=1.02s).\n",
+ " Average Precision (AP) @[ IoU=0.50:0.95 | area= all | maxDets=100 ] = 0.363\n",
+ " Average Precision (AP) @[ IoU=0.50 | area= all | maxDets=100 ] = 0.515\n",
+ " Average Precision (AP) @[ IoU=0.75 | area= all | maxDets=100 ] = 0.397\n",
+ " Average Precision (AP) @[ IoU=0.50:0.95 | area= small | maxDets=100 ] = 0.062\n",
+ " Average Precision (AP) @[ IoU=0.50:0.95 | area=medium | maxDets=100 ] = 0.183\n",
+ " Average Precision (AP) @[ IoU=0.50:0.95 | area= large | maxDets=100 ] = 0.424\n",
+ " Average Recall (AR) @[ IoU=0.50:0.95 | area= all | maxDets= 1 ] = 0.357\n",
+ " Average Recall (AR) @[ IoU=0.50:0.95 | area= all | maxDets= 10 ] = 0.467\n",
+ " Average Recall (AR) @[ IoU=0.50:0.95 | area= all | maxDets=100 ] = 0.470\n",
+ " Average Recall (AR) @[ IoU=0.50:0.95 | area= small | maxDets=100 ] = 0.104\n",
+ " Average Recall (AR) @[ IoU=0.50:0.95 | area=medium | maxDets=100 ] = 0.276\n",
+ " Average Recall (AR) @[ IoU=0.50:0.95 | area= large | maxDets=100 ] = 0.529\n",
+ "4138/4138 [==============================] - 606s 146ms/step - loss: 0.4361 - box_loss: 0.4013 - class_loss: 0.0348 - val_loss: 1.2340 - val_box_loss: 1.1675 - val_class_loss: 0.0665 - val_AP: 0.3634 - val_AP50: 0.5154 - val_AP75: 0.3966 - val_APs: 0.0619 - val_APm: 0.1833 - val_APl: 0.4239 - val_ARmax1: 0.3567 - val_ARmax10: 0.4667 - val_ARmax100: 0.4700 - val_ARs: 0.1042 - val_ARm: 0.2763 - val_ARl: 0.5293\n",
+ "Epoch 117/120\n",
+ "1238/1238 [==============================] - 111s 89ms/step\n",
+ "creating index...\n",
+ "index created!\n",
+ "creating index...\n",
+ "index created!\n",
+ "Running per image evaluation...\n",
+ "Evaluate annotation type *bbox*\n",
+ "DONE (t=5.39s).\n",
+ "Accumulating evaluation results...\n",
+ "DONE (t=1.00s).\n",
+ " Average Precision (AP) @[ IoU=0.50:0.95 | area= all | maxDets=100 ] = 0.357\n",
+ " Average Precision (AP) @[ IoU=0.50 | area= all | maxDets=100 ] = 0.505\n",
+ " Average Precision (AP) @[ IoU=0.75 | area= all | maxDets=100 ] = 0.391\n",
+ " Average Precision (AP) @[ IoU=0.50:0.95 | area= small | maxDets=100 ] = 0.056\n",
+ " Average Precision (AP) @[ IoU=0.50:0.95 | area=medium | maxDets=100 ] = 0.174\n",
+ " Average Precision (AP) @[ IoU=0.50:0.95 | area= large | maxDets=100 ] = 0.421\n",
+ " Average Recall (AR) @[ IoU=0.50:0.95 | area= all | maxDets= 1 ] = 0.352\n",
+ " Average Recall (AR) @[ IoU=0.50:0.95 | area= all | maxDets= 10 ] = 0.458\n",
+ " Average Recall (AR) @[ IoU=0.50:0.95 | area= all | maxDets=100 ] = 0.461\n",
+ " Average Recall (AR) @[ IoU=0.50:0.95 | area= small | maxDets=100 ] = 0.096\n",
+ " Average Recall (AR) @[ IoU=0.50:0.95 | area=medium | maxDets=100 ] = 0.262\n",
+ " Average Recall (AR) @[ IoU=0.50:0.95 | area= large | maxDets=100 ] = 0.526\n",
+ "4138/4138 [==============================] - 606s 146ms/step - loss: 0.4258 - box_loss: 0.3915 - class_loss: 0.0342 - val_loss: 1.2340 - val_box_loss: 1.1670 - val_class_loss: 0.0670 - val_AP: 0.3567 - val_AP50: 0.5053 - val_AP75: 0.3907 - val_APs: 0.0564 - val_APm: 0.1737 - val_APl: 0.4208 - val_ARmax1: 0.3516 - val_ARmax10: 0.4580 - val_ARmax100: 0.4609 - val_ARs: 0.0958 - val_ARm: 0.2620 - val_ARl: 0.5263\n",
+ "Epoch 118/120\n",
+ "1238/1238 [==============================] - 111s 89ms/step\n",
+ "creating index...\n",
+ "index created!\n",
+ "creating index...\n",
+ "index created!\n",
+ "Running per image evaluation...\n",
+ "Evaluate annotation type *bbox*\n",
+ "DONE (t=4.80s).\n",
+ "Accumulating evaluation results...\n",
+ "DONE (t=1.01s).\n",
+ " Average Precision (AP) @[ IoU=0.50:0.95 | area= all | maxDets=100 ] = 0.360\n",
+ " Average Precision (AP) @[ IoU=0.50 | area= all | maxDets=100 ] = 0.513\n",
+ " Average Precision (AP) @[ IoU=0.75 | area= all | maxDets=100 ] = 0.394\n",
+ " Average Precision (AP) @[ IoU=0.50:0.95 | area= small | maxDets=100 ] = 0.071\n",
+ " Average Precision (AP) @[ IoU=0.50:0.95 | area=medium | maxDets=100 ] = 0.176\n",
+ " Average Precision (AP) @[ IoU=0.50:0.95 | area= large | maxDets=100 ] = 0.424\n",
+ " Average Recall (AR) @[ IoU=0.50:0.95 | area= all | maxDets= 1 ] = 0.355\n",
+ " Average Recall (AR) @[ IoU=0.50:0.95 | area= all | maxDets= 10 ] = 0.463\n",
+ " Average Recall (AR) @[ IoU=0.50:0.95 | area= all | maxDets=100 ] = 0.465\n",
+ " Average Recall (AR) @[ IoU=0.50:0.95 | area= small | maxDets=100 ] = 0.106\n",
+ " Average Recall (AR) @[ IoU=0.50:0.95 | area=medium | maxDets=100 ] = 0.268\n",
+ " Average Recall (AR) @[ IoU=0.50:0.95 | area= large | maxDets=100 ] = 0.528\n",
+ "4138/4138 [==============================] - 607s 146ms/step - loss: 0.4199 - box_loss: 0.3861 - class_loss: 0.0338 - val_loss: 1.2327 - val_box_loss: 1.1661 - val_class_loss: 0.0667 - val_AP: 0.3604 - val_AP50: 0.5131 - val_AP75: 0.3940 - val_APs: 0.0706 - val_APm: 0.1765 - val_APl: 0.4237 - val_ARmax1: 0.3550 - val_ARmax10: 0.4625 - val_ARmax100: 0.4654 - val_ARs: 0.1059 - val_ARm: 0.2678 - val_ARl: 0.5282\n",
+ "Epoch 119/120\n",
+ "1238/1238 [==============================] - 111s 89ms/step\n",
+ "creating index...\n",
+ "index created!\n",
+ "creating index...\n",
+ "index created!\n",
+ "Running per image evaluation...\n",
+ "Evaluate annotation type *bbox*\n",
+ "DONE (t=5.49s).\n",
+ "Accumulating evaluation results...\n",
+ "DONE (t=1.03s).\n",
+ " Average Precision (AP) @[ IoU=0.50:0.95 | area= all | maxDets=100 ] = 0.359\n",
+ " Average Precision (AP) @[ IoU=0.50 | area= all | maxDets=100 ] = 0.513\n",
+ " Average Precision (AP) @[ IoU=0.75 | area= all | maxDets=100 ] = 0.389\n",
+ " Average Precision (AP) @[ IoU=0.50:0.95 | area= small | maxDets=100 ] = 0.066\n",
+ " Average Precision (AP) @[ IoU=0.50:0.95 | area=medium | maxDets=100 ] = 0.179\n",
+ " Average Precision (AP) @[ IoU=0.50:0.95 | area= large | maxDets=100 ] = 0.421\n",
+ " Average Recall (AR) @[ IoU=0.50:0.95 | area= all | maxDets= 1 ] = 0.356\n",
+ " Average Recall (AR) @[ IoU=0.50:0.95 | area= all | maxDets= 10 ] = 0.464\n",
+ " Average Recall (AR) @[ IoU=0.50:0.95 | area= all | maxDets=100 ] = 0.467\n",
+ " Average Recall (AR) @[ IoU=0.50:0.95 | area= small | maxDets=100 ] = 0.101\n",
+ " Average Recall (AR) @[ IoU=0.50:0.95 | area=medium | maxDets=100 ] = 0.272\n",
+ " Average Recall (AR) @[ IoU=0.50:0.95 | area= large | maxDets=100 ] = 0.528\n",
+ "4138/4138 [==============================] - 607s 147ms/step - loss: 0.4292 - box_loss: 0.3946 - class_loss: 0.0345 - val_loss: 1.2323 - val_box_loss: 1.1659 - val_class_loss: 0.0665 - val_AP: 0.3593 - val_AP50: 0.5133 - val_AP75: 0.3895 - val_APs: 0.0659 - val_APm: 0.1789 - val_APl: 0.4210 - val_ARmax1: 0.3556 - val_ARmax10: 0.4644 - val_ARmax100: 0.4674 - val_ARs: 0.1006 - val_ARm: 0.2718 - val_ARl: 0.5280\n",
+ "Epoch 120/120\n",
+ "1238/1238 [==============================] - 111s 89ms/step\n",
+ "creating index...\n",
+ "index created!\n",
+ "creating index...\n",
+ "index created!\n",
+ "Running per image evaluation...\n",
+ "Evaluate annotation type *bbox*\n",
+ "DONE (t=4.83s).\n",
+ "Accumulating evaluation results...\n",
+ "DONE (t=1.01s).\n",
+ " Average Precision (AP) @[ IoU=0.50:0.95 | area= all | maxDets=100 ] = 0.359\n",
+ " Average Precision (AP) @[ IoU=0.50 | area= all | maxDets=100 ] = 0.513\n",
+ " Average Precision (AP) @[ IoU=0.75 | area= all | maxDets=100 ] = 0.391\n",
+ " Average Precision (AP) @[ IoU=0.50:0.95 | area= small | maxDets=100 ] = 0.066\n",
+ " Average Precision (AP) @[ IoU=0.50:0.95 | area=medium | maxDets=100 ] = 0.176\n",
+ " Average Precision (AP) @[ IoU=0.50:0.95 | area= large | maxDets=100 ] = 0.422\n",
+ " Average Recall (AR) @[ IoU=0.50:0.95 | area= all | maxDets= 1 ] = 0.355\n",
+ " Average Recall (AR) @[ IoU=0.50:0.95 | area= all | maxDets= 10 ] = 0.464\n",
+ " Average Recall (AR) @[ IoU=0.50:0.95 | area= all | maxDets=100 ] = 0.467\n",
+ " Average Recall (AR) @[ IoU=0.50:0.95 | area= small | maxDets=100 ] = 0.102\n",
+ " Average Recall (AR) @[ IoU=0.50:0.95 | area=medium | maxDets=100 ] = 0.266\n",
+ " Average Recall (AR) @[ IoU=0.50:0.95 | area= large | maxDets=100 ] = 0.530\n",
+ "4138/4138 [==============================] - 606s 146ms/step - loss: 0.4178 - box_loss: 0.3841 - class_loss: 0.0337 - val_loss: 1.2377 - val_box_loss: 1.1710 - val_class_loss: 0.0668 - val_AP: 0.3588 - val_AP50: 0.5127 - val_AP75: 0.3908 - val_APs: 0.0661 - val_APm: 0.1760 - val_APl: 0.4215 - val_ARmax1: 0.3549 - val_ARmax10: 0.4638 - val_ARmax100: 0.4671 - val_ARs: 0.1020 - val_ARm: 0.2658 - val_ARl: 0.5299\n"
+ ]
+ }
+ ]
+ },
+ {
+ "cell_type": "code",
+ "source": [
+ "from keras_cv import bounding_box, visualization\n",
+ "\n",
+ "\n",
+ "def visualize_detections(model, dataset, bounding_box_format, rows, cols):\n",
+ " images, y_true = next(iter(dataset.take(1)))\n",
+ " y_pred = model.predict(images)\n",
+ " y_pred = bounding_box.to_ragged(y_pred)\n",
+ " visualization.plot_bounding_box_gallery(\n",
+ " images,\n",
+ " value_range=(0, 255),\n",
+ " bounding_box_format=bounding_box_format,\n",
+ " y_true=y_true,\n",
+ " y_pred=y_pred,\n",
+ " scale=4,\n",
+ " rows=rows,\n",
+ " cols=cols,\n",
+ " show=True,\n",
+ " font_scale=0.7,\n",
+ " class_mapping=class_mapping,\n",
+ " )"
+ ],
+ "metadata": {
+ "id": "Zt_Wg_PpObgK"
+ },
+ "execution_count": null,
+ "outputs": []
+ },
+ {
+ "cell_type": "code",
+ "source": [
+ "class_ids = [\n",
+ " \"Aeroplane\",\n",
+ " \"Bicycle\",\n",
+ " \"Bird\",\n",
+ " \"Boat\",\n",
+ " \"Bottle\",\n",
+ " \"Bus\",\n",
+ " \"Car\",\n",
+ " \"Cat\",\n",
+ " \"Chair\",\n",
+ " \"Cow\",\n",
+ " \"Dining Table\",\n",
+ " \"Dog\",\n",
+ " \"Horse\",\n",
+ " \"Motorbike\",\n",
+ " \"Person\",\n",
+ " \"Potted Plant\",\n",
+ " \"Sheep\",\n",
+ " \"Sofa\",\n",
+ " \"Train\",\n",
+ " \"Tvmonitor\",\n",
+ " \"Total\",\n",
+ "]\n",
+ "class_mapping = dict(zip(range(len(class_ids)), class_ids))"
+ ],
+ "metadata": {
+ "id": "MOGlE8o9Obbc"
+ },
+ "execution_count": null,
+ "outputs": []
+ },
+ {
+ "cell_type": "code",
+ "source": [
+ "model.prediction_decoder = keras_cv.layers.MultiClassNonMaxSuppression(\n",
+ " bounding_box_format=\"xywh\",\n",
+ " from_logits=False,\n",
+ " confidence_threshold=0.3,\n",
+ " iou_threshold=0.5,\n",
+ ")\n",
+ "model.make_predict_function(force=True)\n",
+ "visualize_detections(model, eval_ds.shuffle(10), \"xywh\", rows=2, cols=2)\n",
+ "old_model = model"
+ ],
+ "metadata": {
+ "id": "qMTWxQQ_Op1Q",
+ "colab": {
+ "base_uri": "https://localhost:8080/",
+ "height": 373
+ },
+ "outputId": "9d21c7f8-a023-43ab-f775-41011eff7753"
+ },
+ "execution_count": null,
+ "outputs": [
+ {
+ "output_type": "error",
+ "ename": "IndexError",
+ "evalue": "ignored",
+ "traceback": [
+ "\u001b[0;31m---------------------------------------------------------------------------\u001b[0m",
+ "\u001b[0;31mIndexError\u001b[0m Traceback (most recent call last)",
+ "\u001b[0;32m\u001b[0m in \u001b[0;36m\u001b[0;34m()\u001b[0m\n\u001b[1;32m 6\u001b[0m )\n\u001b[1;32m 7\u001b[0m \u001b[0mmodel\u001b[0m\u001b[0;34m.\u001b[0m\u001b[0mmake_predict_function\u001b[0m\u001b[0;34m(\u001b[0m\u001b[0mforce\u001b[0m\u001b[0;34m=\u001b[0m\u001b[0;32mTrue\u001b[0m\u001b[0;34m)\u001b[0m\u001b[0;34m\u001b[0m\u001b[0;34m\u001b[0m\u001b[0m\n\u001b[0;32m----> 8\u001b[0;31m \u001b[0mvisualize_detections\u001b[0m\u001b[0;34m(\u001b[0m\u001b[0mmodel\u001b[0m\u001b[0;34m,\u001b[0m \u001b[0meval_ds\u001b[0m\u001b[0;34m.\u001b[0m\u001b[0mshuffle\u001b[0m\u001b[0;34m(\u001b[0m\u001b[0;36m10\u001b[0m\u001b[0;34m)\u001b[0m\u001b[0;34m,\u001b[0m \u001b[0;34m\"xywh\"\u001b[0m\u001b[0;34m,\u001b[0m \u001b[0mrows\u001b[0m\u001b[0;34m=\u001b[0m\u001b[0;36m2\u001b[0m\u001b[0;34m,\u001b[0m \u001b[0mcols\u001b[0m\u001b[0;34m=\u001b[0m\u001b[0;36m2\u001b[0m\u001b[0;34m)\u001b[0m\u001b[0;34m\u001b[0m\u001b[0;34m\u001b[0m\u001b[0m\n\u001b[0m\u001b[1;32m 9\u001b[0m \u001b[0mold_model\u001b[0m \u001b[0;34m=\u001b[0m \u001b[0mmodel\u001b[0m\u001b[0;34m\u001b[0m\u001b[0;34m\u001b[0m\u001b[0m\n",
+ "\u001b[0;32m\u001b[0m in \u001b[0;36mvisualize_detections\u001b[0;34m(model, dataset, bounding_box_format, rows, cols)\u001b[0m\n\u001b[1;32m 2\u001b[0m \u001b[0;32mdef\u001b[0m \u001b[0mvisualize_detections\u001b[0m\u001b[0;34m(\u001b[0m\u001b[0mmodel\u001b[0m\u001b[0;34m,\u001b[0m \u001b[0mdataset\u001b[0m\u001b[0;34m,\u001b[0m \u001b[0mbounding_box_format\u001b[0m\u001b[0;34m,\u001b[0m \u001b[0mrows\u001b[0m\u001b[0;34m,\u001b[0m \u001b[0mcols\u001b[0m\u001b[0;34m)\u001b[0m\u001b[0;34m:\u001b[0m\u001b[0;34m\u001b[0m\u001b[0;34m\u001b[0m\u001b[0m\n\u001b[1;32m 3\u001b[0m \u001b[0mimages\u001b[0m\u001b[0;34m,\u001b[0m \u001b[0my_true\u001b[0m \u001b[0;34m=\u001b[0m \u001b[0mnext\u001b[0m\u001b[0;34m(\u001b[0m\u001b[0miter\u001b[0m\u001b[0;34m(\u001b[0m\u001b[0mdataset\u001b[0m\u001b[0;34m.\u001b[0m\u001b[0mtake\u001b[0m\u001b[0;34m(\u001b[0m\u001b[0;36m1\u001b[0m\u001b[0;34m)\u001b[0m\u001b[0;34m)\u001b[0m\u001b[0;34m)\u001b[0m\u001b[0;34m\u001b[0m\u001b[0;34m\u001b[0m\u001b[0m\n\u001b[0;32m----> 4\u001b[0;31m \u001b[0my_pred\u001b[0m \u001b[0;34m=\u001b[0m \u001b[0mmodel\u001b[0m\u001b[0;34m.\u001b[0m\u001b[0mpredict\u001b[0m\u001b[0;34m(\u001b[0m\u001b[0mimages\u001b[0m\u001b[0;34m)\u001b[0m\u001b[0;34m\u001b[0m\u001b[0;34m\u001b[0m\u001b[0m\n\u001b[0m\u001b[1;32m 5\u001b[0m \u001b[0my_pred\u001b[0m \u001b[0;34m=\u001b[0m \u001b[0mbounding_box\u001b[0m\u001b[0;34m.\u001b[0m\u001b[0mto_ragged\u001b[0m\u001b[0;34m(\u001b[0m\u001b[0my_pred\u001b[0m\u001b[0;34m)\u001b[0m\u001b[0;34m\u001b[0m\u001b[0;34m\u001b[0m\u001b[0m\n\u001b[1;32m 6\u001b[0m visualization.plot_bounding_box_gallery(\n",
+ "\u001b[0;32m/usr/local/lib/python3.10/dist-packages/keras/utils/traceback_utils.py\u001b[0m in \u001b[0;36merror_handler\u001b[0;34m(*args, **kwargs)\u001b[0m\n\u001b[1;32m 68\u001b[0m \u001b[0;31m# To get the full stack trace, call:\u001b[0m\u001b[0;34m\u001b[0m\u001b[0;34m\u001b[0m\u001b[0m\n\u001b[1;32m 69\u001b[0m \u001b[0;31m# `tf.debugging.disable_traceback_filtering()`\u001b[0m\u001b[0;34m\u001b[0m\u001b[0;34m\u001b[0m\u001b[0m\n\u001b[0;32m---> 70\u001b[0;31m \u001b[0;32mraise\u001b[0m \u001b[0me\u001b[0m\u001b[0;34m.\u001b[0m\u001b[0mwith_traceback\u001b[0m\u001b[0;34m(\u001b[0m\u001b[0mfiltered_tb\u001b[0m\u001b[0;34m)\u001b[0m \u001b[0;32mfrom\u001b[0m \u001b[0;32mNone\u001b[0m\u001b[0;34m\u001b[0m\u001b[0;34m\u001b[0m\u001b[0m\n\u001b[0m\u001b[1;32m 71\u001b[0m \u001b[0;32mfinally\u001b[0m\u001b[0;34m:\u001b[0m\u001b[0;34m\u001b[0m\u001b[0;34m\u001b[0m\u001b[0m\n\u001b[1;32m 72\u001b[0m \u001b[0;32mdel\u001b[0m \u001b[0mfiltered_tb\u001b[0m\u001b[0;34m\u001b[0m\u001b[0;34m\u001b[0m\u001b[0m\n",
+ "\u001b[0;32m/usr/local/lib/python3.10/dist-packages/tensorflow/python/framework/tensor_shape.py\u001b[0m in \u001b[0;36m__getitem__\u001b[0;34m(self, key)\u001b[0m\n\u001b[1;32m 955\u001b[0m \u001b[0;32melse\u001b[0m\u001b[0;34m:\u001b[0m\u001b[0;34m\u001b[0m\u001b[0;34m\u001b[0m\u001b[0m\n\u001b[1;32m 956\u001b[0m \u001b[0;32mif\u001b[0m \u001b[0mself\u001b[0m\u001b[0;34m.\u001b[0m\u001b[0m_v2_behavior\u001b[0m\u001b[0;34m:\u001b[0m\u001b[0;34m\u001b[0m\u001b[0;34m\u001b[0m\u001b[0m\n\u001b[0;32m--> 957\u001b[0;31m \u001b[0;32mreturn\u001b[0m \u001b[0mself\u001b[0m\u001b[0;34m.\u001b[0m\u001b[0m_dims\u001b[0m\u001b[0;34m[\u001b[0m\u001b[0mkey\u001b[0m\u001b[0;34m]\u001b[0m\u001b[0;34m\u001b[0m\u001b[0;34m\u001b[0m\u001b[0m\n\u001b[0m\u001b[1;32m 958\u001b[0m \u001b[0;32melse\u001b[0m\u001b[0;34m:\u001b[0m\u001b[0;34m\u001b[0m\u001b[0;34m\u001b[0m\u001b[0m\n\u001b[1;32m 959\u001b[0m \u001b[0;32mreturn\u001b[0m \u001b[0mself\u001b[0m\u001b[0;34m.\u001b[0m\u001b[0mdims\u001b[0m\u001b[0;34m[\u001b[0m\u001b[0mkey\u001b[0m\u001b[0;34m]\u001b[0m\u001b[0;34m\u001b[0m\u001b[0;34m\u001b[0m\u001b[0m\n",
+ "\u001b[0;31mIndexError\u001b[0m: tuple index out of range"
+ ]
+ }
+ ]
+ }
+ ]
+}
\ No newline at end of file
diff --git a/keras_cv/tools/training_scipts/training_deeplab_v3_plus.ipynb b/keras_cv/tools/training_scipts/training_deeplab_v3_plus.ipynb
new file mode 100644
index 0000000000..e7ff38752a
--- /dev/null
+++ b/keras_cv/tools/training_scipts/training_deeplab_v3_plus.ipynb
@@ -0,0 +1,569 @@
+{
+ "cells": [
+ {
+ "cell_type": "markdown",
+ "metadata": {
+ "id": "0YC2vlsGs5tg"
+ },
+ "source": [
+ "# Semantic Segmentation with KerasCV\n",
+ "\n",
+ "**Author:** [Divyashree Sreepathihalli](https://github.com/divyashreepathihalli), [Ian Stenbit](https://github.com/ianstenbit) \n",
+ "**Date created:** 2023/08/22 \n",
+ "**Last modified:** 2023/08/24 \n",
+ "**Description:** Train and use DeepLabv3+ segmentation model with KerasCV."
+ ]
+ },
+ {
+ "cell_type": "markdown",
+ "metadata": {
+ "id": "zEUpBnaGs5th"
+ },
+ "source": [
+ "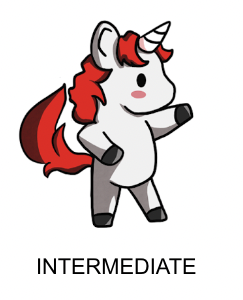\n",
+ "\n",
+ "## Background\n",
+ "Semantic segmentation is a type of computer vision task that involves assigning a\n",
+ "class label such as person, bike, or background to each individual pixel of an\n",
+ "image, effectively dividing the image into regions that correspond to different\n",
+ "fobject classes or categories.\n",
+ "\n",
+ "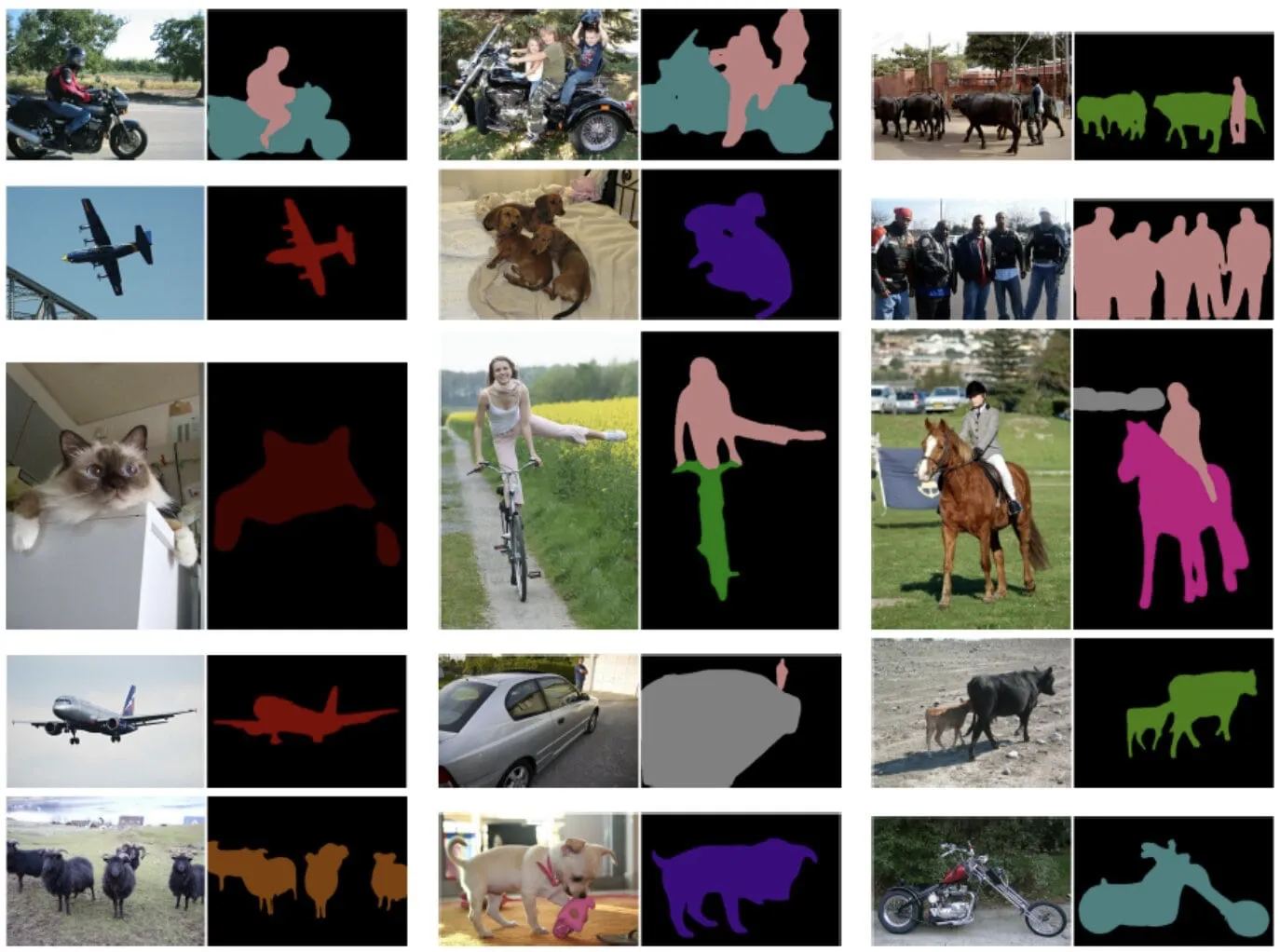\n",
+ "\n",
+ "\n",
+ "\n",
+ "KerasCV offers the DeepLabv3+ model developed by Google for semantic\n",
+ "segmentation. This guide demonstrates how to finetune and use DeepLabv3+ model for\n",
+ "image semantic segmentaion with KerasCV. Its architecture that combines atrous convolutions,\n",
+ "contextual information aggregation, and powerful backbones to achieve accurate and\n",
+ "detailed semantic segmentation. The DeepLabv3+ model has been shown to achieve\n",
+ "state-of-the-art results on a variety of image segmentation benchmarks.\n",
+ "\n",
+ "### References\n",
+ "[Encoder-Decoder with Atrous Separable Convolution for Semantic Image\n",
+ "Segmentation](https://arxiv.org/abs/1802.02611) \n",
+ "[Rethinking Atrous Convolution for Semantic Image\n",
+ "Segmentation](https://arxiv.org/abs/1706.05587)"
+ ]
+ },
+ {
+ "cell_type": "markdown",
+ "metadata": {
+ "id": "vgm-Z4Rus5ti"
+ },
+ "source": [
+ "## Setup and Imports\n",
+ "\n",
+ "Let's install the dependencies and import the necessary modules.\n",
+ "\n",
+ "To run this tutorial, you will need to install the following packages:\n",
+ "\n",
+ "* `keras-cv`\n",
+ "* `keras-core`"
+ ]
+ },
+ {
+ "cell_type": "code",
+ "execution_count": null,
+ "metadata": {
+ "id": "89IDcffts5ti"
+ },
+ "outputs": [],
+ "source": [
+ "!pip install -q --upgrade keras-cv\n",
+ "!pip install -q --upgrade keras # Upgrade to Keras 3."
+ ]
+ },
+ {
+ "cell_type": "markdown",
+ "metadata": {
+ "id": "aT_RCAG3s5tj"
+ },
+ "source": [
+ "After installing `keras-core` and `keras-cv`, set the backend for `keras-core`.\n",
+ "This guide can be run with any backend (Tensorflow, JAX, PyTorch).\n",
+ "\n",
+ "```\n",
+ "import os\n",
+ "\n",
+ "os.environ[\"KERAS_BACKEND\"] = \"jax\"\n",
+ "```"
+ ]
+ },
+ {
+ "cell_type": "code",
+ "execution_count": null,
+ "metadata": {
+ "id": "xRyHrUEDs5tj"
+ },
+ "outputs": [],
+ "source": [
+ "import keras\n",
+ "from keras import ops\n",
+ "\n",
+ "import keras_cv\n",
+ "import numpy as np\n",
+ "\n",
+ "from keras_cv.datasets.pascal_voc.segmentation import load as load_voc"
+ ]
+ },
+ {
+ "cell_type": "markdown",
+ "metadata": {
+ "id": "98f7WhdZs5tj"
+ },
+ "source": [
+ "## Perform semantic segmentation with a pretrained DeepLabv3+ model\n",
+ "\n",
+ "The highest level API in the KerasCV semantic segmentation API is the `keras_cv.models`\n",
+ "API. This API includes fully pretrained semantic segmentation models, such as\n",
+ "`keras_cv.models.DeepLabV3Plus`.\n",
+ "\n",
+ "Let's get started by constructing a DeepLabv3+ pretrained on the pascalvoc dataset."
+ ]
+ },
+ {
+ "cell_type": "code",
+ "execution_count": null,
+ "metadata": {
+ "id": "M97l1P2Ms5tj"
+ },
+ "outputs": [],
+ "source": [
+ "model = keras_cv.models.DeepLabV3Plus.from_preset(\n",
+ " \"deeplab_v3_plus_resnet50_pascalvoc\",\n",
+ " num_classes=21,\n",
+ " input_shape=[512, 512, 3],\n",
+ ")"
+ ]
+ },
+ {
+ "cell_type": "markdown",
+ "metadata": {
+ "id": "9lUDEOr4s5tk"
+ },
+ "source": [
+ "Let us visualize the results of this pretrained model"
+ ]
+ },
+ {
+ "cell_type": "code",
+ "execution_count": null,
+ "metadata": {
+ "id": "nUzsOeyqs5tk"
+ },
+ "outputs": [],
+ "source": [
+ "filepath = keras.utils.get_file(origin=\"https://i.imgur.com/gCNcJJI.jpg\")\n",
+ "image = keras.utils.load_img(filepath)\n",
+ "\n",
+ "resize = keras_cv.layers.Resizing(height=512, width=512)\n",
+ "image = resize(image)\n",
+ "image = keras.ops.expand_dims(np.array(image), axis=0)\n",
+ "preds = ops.expand_dims(ops.argmax(model(image), axis=-1), axis=-1)\n",
+ "keras_cv.visualization.plot_segmentation_mask_gallery(\n",
+ " image,\n",
+ " value_range=(0, 255),\n",
+ " num_classes=1,\n",
+ " y_true=None,\n",
+ " y_pred=preds,\n",
+ " scale=3,\n",
+ " rows=1,\n",
+ " cols=1,\n",
+ ")"
+ ]
+ },
+ {
+ "cell_type": "markdown",
+ "metadata": {
+ "id": "vyqoiZcis5tk"
+ },
+ "source": [
+ "## Train a custom semantic segmentation model\n",
+ "In this guide, we'll assemble a full training pipeline for a KerasCV DeepLabV3 semantic\n",
+ "segmentation model. This includes data loading, augmentation, training, metric\n",
+ "evaluation, and inference!"
+ ]
+ },
+ {
+ "cell_type": "markdown",
+ "metadata": {
+ "id": "bLz1WdoZs5tk"
+ },
+ "source": [
+ "## Download the data\n",
+ "\n",
+ "We download\n",
+ "[Pascal VOC dataset](https://www.eecs.berkeley.edu/Research/Projects/CS/vision/grouping/semantic_contours/benchmark.tgz)\n",
+ "with KerasCV datasets and split them into train dataset `train_ds` and `eval_ds`."
+ ]
+ },
+ {
+ "cell_type": "code",
+ "execution_count": null,
+ "metadata": {
+ "id": "nfB7NSHHs5tk"
+ },
+ "outputs": [],
+ "source": [
+ "train_ds = load_voc(split=\"sbd_train\")\n",
+ "eval_ds = load_voc(split=\"sbd_eval\")"
+ ]
+ },
+ {
+ "cell_type": "markdown",
+ "metadata": {
+ "id": "fFF-YE1fs5tl"
+ },
+ "source": [
+ "## Preprocess the data\n",
+ "\n",
+ "The `preprocess_tfds_inputs` utility function preprocesses the inputs to a dictionary of\n",
+ "`images` and `segmentation_masks`. The images and segmentation masks are resized to\n",
+ "512x512. The resulting dataset is then batched into groups of 4 image and segmentation\n",
+ "mask pairs.\n",
+ "\n",
+ "A batch of this preprocessed input training data can be visualized using the\n",
+ "`keras_cv.visualization.plot_segmentation_mask_gallery` function. This function takes a\n",
+ "batch of images and segmentation masks as input and displays them in a grid."
+ ]
+ },
+ {
+ "cell_type": "code",
+ "execution_count": null,
+ "metadata": {
+ "id": "mD0Y8iMLs5tl"
+ },
+ "outputs": [],
+ "source": [
+ "def preprocess_tfds_inputs(inputs):\n",
+ " def unpackage_tfds_inputs(tfds_inputs):\n",
+ " return {\n",
+ " \"images\": tfds_inputs[\"image\"],\n",
+ " \"segmentation_masks\": tfds_inputs[\"class_segmentation\"],\n",
+ " }\n",
+ "\n",
+ " outputs = inputs.map(unpackage_tfds_inputs)\n",
+ " outputs = outputs.map(keras_cv.layers.Resizing(height=512, width=512))\n",
+ " outputs = outputs.batch(4, drop_remainder=True)\n",
+ " return outputs\n",
+ "\n",
+ "\n",
+ "train_ds = preprocess_tfds_inputs(train_ds)\n",
+ "batch = train_ds.take(1).get_single_element()\n",
+ "keras_cv.visualization.plot_segmentation_mask_gallery(\n",
+ " batch[\"images\"],\n",
+ " value_range=(0, 255),\n",
+ " num_classes=21, # The number of classes for the oxford iiit pet dataset. The VOC dataset also includes 1 class for the background.\n",
+ " y_true=batch[\"segmentation_masks\"],\n",
+ " scale=3,\n",
+ " rows=2,\n",
+ " cols=2,\n",
+ ")"
+ ]
+ },
+ {
+ "cell_type": "markdown",
+ "metadata": {
+ "id": "7NIGx0zHs5tl"
+ },
+ "source": [
+ "The preprocessing is applied to the evaluation dataset `eval_ds`."
+ ]
+ },
+ {
+ "cell_type": "code",
+ "execution_count": null,
+ "metadata": {
+ "id": "t0264OIJs5tl"
+ },
+ "outputs": [],
+ "source": [
+ "eval_ds = preprocess_tfds_inputs(eval_ds)"
+ ]
+ },
+ {
+ "cell_type": "markdown",
+ "metadata": {
+ "id": "KfPbd-TTs5tl"
+ },
+ "source": [
+ "## Data Augmentation\n",
+ "\n",
+ "KerasCV provides a variety of image augmentation options. In this example, we will use\n",
+ "the `RandomFlip` augmentation to augment the training dataset. The `RandomFlip`\n",
+ "augmentation randomly flips the images in the training dataset horizontally or\n",
+ "vertically. This can help to improve the model's robustness to changes in the orientation\n",
+ "of the objects in the images."
+ ]
+ },
+ {
+ "cell_type": "code",
+ "execution_count": null,
+ "metadata": {
+ "id": "W_0Ei44ls5tl"
+ },
+ "outputs": [],
+ "source": [
+ "train_ds = train_ds.map(keras_cv.layers.RandomFlip())\n",
+ "batch = train_ds.take(1).get_single_element()\n",
+ "\n",
+ "keras_cv.visualization.plot_segmentation_mask_gallery(\n",
+ " batch[\"images\"],\n",
+ " value_range=(0, 255),\n",
+ " num_classes=21,\n",
+ " y_true=batch[\"segmentation_masks\"],\n",
+ " scale=3,\n",
+ " rows=2,\n",
+ " cols=2,\n",
+ ")"
+ ]
+ },
+ {
+ "cell_type": "markdown",
+ "metadata": {
+ "id": "M99ecGY4s5tm"
+ },
+ "source": [
+ "## Model Configuration\n",
+ "\n",
+ "Please feel free to modify the configurations for model training and note how the\n",
+ "training results changes. This is an great exercise to get a better understanding of the\n",
+ "training pipeline.\n",
+ "\n",
+ "The learning rate schedule is used by the optimizer to calculate the learning rate for\n",
+ "each epoch. The optimizer then uses the learning rate to update the weights of the model.\n",
+ "In this case, the learning rate schedule uses a cosine decay function. A cosine decay\n",
+ "function starts high and then decreases over time, eventually reaching zero. The\n",
+ "cardinality of the VOC dataset is 2124 with a batch size of 4. The dataset cardinality\n",
+ "is important for learning rate decay because it determines how many steps the model\n",
+ "will train for. The initial learning rate is proportional to 0.007 and the decay\n",
+ "steps are 2124. This means that the learning rate will start at `INITIAL_LR` and then\n",
+ "decrease to zero over 2124 steps.\n",
+ ""
+ ]
+ },
+ {
+ "cell_type": "code",
+ "execution_count": null,
+ "metadata": {
+ "id": "4zqr0oF5s5tm"
+ },
+ "outputs": [],
+ "source": [
+ "BATCH_SIZE = 4\n",
+ "INITIAL_LR = 0.007 * BATCH_SIZE / 16\n",
+ "EPOCHS = 1\n",
+ "NUM_CLASSES = 21\n",
+ "learning_rate = keras.optimizers.schedules.CosineDecay(\n",
+ " INITIAL_LR,\n",
+ " decay_steps=EPOCHS * 2124,\n",
+ ")"
+ ]
+ },
+ {
+ "cell_type": "markdown",
+ "metadata": {
+ "id": "ES4SUSims5tm"
+ },
+ "source": [
+ "We instantiate a DeepLabV3+ model with a ResNet50 backbone pretrained on ImageNet classification:\n",
+ "`resnet50_v2_imagenet` pre-trained weights will be used as the backbone feature\n",
+ "extractor for the DeepLabV3Plus model. The `num_classes` parameter specifies the number of\n",
+ "classes that the model will be trained to segment."
+ ]
+ },
+ {
+ "cell_type": "code",
+ "execution_count": null,
+ "metadata": {
+ "id": "LoNY90Cgs5tm"
+ },
+ "outputs": [],
+ "source": [
+ "model = keras_cv.models.DeepLabV3Plus.from_preset(\n",
+ " \"resnet50_v2_imagenet\", num_classes=NUM_CLASSES\n",
+ ")"
+ ]
+ },
+ {
+ "cell_type": "markdown",
+ "metadata": {
+ "id": "wlwA_LTUs5tm"
+ },
+ "source": [
+ "## Compile the model\n",
+ "\n",
+ "The model.compile() function sets up the training process for the model. It defines the\n",
+ "- optimization algorithm - Stochastic Gradient Descent (SGD)\n",
+ "- the loss function - categorical cross-entropy\n",
+ "- the evaluation metrics - Mean IoU and categorical accuracy\n",
+ "\n",
+ "Semantic segmentation evaluation metrics:\n",
+ "\n",
+ "Mean Intersection over Union (MeanIoU):\n",
+ "MeanIoU measures how well a semantic segmentation model accurately identifies\n",
+ "and delineates different objects or regions in an image. It calculates the\n",
+ "overlap between predicted and actual object boundaries, providing a score\n",
+ "between 0 and 1, where 1 represents a perfect match.\n",
+ "\n",
+ "Categorical Accuracy:\n",
+ "Categorical Accuracy measures the proportion of correctly classified pixels in\n",
+ "an image. It gives a simple percentage indicating how accurately the model\n",
+ "predicts the categories of pixels in the entire image.\n",
+ "\n",
+ "In essence, MeanIoU emphasizes the accuracy of identifying specific object\n",
+ "boundaries, while Categorical Accuracy gives a broad overview of overall\n",
+ "pixel-level correctness."
+ ]
+ },
+ {
+ "cell_type": "code",
+ "execution_count": null,
+ "metadata": {
+ "id": "uM-Im0Mjs5tn"
+ },
+ "outputs": [],
+ "source": [
+ "model.compile(\n",
+ " optimizer=keras.optimizers.SGD(\n",
+ " learning_rate=learning_rate,\n",
+ " weight_decay=0.0001,\n",
+ " momentum=0.9,\n",
+ " clipnorm=10.0,\n",
+ " ),\n",
+ " loss=keras.losses.CategoricalCrossentropy(from_logits=False),\n",
+ " metrics=[\n",
+ " keras.metrics.MeanIoU(\n",
+ " num_classes=NUM_CLASSES, sparse_y_true=False, sparse_y_pred=False\n",
+ " ),\n",
+ " keras.metrics.CategoricalAccuracy(),\n",
+ " ],\n",
+ ")\n",
+ "\n",
+ "model.summary()"
+ ]
+ },
+ {
+ "cell_type": "markdown",
+ "metadata": {
+ "id": "Buh6A_1fs5tn"
+ },
+ "source": [
+ "The utility function `dict_to_tuple` effectively transforms the dictionaries of training\n",
+ "and validation datasets into tuples of images and one-hot encoded segmentation masks,\n",
+ "which is used during training and evaluation of the DeepLabv3+ model."
+ ]
+ },
+ {
+ "cell_type": "code",
+ "execution_count": null,
+ "metadata": {
+ "id": "kOLcpKLbs5tn"
+ },
+ "outputs": [],
+ "source": [
+ "def dict_to_tuple(x):\n",
+ " import tensorflow as tf\n",
+ "\n",
+ " return x[\"images\"], tf.one_hot(\n",
+ " tf.cast(tf.squeeze(x[\"segmentation_masks\"], axis=-1), \"int32\"), 21\n",
+ " )\n",
+ "\n",
+ "\n",
+ "train_ds = train_ds.map(dict_to_tuple)\n",
+ "eval_ds = eval_ds.map(dict_to_tuple)\n",
+ "\n",
+ "model.fit(train_ds, validation_data=eval_ds, epochs=EPOCHS)"
+ ]
+ },
+ {
+ "cell_type": "markdown",
+ "metadata": {
+ "id": "r8ZSZmtPs5tn"
+ },
+ "source": [
+ "## Predictions with trained model\n",
+ "Now that the model training of DeepLabv3+ has completed, let's test it by making\n",
+ "predications\n",
+ "on a few sample images."
+ ]
+ },
+ {
+ "cell_type": "code",
+ "execution_count": null,
+ "metadata": {
+ "id": "RG07dyEUs5tn"
+ },
+ "outputs": [],
+ "source": [
+ "test_ds = load_voc(split=\"sbd_eval\")\n",
+ "test_ds = preprocess_tfds_inputs(test_ds)\n",
+ "\n",
+ "images, masks = next(iter(train_ds.take(1)))\n",
+ "images = ops.convert_to_tensor(images)\n",
+ "masks = ops.convert_to_tensor(masks)\n",
+ "preds = ops.expand_dims(ops.argmax(model(images), axis=-1), axis=-1)\n",
+ "masks = ops.expand_dims(ops.argmax(masks, axis=-1), axis=-1)\n",
+ "\n",
+ "keras_cv.visualization.plot_segmentation_mask_gallery(\n",
+ " images,\n",
+ " value_range=(0, 255),\n",
+ " num_classes=21,\n",
+ " y_true=masks,\n",
+ " y_pred=preds,\n",
+ " scale=3,\n",
+ " rows=1,\n",
+ " cols=4,\n",
+ ")"
+ ]
+ },
+ {
+ "cell_type": "markdown",
+ "metadata": {
+ "id": "loWDjb1_s5tn"
+ },
+ "source": [
+ "Here are some additional tips for using the KerasCV DeepLabv3+ model:\n",
+ "\n",
+ "- The model can be trained on a variety of datasets, including the COCO dataset, the\n",
+ "PASCAL VOC dataset, and the Cityscapes dataset.\n",
+ "- The model can be fine-tuned on a custom dataset to improve its performance on a\n",
+ "specific task.\n",
+ "- The model can be used to perform real-time inference on images.\n",
+ "- Also, try out KerasCV's SegFormer model `keras_cv.models.segmentation.SegFormer`. The\n",
+ "SegFormer model is a newer model that has been shown to achieve state-of-the-art results\n",
+ "on a variety of image segmentation benchmarks. It is based on the Swin Transformer\n",
+ "architecture, and it is more efficient and accurate than previous image segmentation\n",
+ "models."
+ ]
+ }
+ ],
+ "metadata": {
+ "accelerator": "GPU",
+ "colab": {
+ "name": "semantic_segmentation_deeplab_v3_plus",
+ "provenance": [],
+ "toc_visible": true
+ },
+ "kernelspec": {
+ "display_name": "Python 3",
+ "language": "python",
+ "name": "python3"
+ },
+ "language_info": {
+ "codemirror_mode": {
+ "name": "ipython",
+ "version": 3
+ },
+ "file_extension": ".py",
+ "mimetype": "text/x-python",
+ "name": "python",
+ "nbconvert_exporter": "python",
+ "pygments_lexer": "ipython3",
+ "version": "3.7.0"
+ }
+ },
+ "nbformat": 4,
+ "nbformat_minor": 0
+}
\ No newline at end of file
diff --git a/requirements-common.txt b/requirements-common.txt
index fc21cc5f96..29f7ee9a19 100644
--- a/requirements-common.txt
+++ b/requirements-common.txt
@@ -13,4 +13,4 @@ isort
black
pytest
build
-namex
\ No newline at end of file
+namex
diff --git a/requirements-jax-cuda.txt b/requirements-jax-cuda.txt
index 99157c6d66..b3bb025e42 100644
--- a/requirements-jax-cuda.txt
+++ b/requirements-jax-cuda.txt
@@ -9,6 +9,6 @@ torchvision>=0.16.0
# Jax with cuda support.
# TODO: 0.4.24 has an updated Cuda version breaks Jax CI.
--find-links https://storage.googleapis.com/jax-releases/jax_cuda_releases.html
-jax[cuda12_pip]==0.4.24
+jax[cuda12_pip]==0.4.23
-r requirements-common.txt
\ No newline at end of file
|